Convergence of Gradient Descent for Recurrent Neural Networks: A Nonasymptotic Analysis
CoRR(2024)
摘要
We analyze recurrent neural networks trained with gradient descent in the
supervised learning setting for dynamical systems, and prove that gradient
descent can achieve optimality without massive overparameterization. Our
in-depth nonasymptotic analysis (i) provides sharp bounds on the network size
m and iteration complexity τ in terms of the sequence length T, sample
size n and ambient dimension d, and (ii) identifies the significant impact
of long-term dependencies in the dynamical system on the convergence and
network width bounds characterized by a cutoff point that depends on the
Lipschitz continuity of the activation function. Remarkably, this analysis
reveals that an appropriately-initialized recurrent neural network trained with
n samples can achieve optimality with a network size m that scales only
logarithmically with n. This sharply contrasts with the prior works that
require high-order polynomial dependency of m on n to establish strong
regularity conditions. Our results are based on an explicit characterization of
the class of dynamical systems that can be approximated and learned by
recurrent neural networks via norm-constrained transportation mappings, and
establishing local smoothness properties of the hidden state with respect to
the learnable parameters.
更多查看译文
AI 理解论文
溯源树
样例
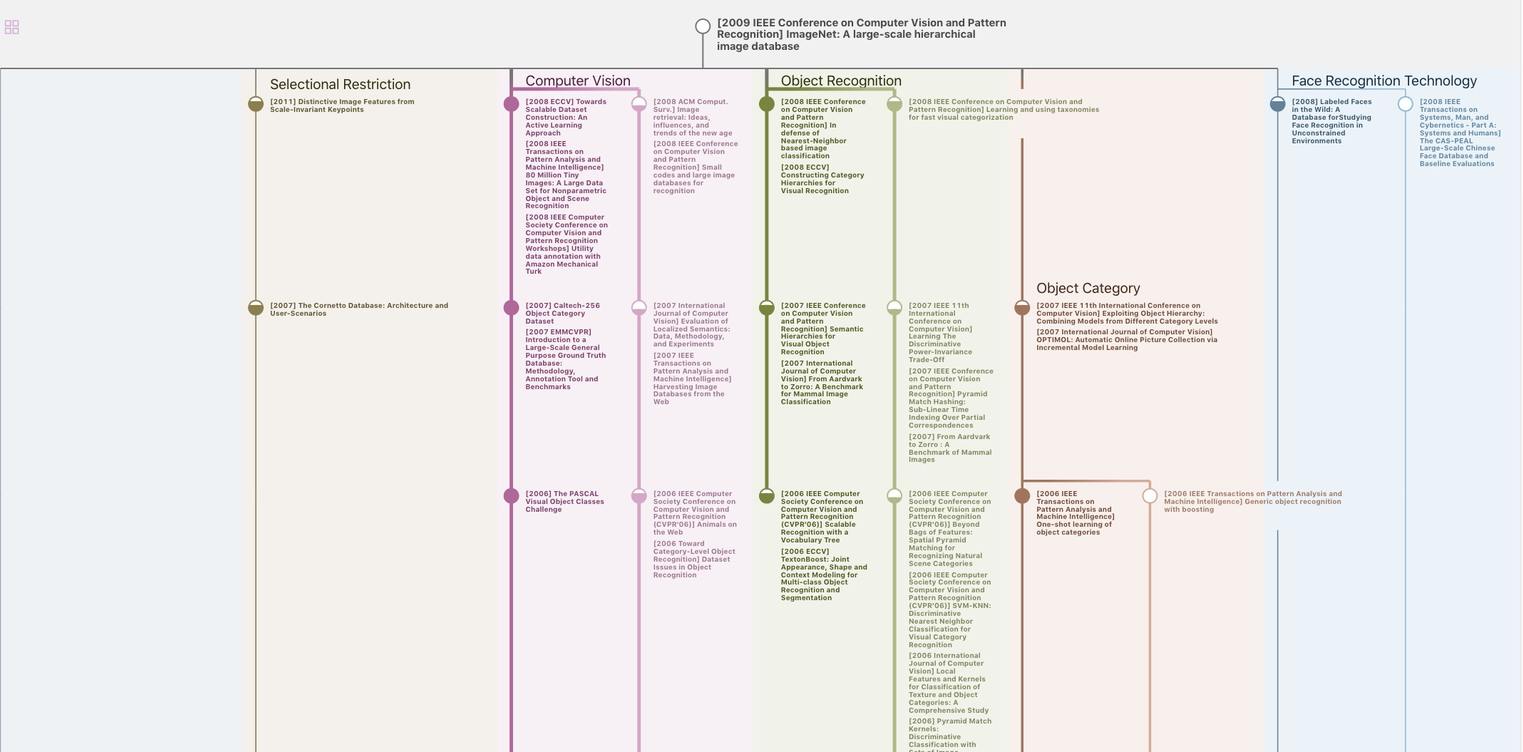
生成溯源树,研究论文发展脉络
Chat Paper
正在生成论文摘要