Synthetic location trajectory generation using categorical diffusion models
CoRR(2024)
摘要
Diffusion probabilistic models (DPMs) have rapidly evolved to be one of the
predominant generative models for the simulation of synthetic data, for
instance, for computer vision, audio, natural language processing, or
biomolecule generation. Here, we propose using DPMs for the generation of
synthetic individual location trajectories (ILTs) which are sequences of
variables representing physical locations visited by individuals. ILTs are of
major importance in mobility research to understand the mobility behavior of
populations and to ultimately inform political decision-making. We represent
ILTs as multi-dimensional categorical random variables and propose to model
their joint distribution using a continuous DPM by first applying the diffusion
process in a continuous unconstrained space and then mapping the continuous
variables into a discrete space. We demonstrate that our model can synthesize
realistic ILPs by comparing conditionally and unconditionally generated
sequences to real-world ILPs from a GNSS tracking data set which suggests the
potential use of our model for synthetic data generation, for example, for
benchmarking models used in mobility research.
更多查看译文
AI 理解论文
溯源树
样例
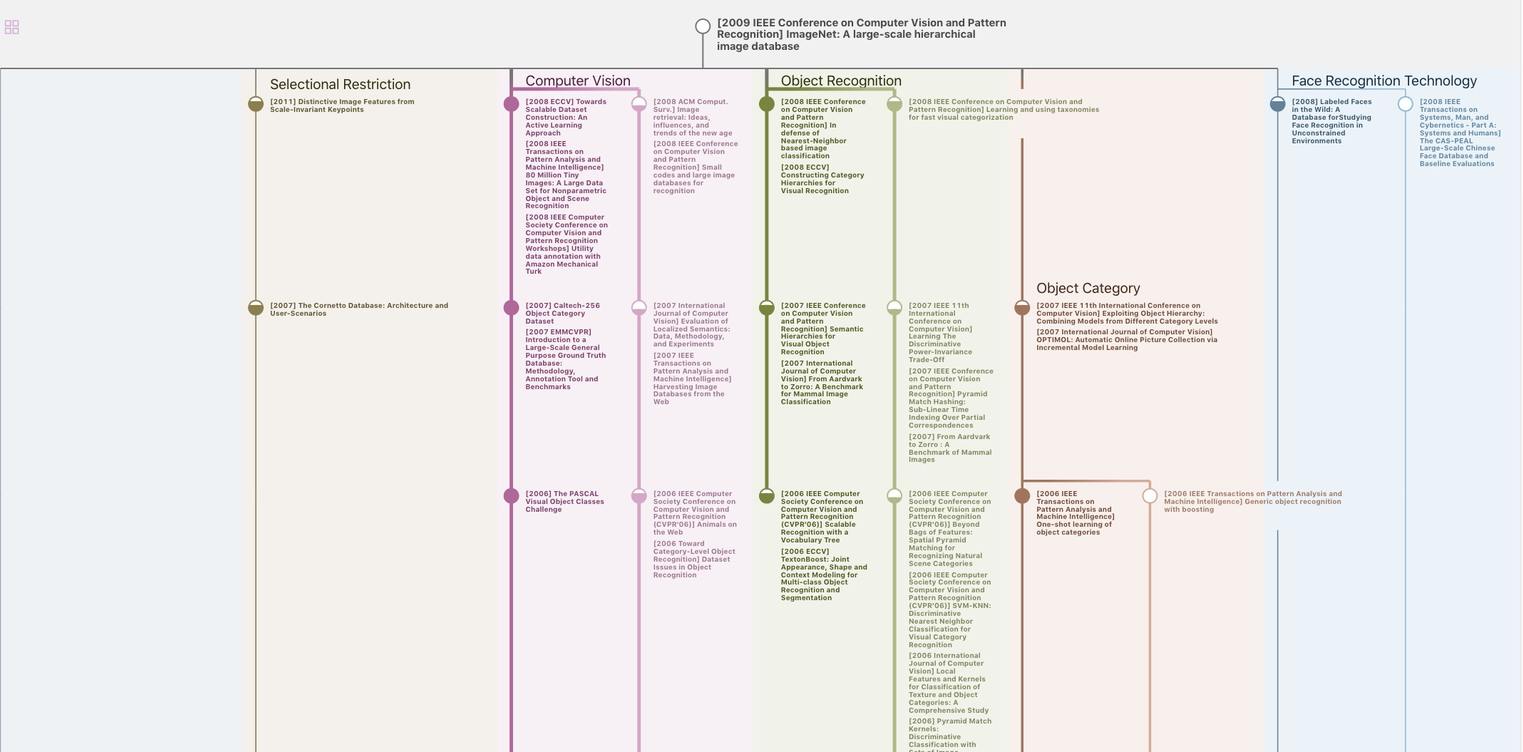
生成溯源树,研究论文发展脉络
Chat Paper
正在生成论文摘要