One-Bit Quantization and Sparsification for Multiclass Linear Classification via Regularized Regression
CoRR(2024)
摘要
We study the use of linear regression for multiclass classification in the
over-parametrized regime where some of the training data is mislabeled. In such
scenarios it is necessary to add an explicit regularization term, λ
f(w), for some convex function f(·), to avoid overfitting the mislabeled
data. In our analysis, we assume that the data is sampled from a Gaussian
Mixture Model with equal class sizes, and that a proportion c of the training
labels is corrupted for each class. Under these assumptions, we prove that the
best classification performance is achieved when f(·) = ·^2_2 and
λ→∞. We then proceed to analyze the classification errors for
f(·) = ·_1 and f(·) = ·_∞ in the large
λ regime and notice that it is often possible to find sparse and
one-bit solutions, respectively, that perform almost as well as the one
corresponding to f(·) = ·_2^2.
更多查看译文
AI 理解论文
溯源树
样例
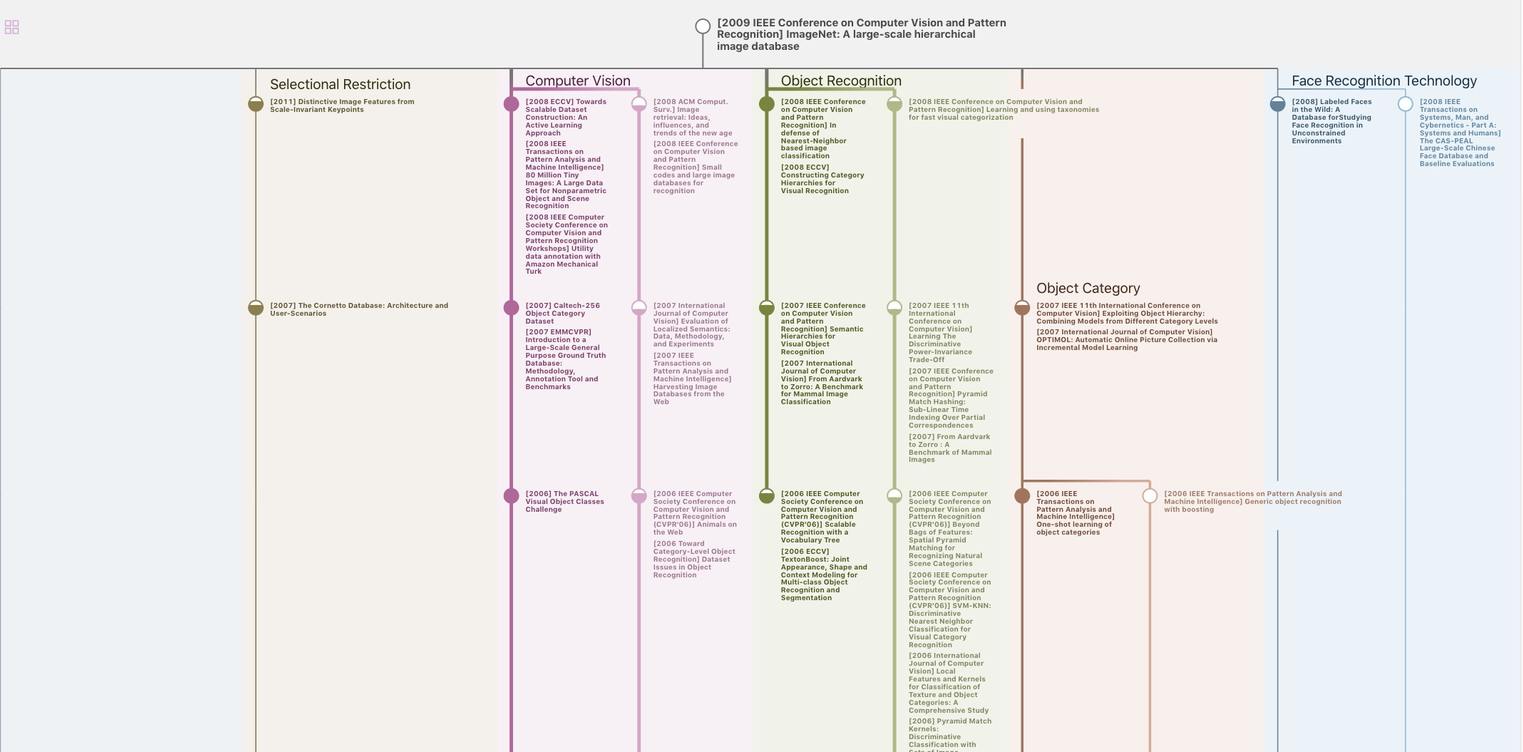
生成溯源树,研究论文发展脉络
Chat Paper
正在生成论文摘要