Geometric deep learning for the prediction of magnesium-binding sites in RNA structures
INTERNATIONAL JOURNAL OF BIOLOGICAL MACROMOLECULES(2024)
摘要
Magnesium ions (Mg2+) are essential for the folding, functional expression, and structural stability of RNA molecules. However, predicting Mg2+-binding sites in RNA molecules based solely on RNA structures is still challenging. The molecular surface, characterized by a continuous shape with geometric and chemical properties, is important for RNA modelling and carries essential information for understanding the interactions between RNAs and Mg2+ ions. Here, we propose an approach named RNA-magnesium ion surface interaction fingerprinting (RMSIF), a geometric deep learning -based conceptual framework to predict magnesium ion binding sites in RNA structures. To evaluate the performance of RMSIF, we systematically enumerated decoy Mg2+ ions across a full -space grid within the range of 2 to 10 angstrom from the RNA molecule and made predictions accordingly. Visualization techniques were used to validate the prediction results and calculate success rates. Comparative assessments against state-of-the-art methods like MetalionRNA, MgNet, and Metal3DRNA revealed that RMSIF achieved superior success rates and accuracy in predicting Mg2+-binding sites. Additionally, in terms of the spatial distribution of Mg2+ ions within the RNA structures, a majority were situated in the deep grooves, while a minority occupied the shallow grooves. Collectively, the conceptual framework developed in this study holds promise for advancing insights into drug design, RNA co -transcriptional folding, and structure prediction.
更多查看译文
关键词
Molecular fingerprints,Geometric deep learning,Magnesium binding site,RNA
AI 理解论文
溯源树
样例
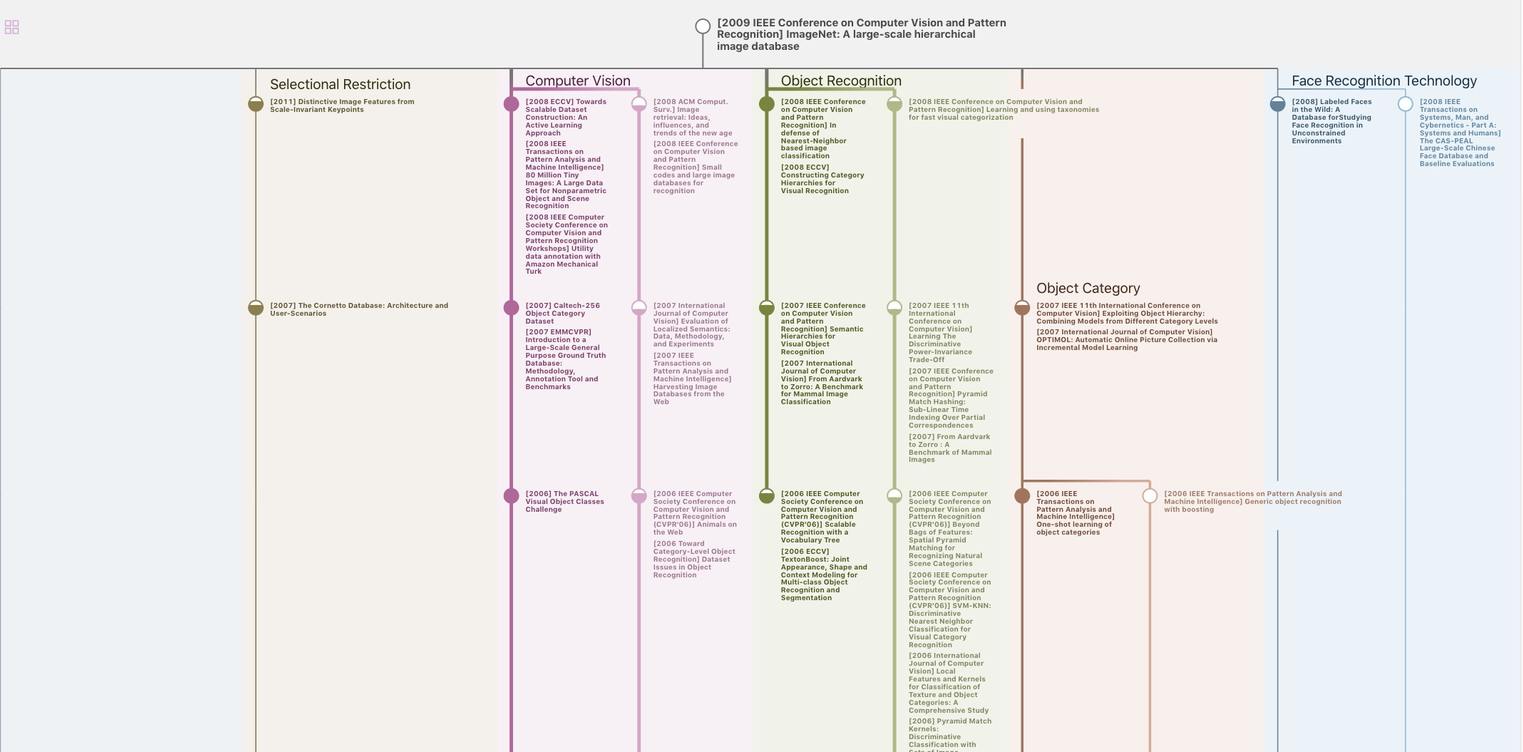
生成溯源树,研究论文发展脉络
Chat Paper
正在生成论文摘要