Hugs Bring Double Benefits: Unsupervised Cross-Modal Hashing with Multi-granularity Aligned Transformers
International Journal of Computer Vision(2024)
摘要
Unsupervised cross-modal hashing (UCMH) has been commonly explored to support large-scale cross-modal retrieval of unlabeled data. Despite promising progress, most existing approaches are developed on convolutional neural network and multilayer perceptron architectures, sacrificing the quality of hash codes due to limited capacity for excavating multi-modal semantics. To pursue better content understanding, we break this convention for UCMH and delve into a transformer-based paradigm. Unlike naïve adaptations via backbone substitution that overlook the heterogeneous semantics from transformers, we propose a multi-granularity learning framework called hugging to bridge the modality gap. Specifically, we first construct a fine-grained semantic space composed of a series of aggregated local embeddings that capture implicit attribute-level semantics. In the hash learning stage, we innovatively incorporate fine-grained alignment with these local embeddings to enhance global hash code alignment. Notably, this fine-grained alignment only facilitates robust cross-modal learning without complicating global hash code generation at test time, thus fully maintaining the high efficiency of hash-based retrieval. To make the most of fine-grained information, we further propose a differentiable optimized quantization algorithm and extend our framework to hugging ^+ . This variant neatly integrates quantization learning into the fine-grained alignment during training, producing quantization codes of local embeddings as a gift at test time, which can augment the retrieval performance through an efficient reranking stage. We instantiate simple baselines with contrastive learning objectives for hugging and hugging ^+ , namely HuggingHash and HuggingHash ^+ . Extensive experiments on 4 text-image retrieval and 2 text-video retrieval benchmark datasets show the competitive performance of HuggingHash and HuggingHash ^+ against state-of-the-art baselines. More encouragingly, we also validate that hugging and hugging ^+ are flexible and effective across various baselines, suggesting their universal applicability in the realm of UCMH.
更多查看译文
关键词
Unsupervised cross-modal hashing (UCMH),Transformers,Image retrieval,Video retrieval,Vector of locally aggregated descriptors (VLAD),Optimized product quantization (OPQ)
AI 理解论文
溯源树
样例
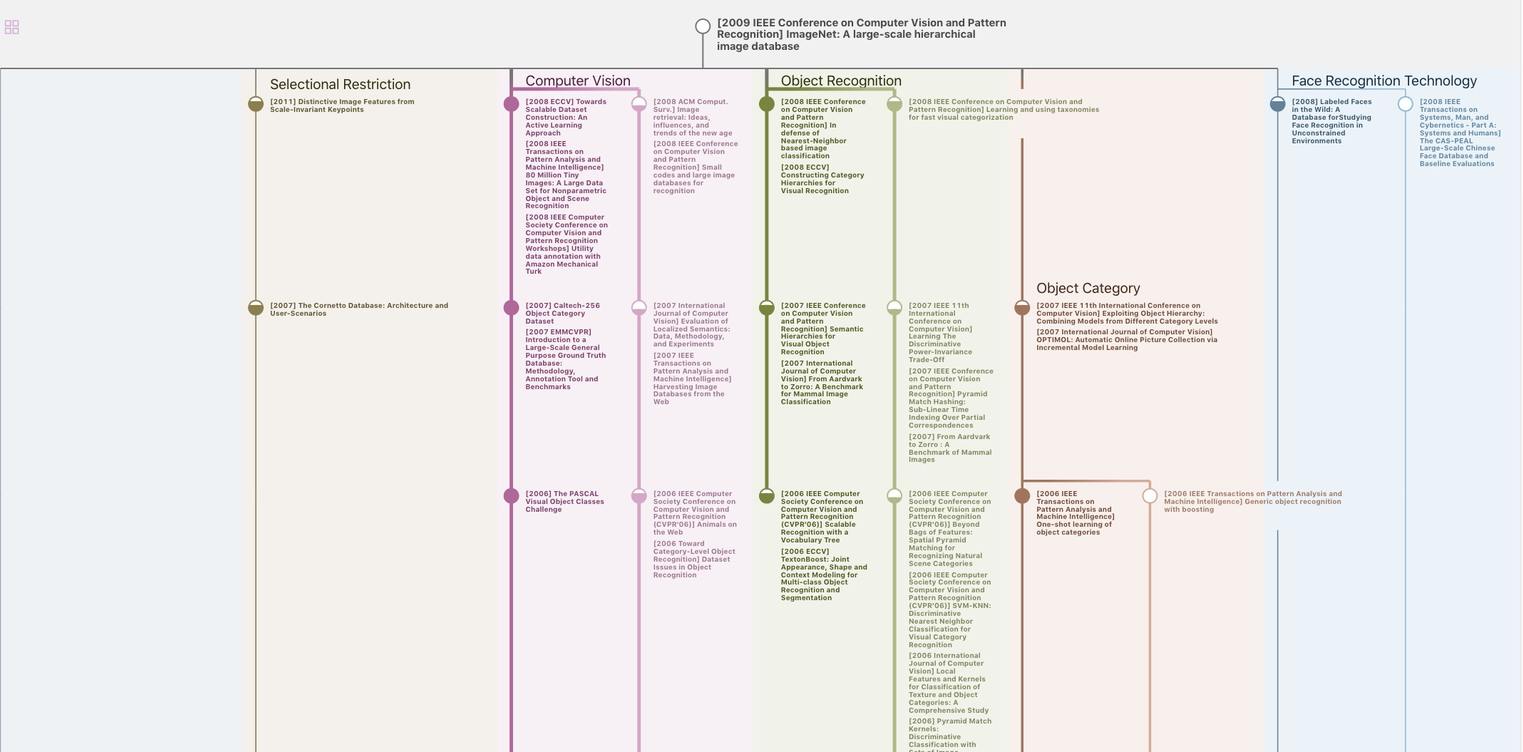
生成溯源树,研究论文发展脉络
Chat Paper
正在生成论文摘要