K-Means Featurizer: A booster for intricate datasets
Earth Science Informatics(2024)
摘要
Machine Learning (ML) has become pivotal across various fields, offering innovative solutions to complex data challenges. Professionals typically seek models that excel in both performance and reliability, aiming to achieve optimal generalization on future data. Since, then a variety of methods such as dummy coding, up/down-sampling, and bin-counting have been explored. However, finding a solution that effectively navigates the intricacies of limited and complex datasets still remains a challenge. This study introduces the K-Means Featurizer (KMF), an innovative algorithm crafted to enhance model performance and reliability, especially in scenarios involving complex and limited datasets. KMF employs K-Means clustering to generate enriched features that provide a nuanced understanding of the data, effectively balancing the similarity between the target variable and the feature space. This results in a more efficient predictive task by minimizing Euclidean distances and enhancing model generalizability. Our research validates KMF's effectiveness through an experiment in geoscience engineering, focusing on hydraulic conductivity (K) prediction, a vital parameter in well monitoring and infrastructure planning. Traditionally, K extraction is laborious and costly, requiring extensive pumping tests. KMF's application in this context demonstrates its potential to substantially reduce data losses during such operations. Applying KMF to the Extreme Gradient Boosting, Random Forest, K-Neighbors, Support Vector Machines, and Multiple Layers Neural Networks resulted in a significant improvement in prediction accuracy, with K-scores reaching up to 90%. While our experiment centers on geoscience engineering, KMF's utility extends to various domains facing similar data intricacies. Its adaptability to different types of complex datasets positions it as a valuable tool for diverse data-driven applications.
更多查看译文
关键词
Machine Learning,K-Means,Data Generalization,Predictive Modeling,Geoscience Engineering,Hydraulic Conductivity
AI 理解论文
溯源树
样例
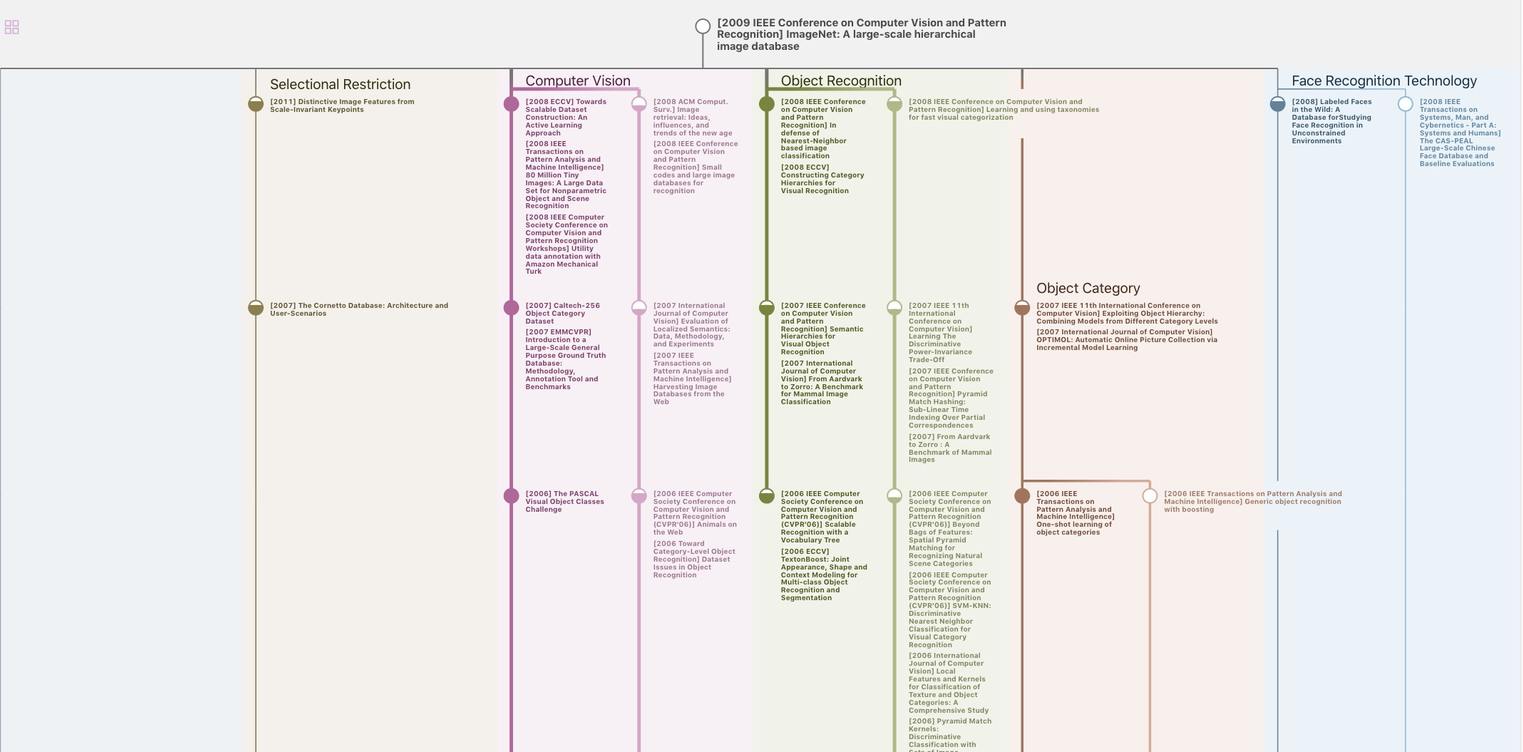
生成溯源树,研究论文发展脉络
Chat Paper
正在生成论文摘要