Correcting Selection Bias in Big Data by Pseudo-Weighting (vol 11, pg 1181, 2022)
JOURNAL OF SURVEY STATISTICS AND METHODOLOGY(2023)
Abstract
Abstract Nonprobability samples, for example observational studies, online opt-in surveys, or register data, do not come from a sampling design and therefore may suffer from selection bias. To correct for selection bias, Elliott and Valliant (EV) proposed a pseudo-weight estimation method that applies a two-sample setup for a probability sample and a nonprobability sample drawn from the same population, sharing some common auxiliary variables. By estimating the propensities of inclusion in the nonprobability sample given the two samples, we may correct the selection bias by (pseudo) design-based approaches. This paper expands the original method, allowing for large sampling fractions in either sample or for high expected overlap between selected units in each sample, conditions often present in administrative data sets and more frequently occurring with Big Data.
MoreTranslated text
Key words
Big Data,Nonprobability sample,Propensity score,Pseudo population bootstrap,Selection bias
AI Read Science
Must-Reading Tree
Example
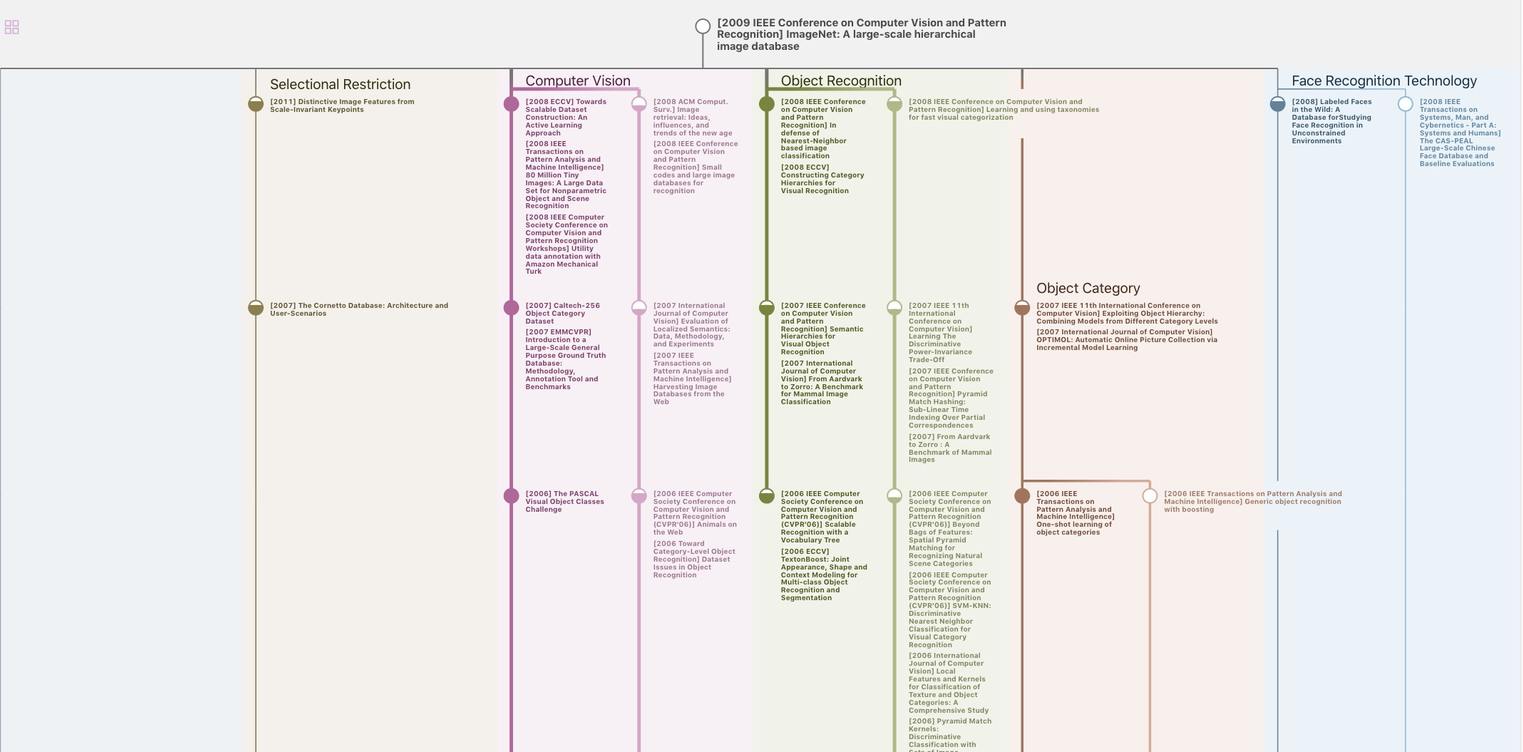
Generate MRT to find the research sequence of this paper
Chat Paper
Summary is being generated by the instructions you defined