I Learn Better If You Speak My Language: Enhancing Large Language Model Fine-Tuning with Style-Aligned Response Adjustments
CoRR(2024)
摘要
Fine-tuning large language models (LLMs) with a small data set for particular
tasks is a widely encountered yet complex challenge. The potential for
overfitting on a limited number of examples can negatively impact the model's
ability to generalize and retain its original skills. Our research explores the
impact of the style of ground-truth responses during the fine-tuning process.
We found that matching the ground-truth response style with the LLM's inherent
style results in better learning outcomes. Building on this insight, we
developed a method that minimally alters the LLM's pre-existing responses to
correct errors, using these adjusted responses as training targets. This
technique enables precise corrections in line with the model's native response
style, safeguarding the model's core capabilities and thus avoid overfitting.
Our findings show that this approach not only improves the LLM's task-specific
accuracy but also crucially maintains its original competencies and
effectiveness.
更多查看译文
AI 理解论文
溯源树
样例
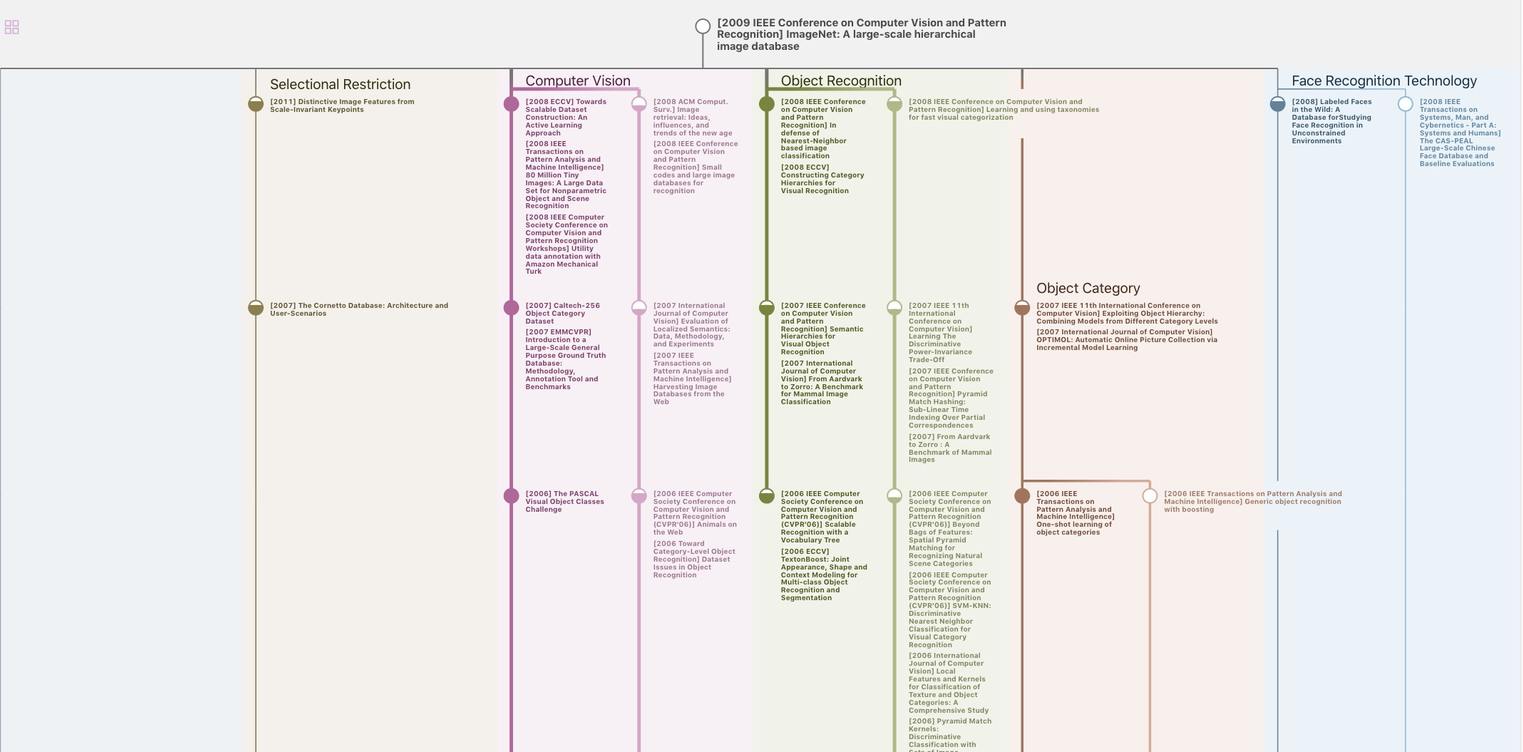
生成溯源树,研究论文发展脉络
Chat Paper
正在生成论文摘要