Accuracy Analysis of Type-2 Fuzzy System in Predicting Parkinson’s Disease Using Biomedical Voice Measures
International Journal of Fuzzy Systems(2024)
摘要
Parkinson’s disease (PD) is a progressive neurodegenerative illness triggered by decreased dopamine secretion. Fuzzy logic has gained substantial attention in PD diagnosis research. PD detection using fuzzy logic has presented more precise outcomes as compared with common machine learning approaches. In this research, a hybrid method combining supervised learning, unsupervised learning and feature selection techniques is developed. In a type-1 fuzzy system, the membership functions used for the fuzzification of the crisp inputs are mapped to single numbers. However, in a type-2 fuzzy system, these numbers are represented as intervals, adding an extra dimension to the definition of the membership function. The first step of the proposed method involves clustering the data using the Expectation–Maximization (EM) technique. The performance of EM clustering is performed using the Davies–Bouldin index. Subsequently, feature selection is performed using the backward stepwise regression. To predict the UPDRS, Type-2 Sugeno fuzzy inference system (T2SFIS) is implemented on the clusters generated from the previous steps. The Parkinson's telemonitoring dataset is used in this study for method evaluation. Using the EM algorithm, the PD dataset was clustered into 13 segments, and the most important features for accurate UPDRS prediction were chosen in each segment using backward stepwise regression. The hybrid method was evaluated using R-squared ( R 2 ) and RMSE. The evaluation results showed that the combination of EM, backward stepwise regression, and type-2 Sugeno FIS obtained the best accuracy in predicting Motor-UPDRS and Total-UPDRS.
更多查看译文
关键词
Parkinson’s disease,Type-2 Sugeno fuzzy inference system,Expectation–maximization,Backward stepwise regression
AI 理解论文
溯源树
样例
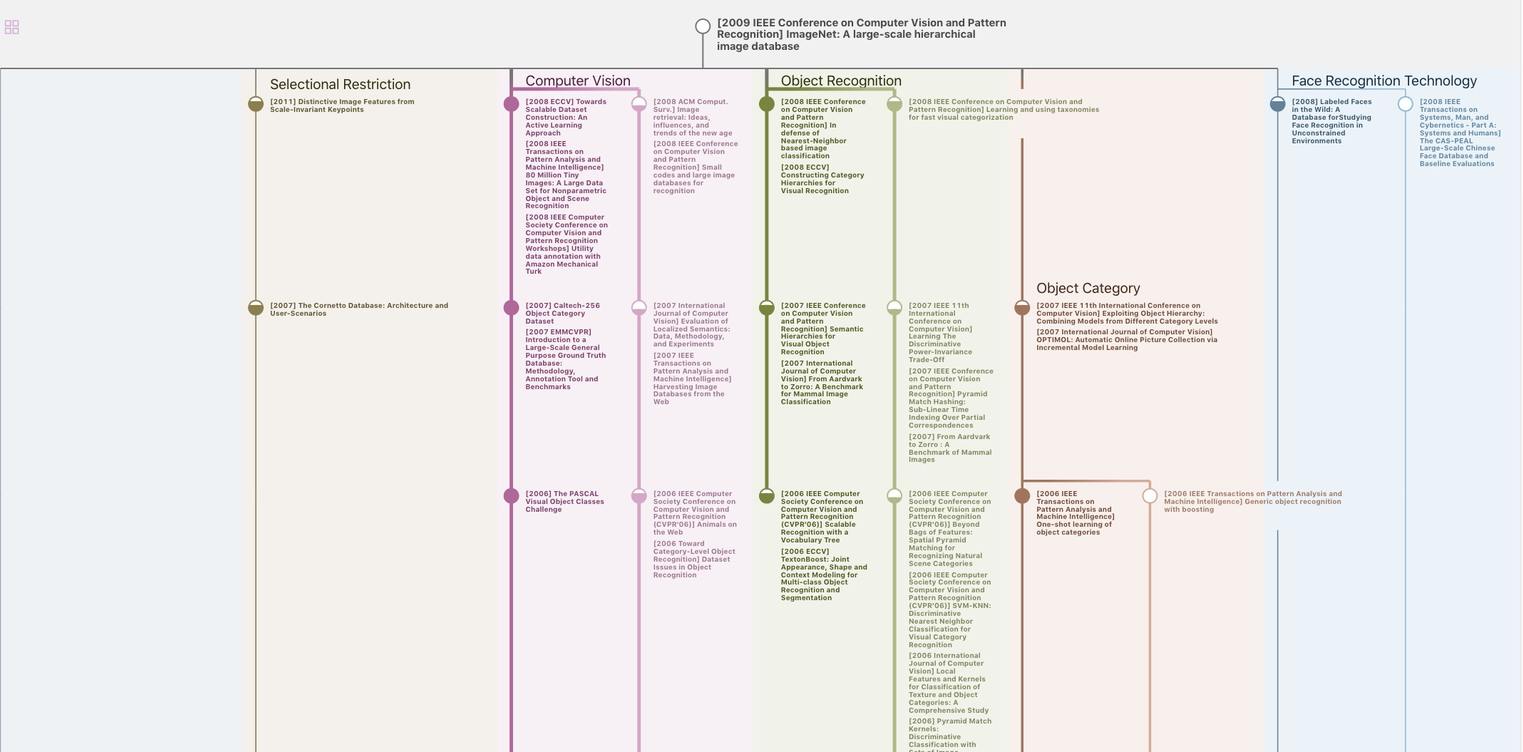
生成溯源树,研究论文发展脉络
Chat Paper
正在生成论文摘要