Judging Knowledge by its Cover: Leveraging Large Language Models in Establishing Criteria for Knowledge Graph Sources Selection
2023 8th International Conference on Information Technology and Digital Applications (ICITDA)(2023)
摘要
The Knowledge Graph (KG) is a widely used paradigm for knowledge representation that leverages graph structures. It facilitates the discovery of relationships and provides context across various domains. Despite its popularity, there's a notable lack of emphasis on quality control during the KG construction process, particularly concerning the selection of knowledge sources. Our paper addresses this research gap by introducing criteria for selecting high-quality websites during the data acquisition phase of KG construction. We propose a set of criteria—credibility, relevance, content quality, coverage, comprehensiveness, and accessibility—that serves as a benchmark for evaluating potential online knowledge sources for KGs. We determine the weight of each criterion through expert judgment and validation using Large Language Models (LLMs) representing AI technologies. Our panel, consisting of academicians and practitioners, ranks the criteria by importance, with the Rank Ordered Centroid and Rank Reciprocal weighting methods generating weights from these rankings. We employ four LLMs: Google Bard, OpenAI ChatGPT 4, Anthropic Claude-2, and Meta Llama-2. Our findings suggest that LLMs can effectively validate human weighting results, as evidenced by a high correlation (Pearson's
$r > 0.9$
) between human and LLM criterion weights. Furthermore, hypothesis testing reveals no significant differences (
$p > 0.8$
), with the models demonstrating high internal consistency. Our study offers valuable insights for academics and industry professionals seeking to improve KG construction processes and establish standards for data quality and verification.
更多查看译文
关键词
knowledge graphs construction,knowledge source selection,large language models,website evaluation criteria,criteria development approach
AI 理解论文
溯源树
样例
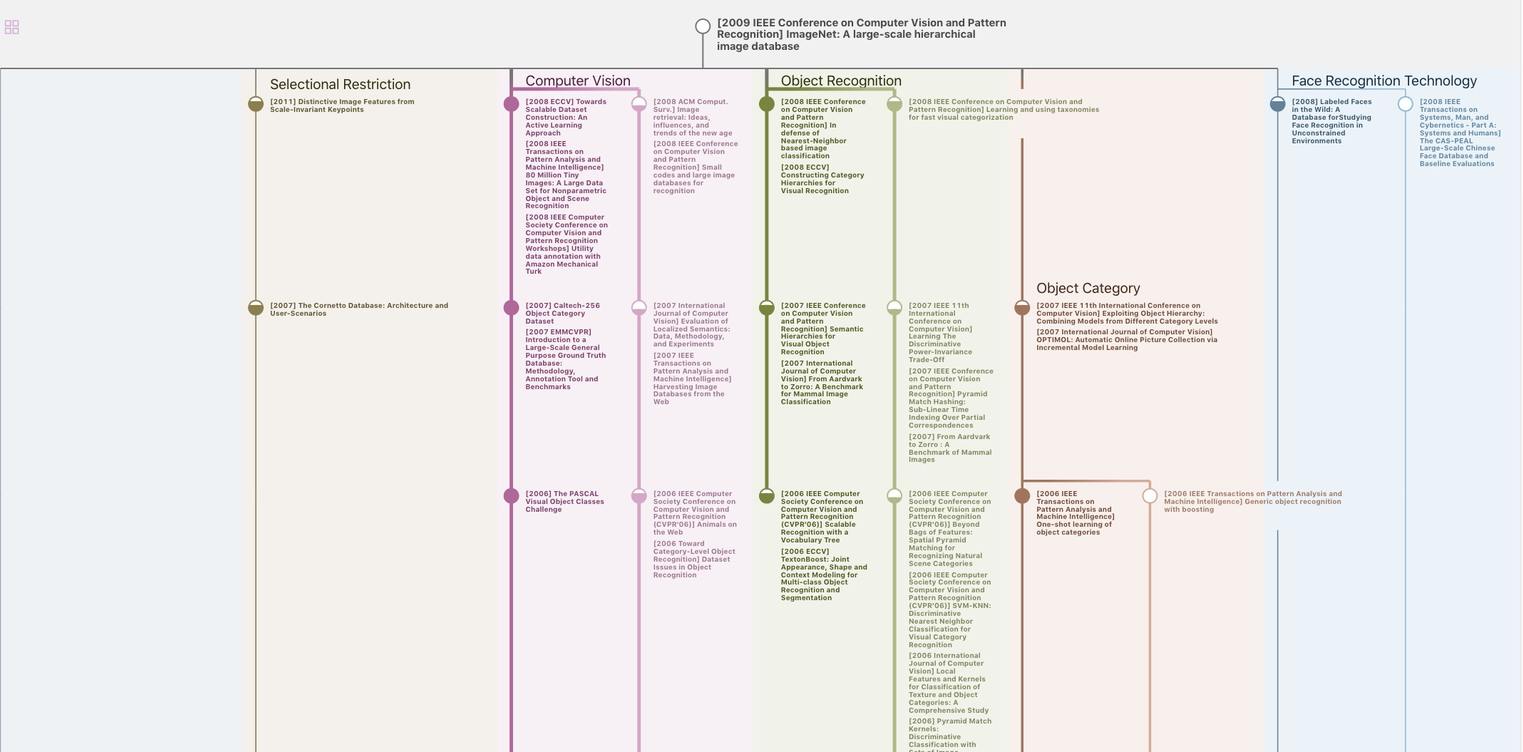
生成溯源树,研究论文发展脉络
Chat Paper
正在生成论文摘要