Adaptive Decision-Making for Autonomous Vehicles: A Learning-Enhanced Game-Theoretic Approach in Interactive Environments
CoRR(2024)
摘要
This paper proposes an adaptive behavioral decision-making method for
autonomous vehicles (AVs) focusing on complex merging scenarios. Leveraging
principles from non-cooperative game theory, we develop a vehicle interaction
behavior model that defines key traffic elements and integrates a
multifactorial reward function. Maximum entropy inverse reinforcement learning
(IRL) is employed for behavior model parameter optimization. Optimal matching
parameters can be obtained using the interaction behavior feature vector and
the behavior probabilities output by the vehicle interaction model. Further, a
behavioral decision-making method adapted to dynamic environments is proposed.
By establishing a mapping model between multiple environmental variables and
model parameters, it enables parameters online learning and recognition, and
achieves to output interactive behavior probabilities of AVs. Quantitative
analysis employing naturalistic driving datasets (highD and exiD) and
real-vehicle test data validates the model's high consistency with human
decision-making. In 188 tested interaction scenarios, the average human-like
similarity rate is 81.73
Furthermore, in 145 dynamic interactions, the method matches human decisions at
77.12
72.73
the effectiveness of our proposed method in enabling AVs to make informed
adaptive behavior decisions in interactive environments.
更多查看译文
AI 理解论文
溯源树
样例
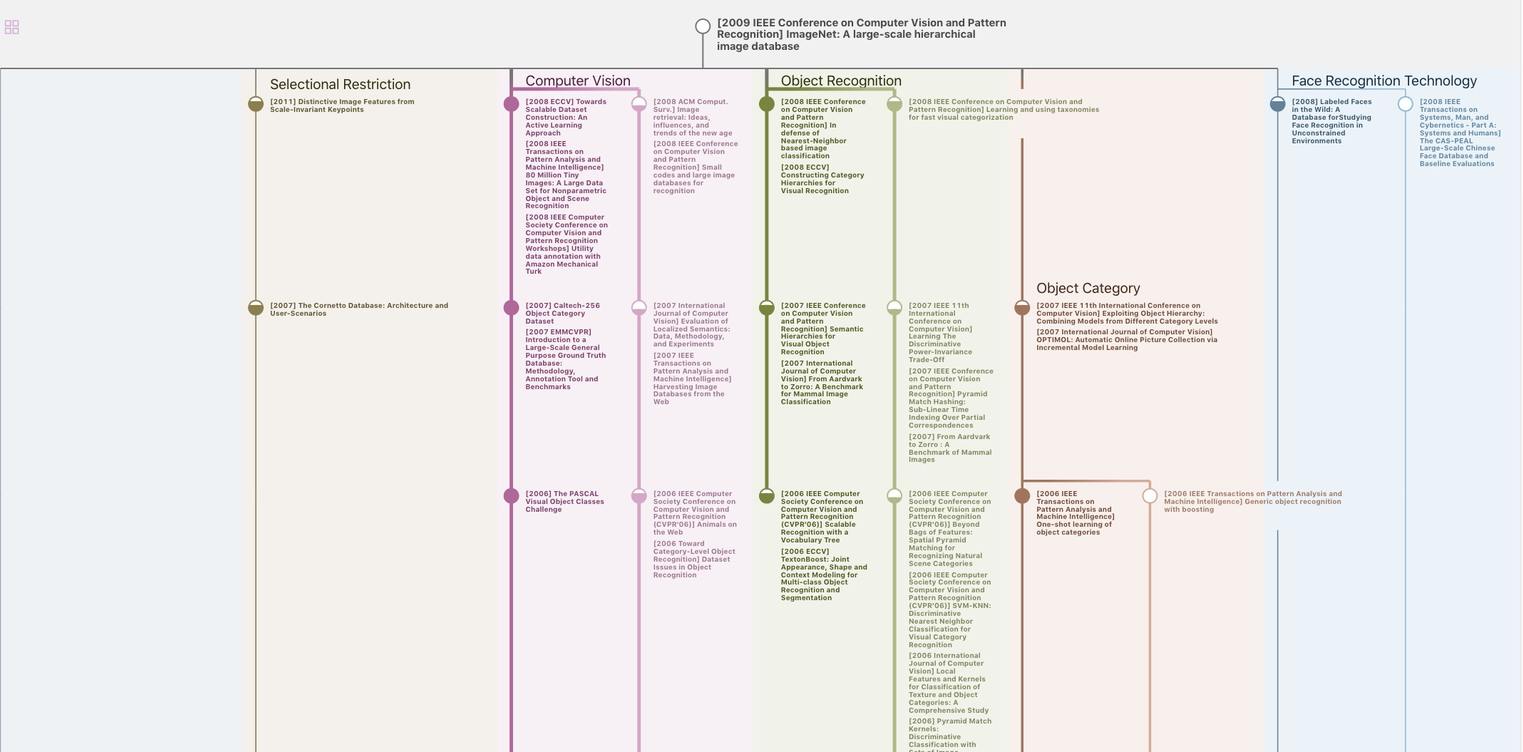
生成溯源树,研究论文发展脉络
Chat Paper
正在生成论文摘要