Kernel KMeans clustering splits for end-to-end unsupervised decision trees
CoRR(2024)
Abstract
Trees are convenient models for obtaining explainable predictions on
relatively small datasets. Although there are many proposals for the end-to-end
construction of such trees in supervised learning, learning a tree end-to-end
for clustering without labels remains an open challenge. As most works focus on
interpreting with trees the result of another clustering algorithm, we present
here a novel end-to-end trained unsupervised binary tree for clustering: Kauri.
This method performs a greedy maximisation of the kernel KMeans objective
without requiring the definition of centroids. We compare this model on
multiple datasets with recent unsupervised trees and show that Kauri performs
identically when using a linear kernel. For other kernels, Kauri often
outperforms the concatenation of kernel KMeans and a CART decision tree.
MoreTranslated text
AI Read Science
Must-Reading Tree
Example
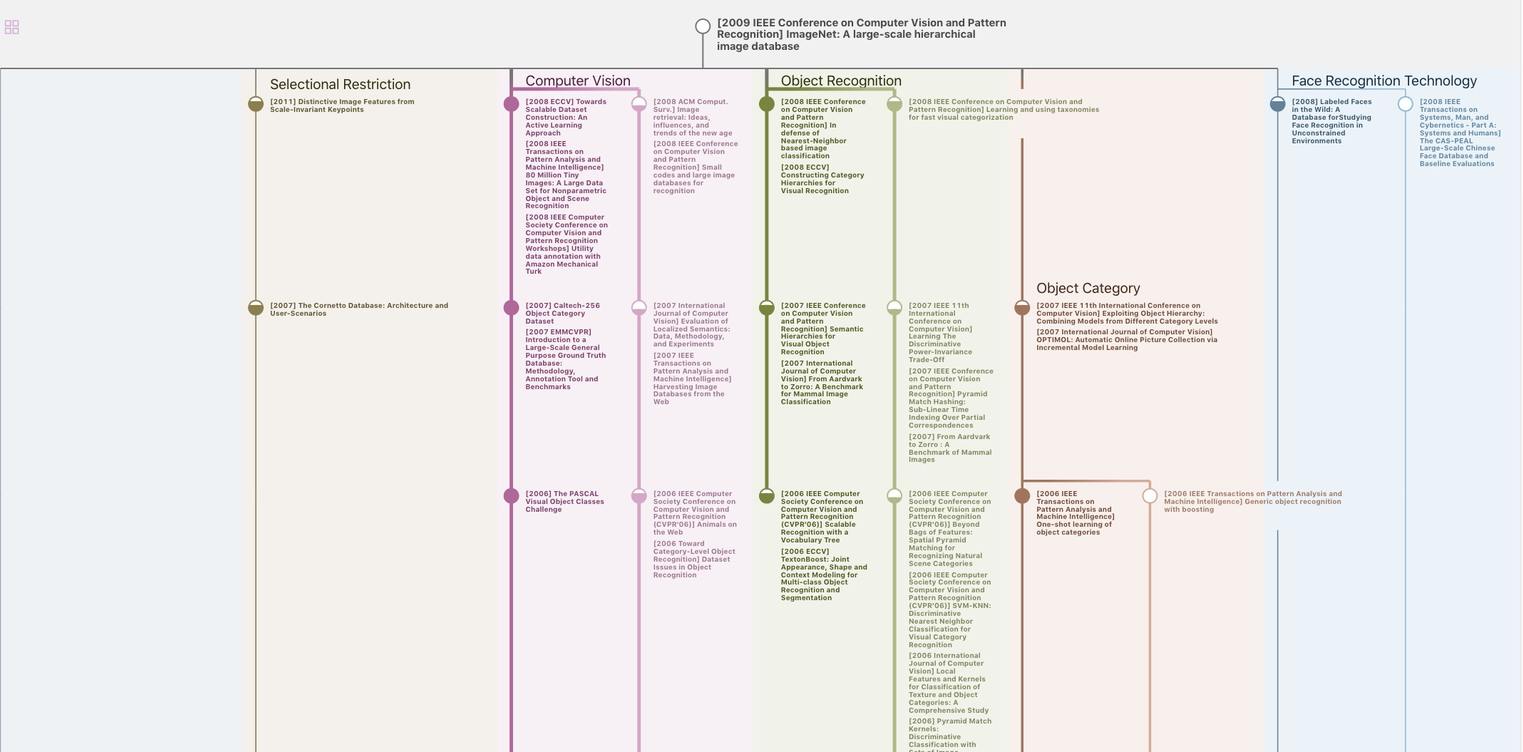
Generate MRT to find the research sequence of this paper
Chat Paper
Summary is being generated by the instructions you defined