An Empirical Evaluation of Neural and Neuro-symbolic Approaches to Real-time Multimodal Complex Event Detection
CoRR(2024)
摘要
Robots and autonomous systems require an understanding of complex events
(CEs) from sensor data to interact with their environments and humans
effectively. Traditional end-to-end neural architectures, despite processing
sensor data efficiently, struggle with long-duration events due to limited
context sizes and reasoning capabilities. Recent advances in neuro-symbolic
methods, which integrate neural and symbolic models leveraging human knowledge,
promise improved performance with less data. This study addresses the gap in
understanding these approaches' effectiveness in complex event detection (CED),
especially in temporal reasoning. We investigate neural and neuro-symbolic
architectures' performance in a multimodal CED task, analyzing IMU and acoustic
data streams to recognize CE patterns. Our methodology includes (i) end-to-end
neural architectures for direct CE detection from sensor embeddings, (ii)
two-stage concept-based neural models mapping sensor embeddings to atomic
events (AEs) before CE detection, and (iii) a neuro-symbolic approach using a
symbolic finite-state machine for CE detection from AEs. Empirically, the
neuro-symbolic architecture significantly surpasses purely neural models,
demonstrating superior performance in CE recognition, even with extensive
training data and ample temporal context for neural approaches.
更多查看译文
AI 理解论文
溯源树
样例
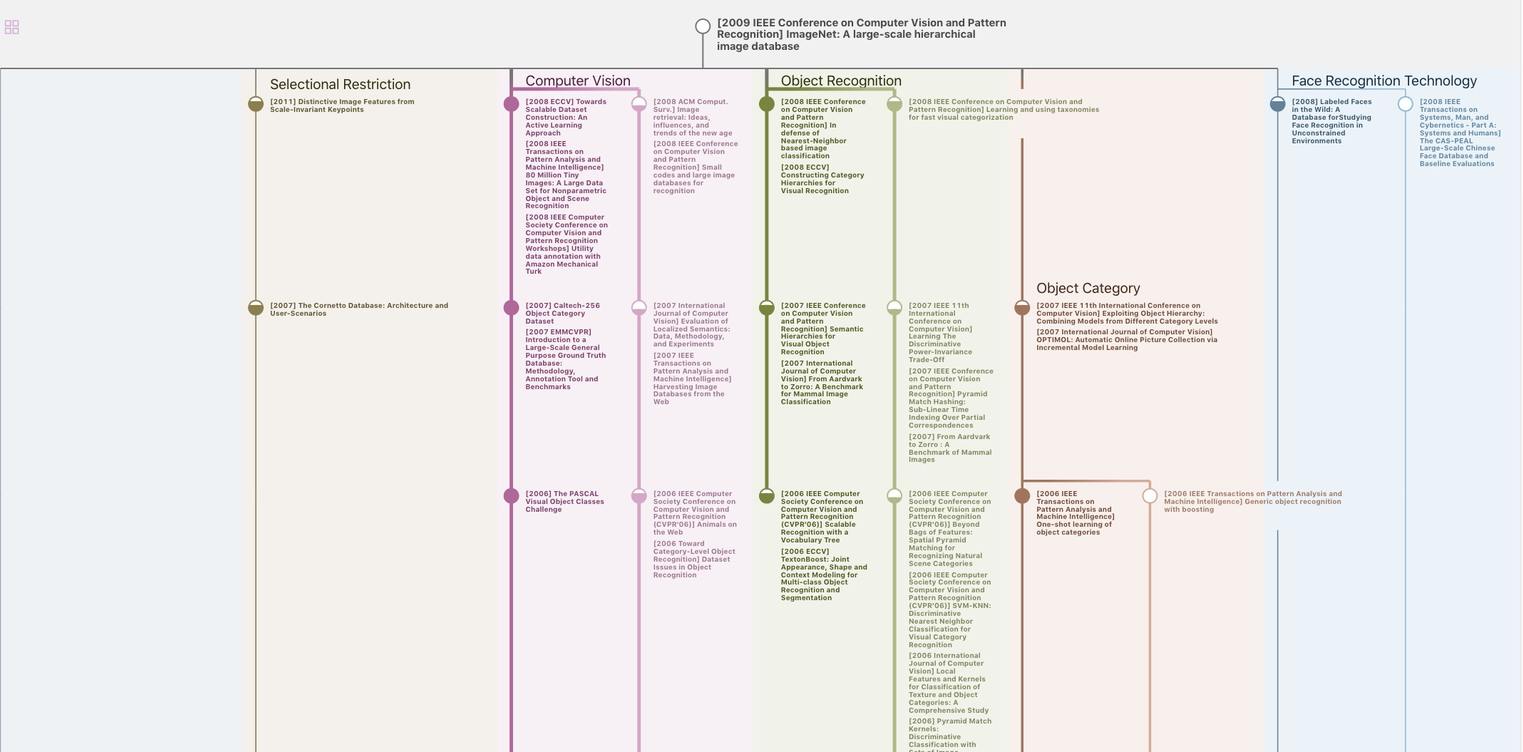
生成溯源树,研究论文发展脉络
Chat Paper
正在生成论文摘要