Predicting coal workers' pneumoconiosis trends: Leveraging historical data with the GARCH model in a Chinese Miner Cohort
MEDICINE(2024)
Abstract
Coal workers' pneumoconiosis (CWP) is one of the most common and severe occupational diseases worldwide. The main risk factor of CWP is exposure to respirable mine dust. Prediction theory was widely applied in the prediction of the epidemic. Here, it was used to identify the characteristics of CWP today and the incidence trends of CWP in the future. Eight thousand nine hundred twenty-eight coal workers from a state-owned coal mine were included during the observation period from 1963 to 2014. In observations, the dust concentration gradually decreased over time, and the incidence of tunnels and mine, transportation, and assistance workers showed an overall downward trend. We choose a better prediction model by comparing the prediction effect of the Auto Regression Integrate Moving Average model and Generalized Autoregressive Conditional Heteroskedasticity model. Compared with the Auto Regression Integrate Moving Average model, the Generalized Autoregressive Conditional Heteroskedasticity model has a better prediction effect. Furthermore, the status quo and future trend of coal miners' CWP are still at a high level.
MoreTranslated text
Key words
ARIMA,coal worker's pneumoconiosis,GARCH,prediction
AI Read Science
Must-Reading Tree
Example
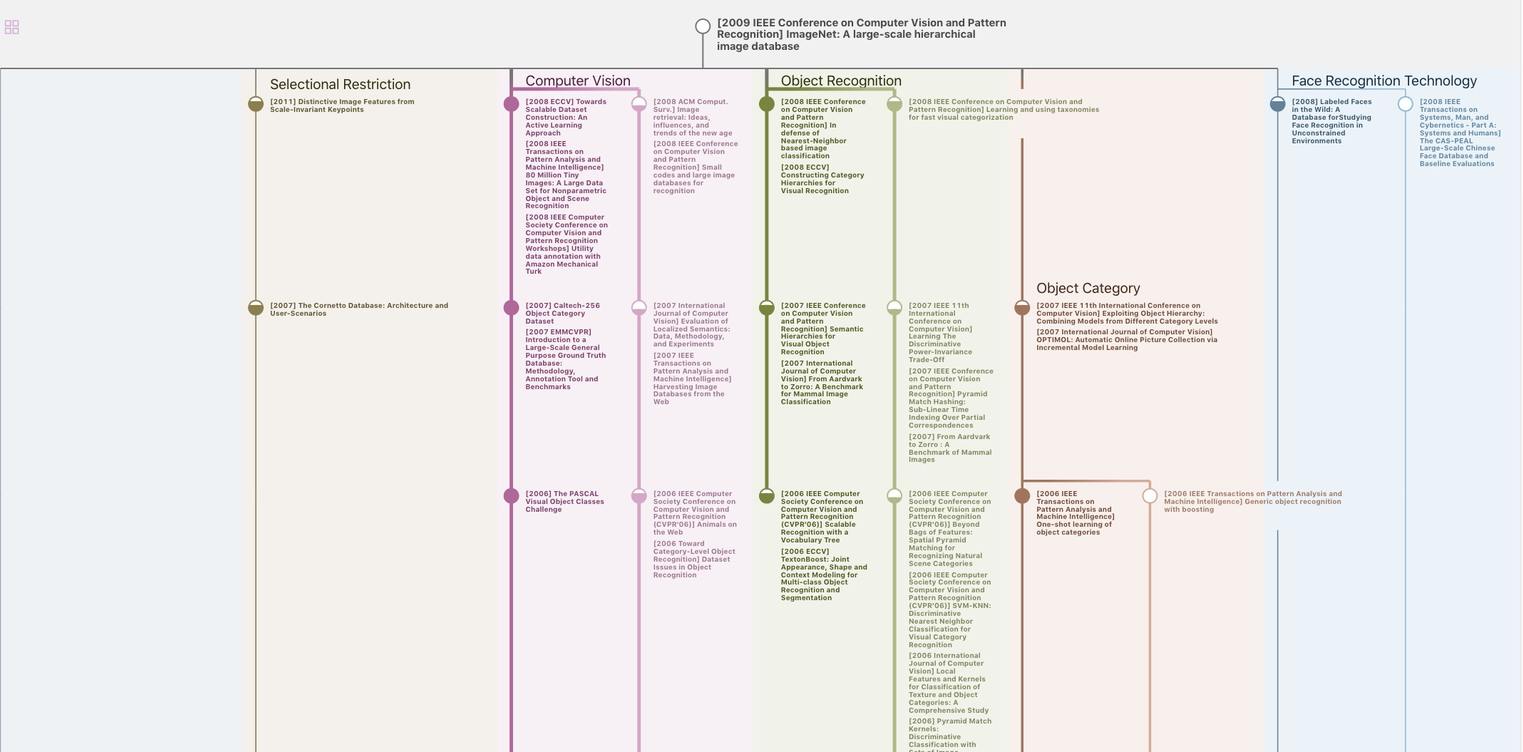
Generate MRT to find the research sequence of this paper
Chat Paper
Summary is being generated by the instructions you defined