When Do Off-Policy and On-Policy Policy Gradient Methods Align?
CoRR(2024)
Abstract
Policy gradient methods are widely adopted reinforcement learning algorithms
for tasks with continuous action spaces. These methods succeeded in many
application domains, however, because of their notorious sample inefficiency
their use remains limited to problems where fast and accurate simulations are
available. A common way to improve sample efficiency is to modify their
objective function to be computable from off-policy samples without importance
sampling. A well-established off-policy objective is the excursion objective.
This work studies the difference between the excursion objective and the
traditional on-policy objective, which we refer to as the on-off gap. We
provide the first theoretical analysis showing conditions to reduce the on-off
gap while establishing empirical evidence of shortfalls arising when these
conditions are not met.
MoreTranslated text
AI Read Science
Must-Reading Tree
Example
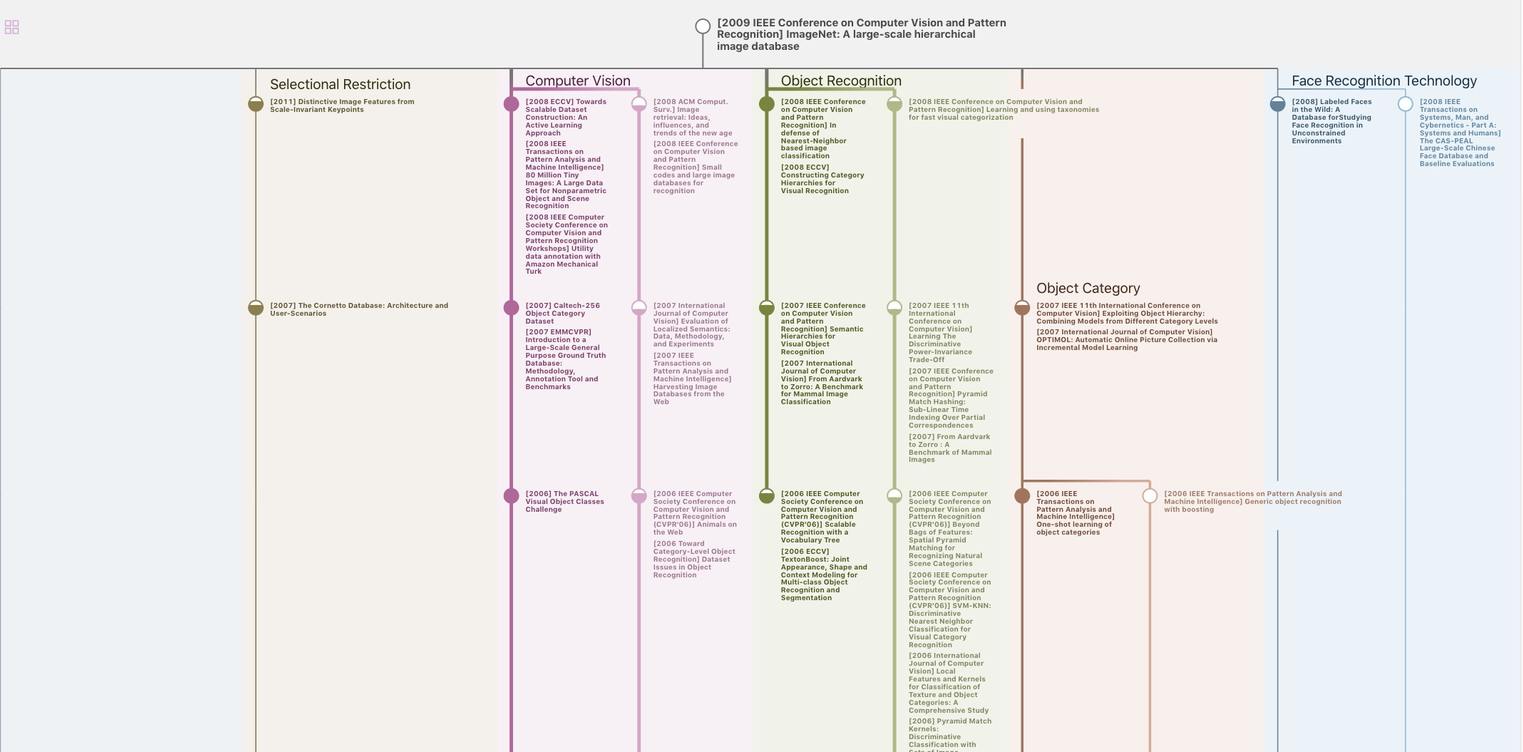
Generate MRT to find the research sequence of this paper
Chat Paper
Summary is being generated by the instructions you defined