Energy-Efficient Edge Learning Via Joint Data Deepening-and-Prefetching
ICC 2023 - IEEE INTERNATIONAL CONFERENCE ON COMMUNICATIONS(2023)
摘要
The vision of pervasive artificial intelligence (AI) services can be realizedby training an AI model on time using real-time data collected by internet ofthings (IoT) devices. To this end, IoT devices require offloading their data toan edge server in proximity. However, transmitting high-dimensional andvoluminous data from energy-constrained IoT devices poses a significantchallenge. To address this limitation, we propose a novel offloadingarchitecture, called joint data deepening-and-prefetching (JD2P), which isfeature-by-feature offloading comprising two key techniques. The first one isdata deepening, where each data sample's features are sequentially offloaded inthe order of importance determined by the data embedding technique such asprinciple component analysis (PCA). Offloading is terminated once the alreadytransmitted features are sufficient for accurate data classification, resultingin a reduction in the amount of transmitted data. The criteria to offload dataare derived for binary and multi-class classifiers, which are designed based onsupport vector machine (SVM) and deep neural network (DNN), respectively. Thesecond one is data prefetching, where some features potentially required in thefuture are offloaded in advance, thus achieving high efficiency via preciseprediction and parameter optimization. We evaluate the effectiveness of JD2Pthrough experiments using the MNIST dataset, and the results demonstrate itssignificant reduction in expected energy consumption compared to severalbenchmarks without degrading learning accuracy.
更多查看译文
关键词
Edge learning,principal component analysis,feature importance,energy efficiency,data deepening,data prefetching
AI 理解论文
溯源树
样例
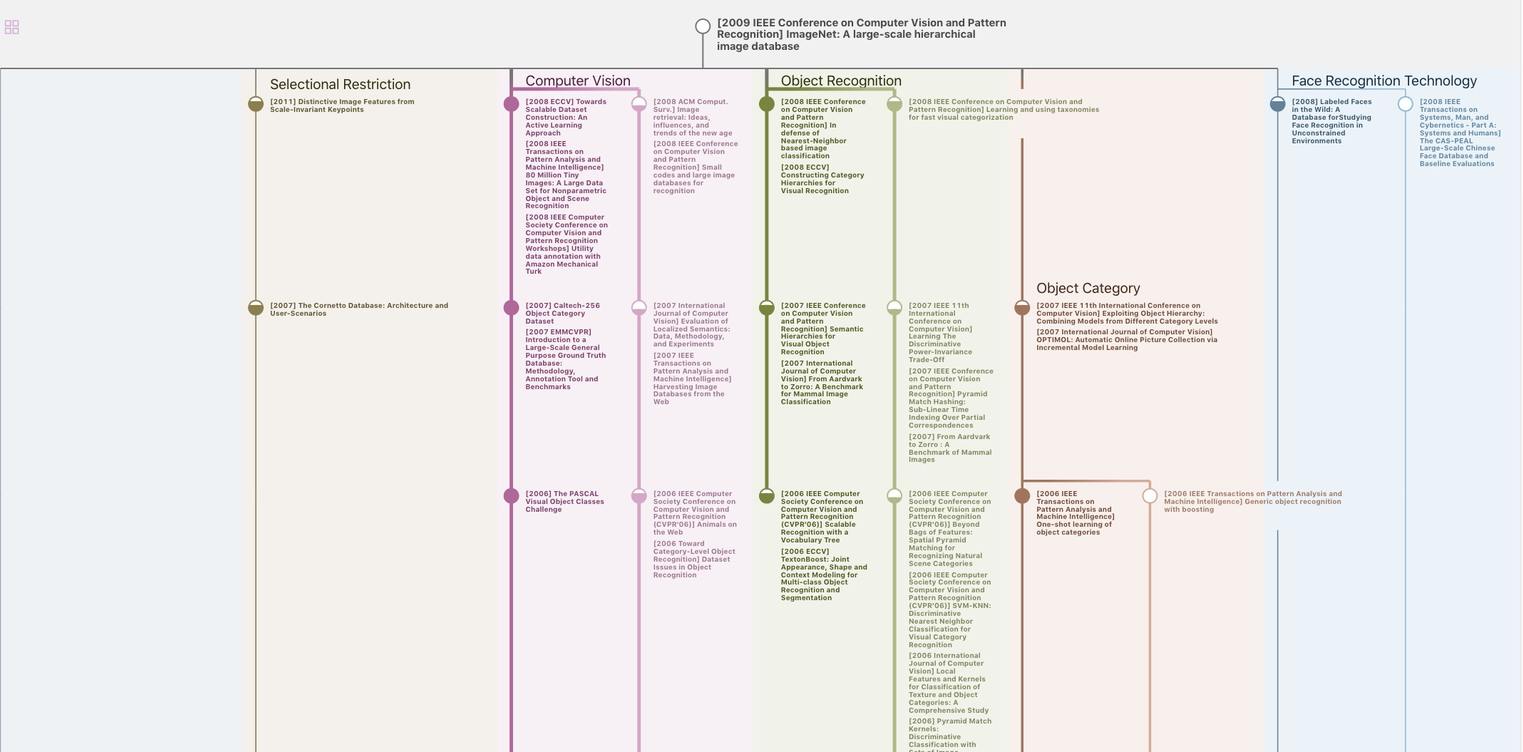
生成溯源树,研究论文发展脉络
Chat Paper
正在生成论文摘要