Integrating Pre-Trained Language Model with Physical Layer Communications
CoRR(2024)
Abstract
The burgeoning field of on-device AI communication, where devices exchange
information directly through embedded foundation models, such as language
models (LMs), requires robust, efficient, and generalizable communication
frameworks. However, integrating these frameworks with existing wireless
systems and effectively managing noise and bit errors pose significant
challenges. In this work, we introduce a practical on-device AI communication
framework, integrated with physical layer (PHY) communication functions,
demonstrated through its performance on a link-level simulator. Our framework
incorporates end-to-end training with channel noise to enhance resilience,
incorporates vector quantized variational autoencoders (VQ-VAE) for efficient
and robust communication, and utilizes pre-trained encoder-decoder transformers
for improved generalization capabilities. Simulations, across various
communication scenarios, reveal that our framework achieves a 50
transmission size while demonstrating substantial generalization ability and
noise robustness under standardized 3GPP channel models.
MoreTranslated text
AI Read Science
Must-Reading Tree
Example
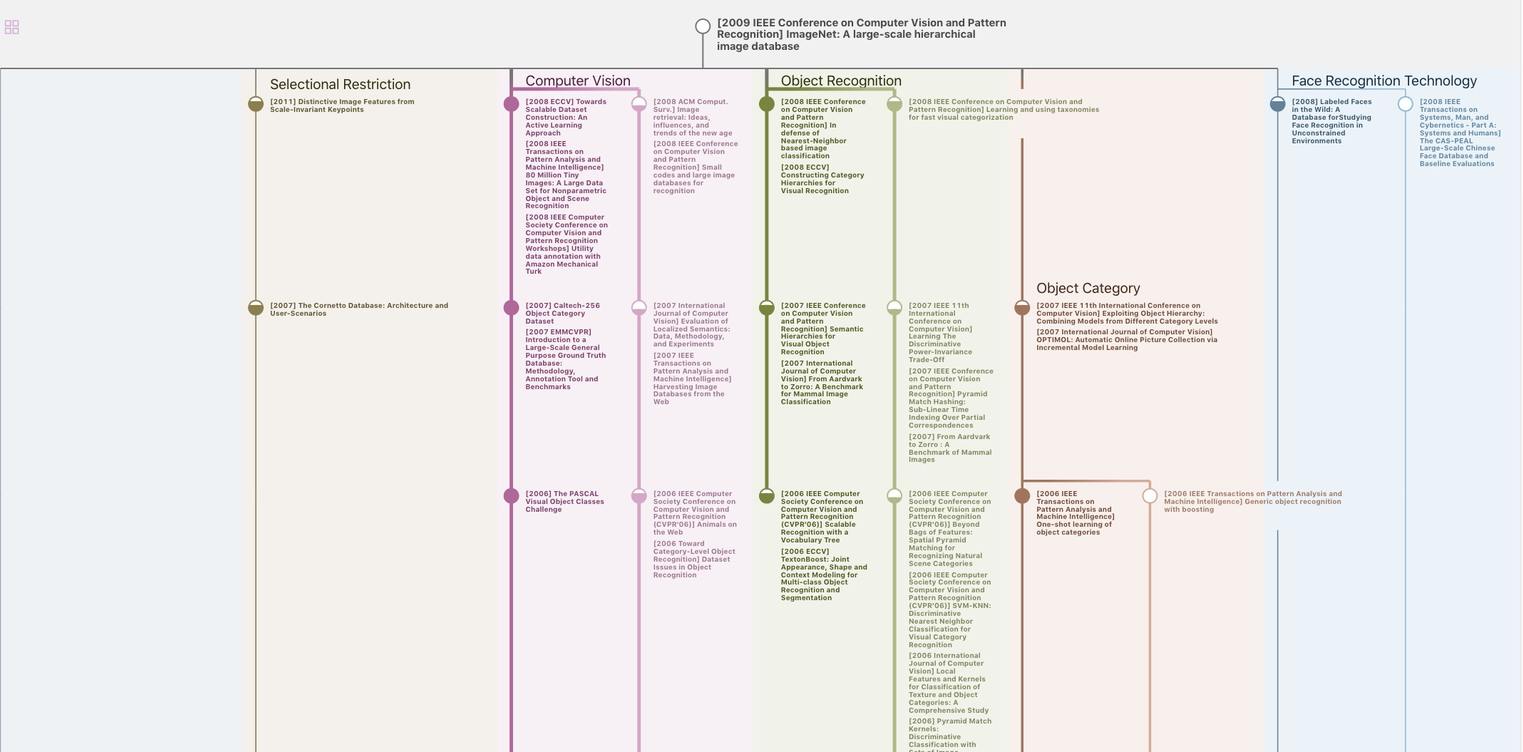
Generate MRT to find the research sequence of this paper
Chat Paper
Summary is being generated by the instructions you defined