Integrating single-cell multimodal epigenomic data using 1D-convolutional neural networks
bioRxiv the preprint server for biology(2024)
Abstract
Recent experimental developments enable single-cell multimodal epigenomic profiling, which measures multiple histone modifications and chromatin accessibility within the same cell. Such parallel measurements provide exciting new opportunities to investigate how epigenomic modalities vary to-gether across cell types and states. A pivotal step in using this type of data is integrating the epigenomic modalities to learn a unified representation of each cell, but existing approaches are not designed to model the unique nature of this data type. Our key insight is to model single-cell multimodal epigenome data as a multi-channel sequential signal. Based on this insight, we developed ConvNet-VAEs, a novel framework that uses 1D-convolutional variational autoencoders (VAEs) for single-cell multimodal epigenomic data integration. We evaluated ConvNet-VAEs on nano-CT and scNTT-seq data generated from juvenile mouse brain and human bone marrow. We found that ConvNet-VAEs can perform dimension reduction and batch correction better than previous architectures while using significantly fewer parameters. Furthermore, the performance gap between convolutional and fully-connected architectures increases with the number of modalities, and deeper convolutional architectures can increase performance while performance degrades for deeper fully-connected architectures. Our results indicate that convolutional autoencoders are a promising method for integrating current and future single-cell multimodal epigenomic datasets.
### Competing Interest Statement
The authors have declared no competing interest.
MoreTranslated text
AI Read Science
Must-Reading Tree
Example
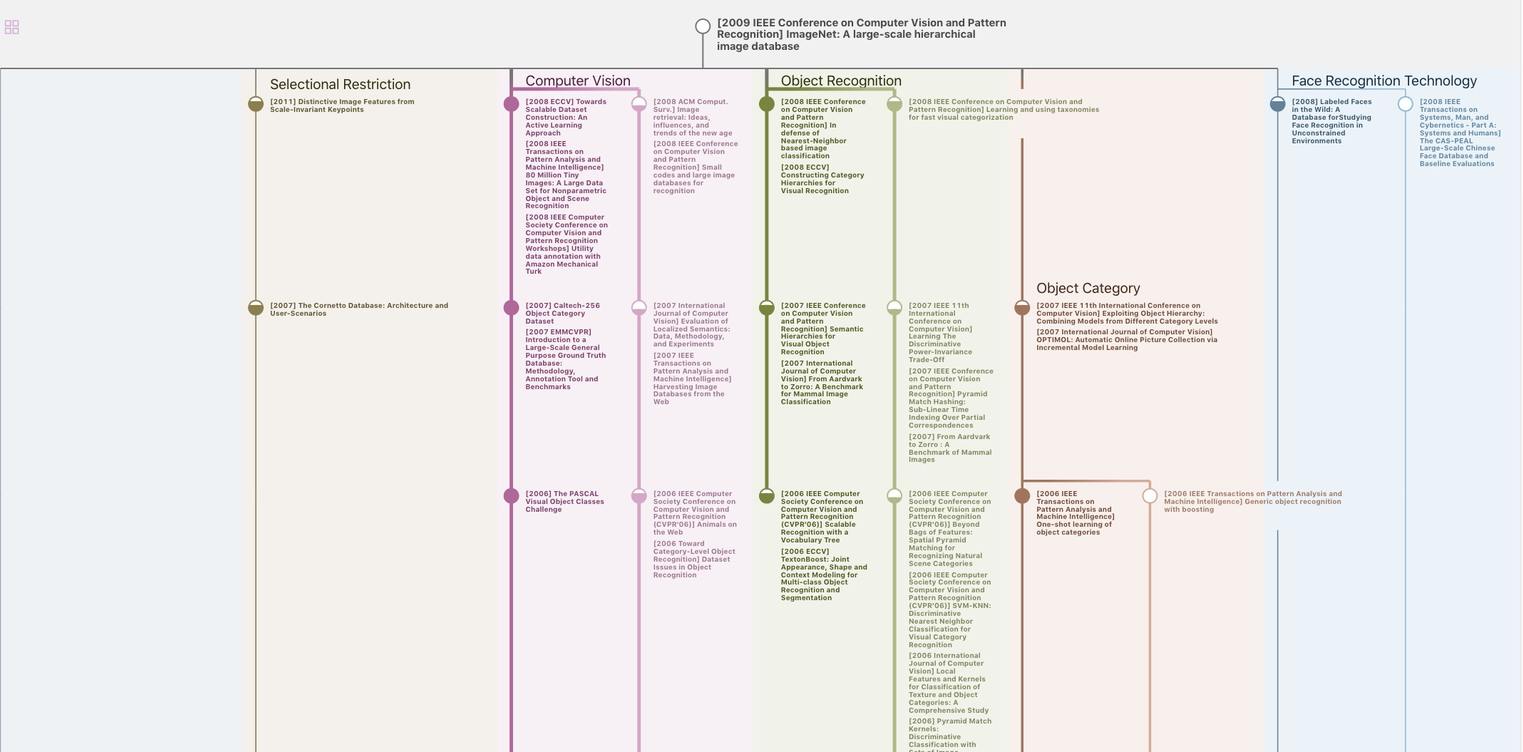
Generate MRT to find the research sequence of this paper
Chat Paper
Summary is being generated by the instructions you defined