CT radiomics analysis discriminates pulmonary lesions in patients with pulmonary MALT lymphoma and non-pulmonary MALT lymphoma
METHODS(2024)
摘要
Purpose: The aim of this study is to create and validate a radiomics model based on CT scans, enabling the distinction between pulmonary mucosa-associated lymphoid tissue (MALT) lymphoma and other pulmonary lesion causes. Methods: Patients diagnosed with primary pulmonary MALT lymphoma and lung infections at Fuzhou Pulmonary Hospital were randomly assigned to either a training group or a validation group. Meanwhile, individuals diagnosed with primary pulmonary MALT lymphoma and lung infections at Fujian Provincial Cancer Hospital were chosen as the external test group. We employed ITK-SNAP software for delineating the Region of Interest (ROI) within the images. Subsequently, we extracted radiomics features and convolutional neural networks using PyRadiomics, a component of the Onekey AI software suite. Relevant radiomic features were selected to build an intelligent diagnostic prediction model utilizing CT images, and the model's efficacy was assessed in both the validation group and the external test group. Results: Leveraging radiomics, ten distinct features were carefully chosen for analysis. Subsequently, this study employed the machine learning techniques of Logistic Regression (LR), Support Vector Machine (SVM), and kNearest Neighbors (KNN) to construct models using these ten selected radiomics features within the training groups. Among these, SVM exhibited the highest performance, achieving an accuracy of 0.868, 0.870, and 0.90 on the training, validation, and external testing groups, respectively. For LR, the accuracy was 0.837, 0.863, and 0.90 on the training, validation, and external testing groups, respectively. For KNN, the accuracy was 0.884, 0.859, and 0.790 on the training, validation, and external testing groups, respectively. Conclusion: We established a noninvasive radiomics model utilizing CT imaging to diagnose pulmonary MALT lymphoma associated with pulmonary lesions. This model presents a promising adjunct tool to enhance diagnostic specificity for pulmonary MALT lymphoma, particularly in populations where pulmonary lesion changes may be attributed to other causes.
更多查看译文
关键词
Pulmonary MALT lymphoma,Machine learning,Radiomics,Diagnosis
AI 理解论文
溯源树
样例
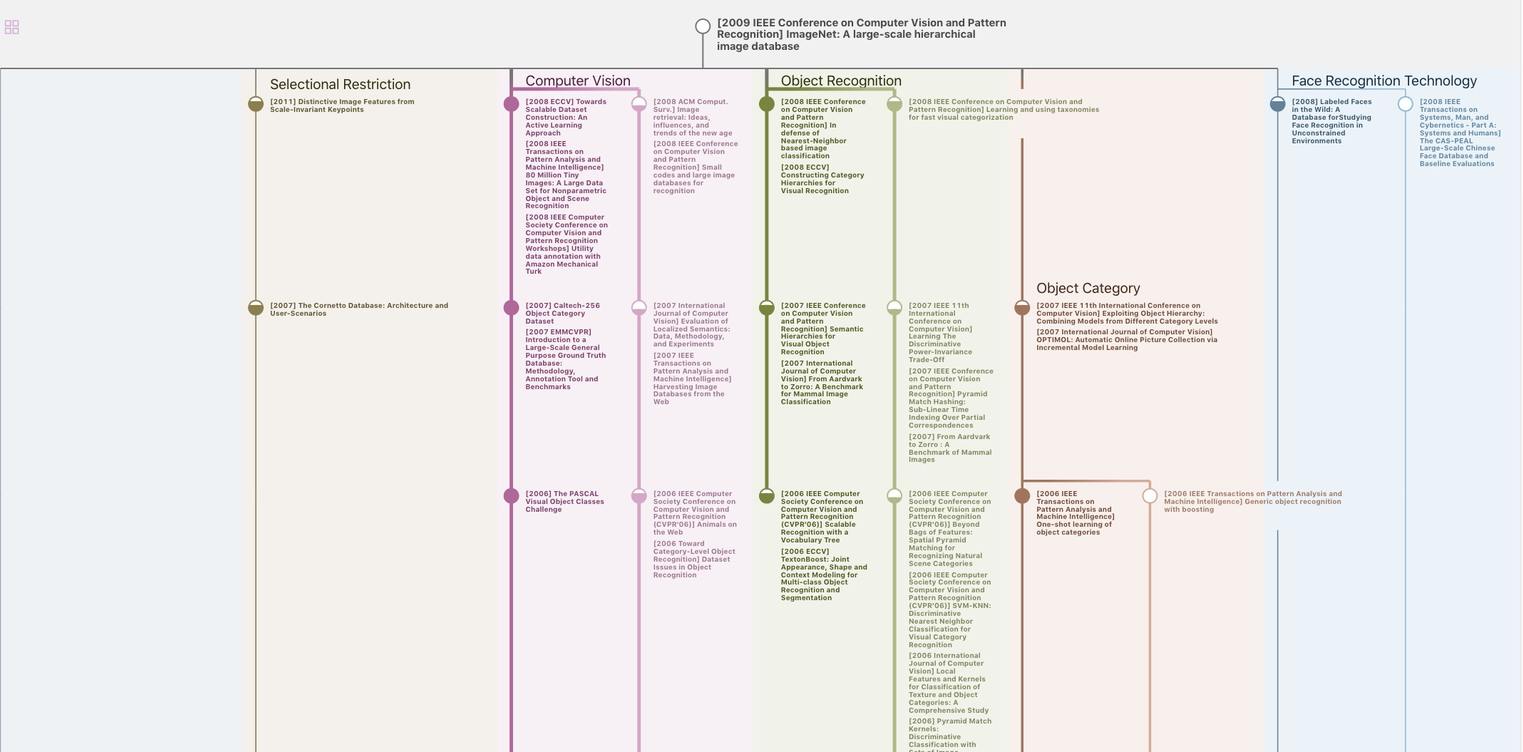
生成溯源树,研究论文发展脉络
Chat Paper
正在生成论文摘要