Integrating Dynamic Weighted Approach with Fictitious Play and Pure Counterfactual Regret Minimization for Equilibrium Finding
CoRR(2024)
摘要
Developing efficient algorithms to converge to Nash Equilibrium is a key
focus in game theory. The use of dynamic weighting has been especially
advantageous in normal-form games, enhancing the rate of convergence. For
instance, the Greedy Regret Minimization (RM) algorithm has markedly
outperformed earlier techniques. Nonetheless, its dependency on mixed
strategies throughout the iterative process introduces complexity to dynamic
weighting, which in turn restricts its use in extensive-form games.
In this study, we introduce two novel dynamic weighting algorithms: Dynamic
Weighted Fictitious Play (DW-FP) and Dynamic Weighted Pure Counterfactual
Regret Minimization (DW-PCFR). These algorithms, utilizing pure strategies in
each iteration, offer key benefits: (i) Addressing the complexity of dynamic
weight computation in Greedy RM, thereby facilitating application in
extensive-form games; (ii) Incorporating the low-memory usage and ease-of-use
features of FP and CFR; (iii) They guarantee a convergence lower bound of
𝒪(T^-1/2), with a tendency to achieve a
convergence rate of 𝒪(T^-1) as runtime increases. This research
not only theoretically affirms the convergence capabilities of these algorithms
but also empirically demonstrates their superiority over existing leading
algorithms across all our tests.
更多查看译文
AI 理解论文
溯源树
样例
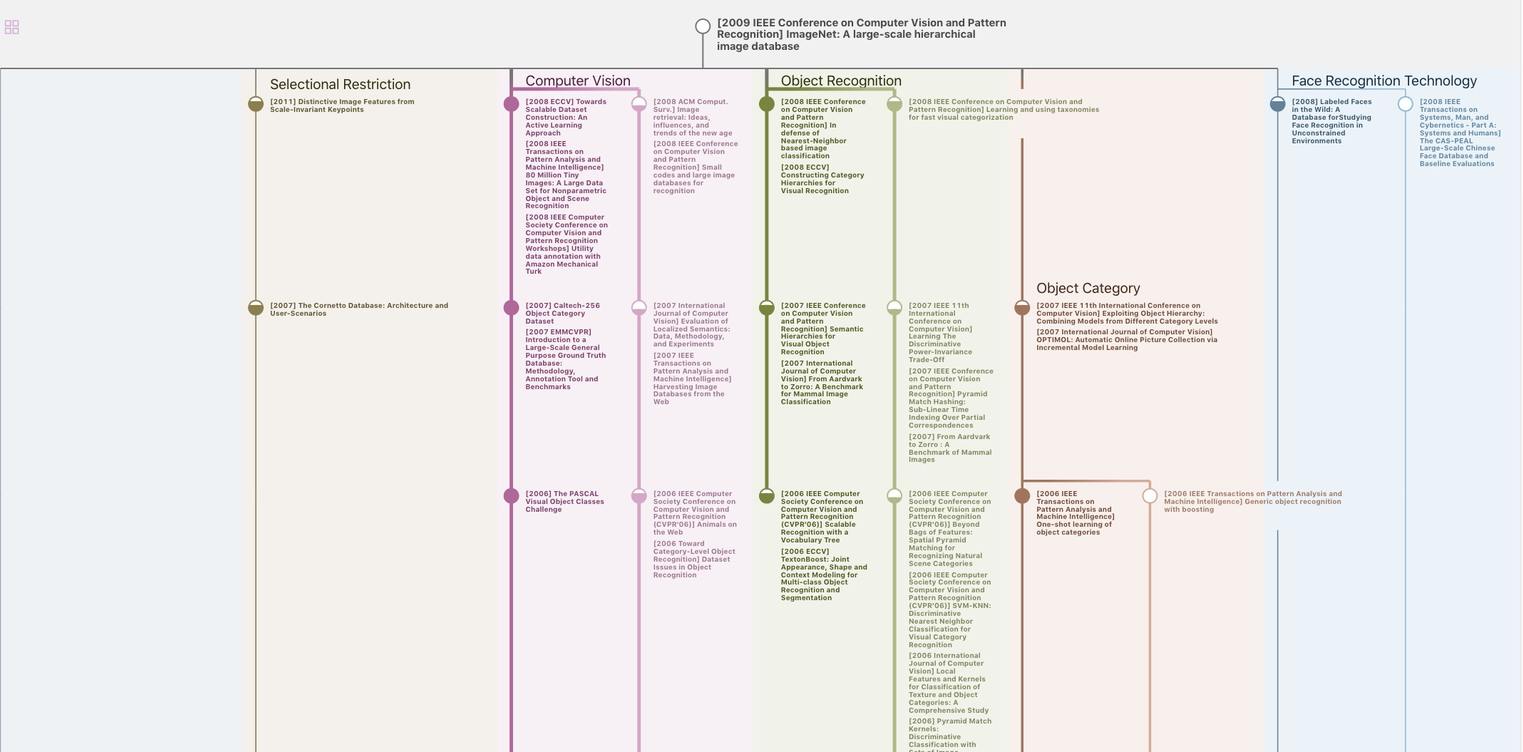
生成溯源树,研究论文发展脉络
Chat Paper
正在生成论文摘要