A Privacy-Preserving Framework for Collaborative Machine Learning with Kernel Methods.
International Conference on Trust, Privacy and Security in Intelligent Systems and Applications(2023)
摘要
It is challenging to implement Kernel methods, if the data sources are distributed and cannot be joined at a trusted third party for privacy reasons. It is even more challenging, if the use case rules out privacy-preserving approaches that introduce noise or entail significant computational overhead. An example for such a use case is machine learning on clinical data. To realize exact and efficient privacy preserving computation of kernel methods, we propose FLAKE, a Framework for Learning with Anonymized KErnels on horizontally distributed data. With our method, the data sources mask their data so that a Gram matrix can be computed without compromising privacy or utility. The Gram matrix allows to calculate many kernel matrices, which can be used to train kernel-based machine learning algorithms such as Support Vector Machines. We prove that our framework prevents an adversary from learning the input data or the number of input features under a semi-honest threat model. The conducted experiments on clinical, genomic, and image data provide confirmation that our approach is applicable across a wide range of settings. Additionally, our method outperforms comparable approaches in both computational efficiency and accuracy. Thus, FLAKE is a lightweight, applicable approach suitable for various use cases.
更多查看译文
关键词
Privacy,Kernel-based Machine Learning,Distributed Learning
AI 理解论文
溯源树
样例
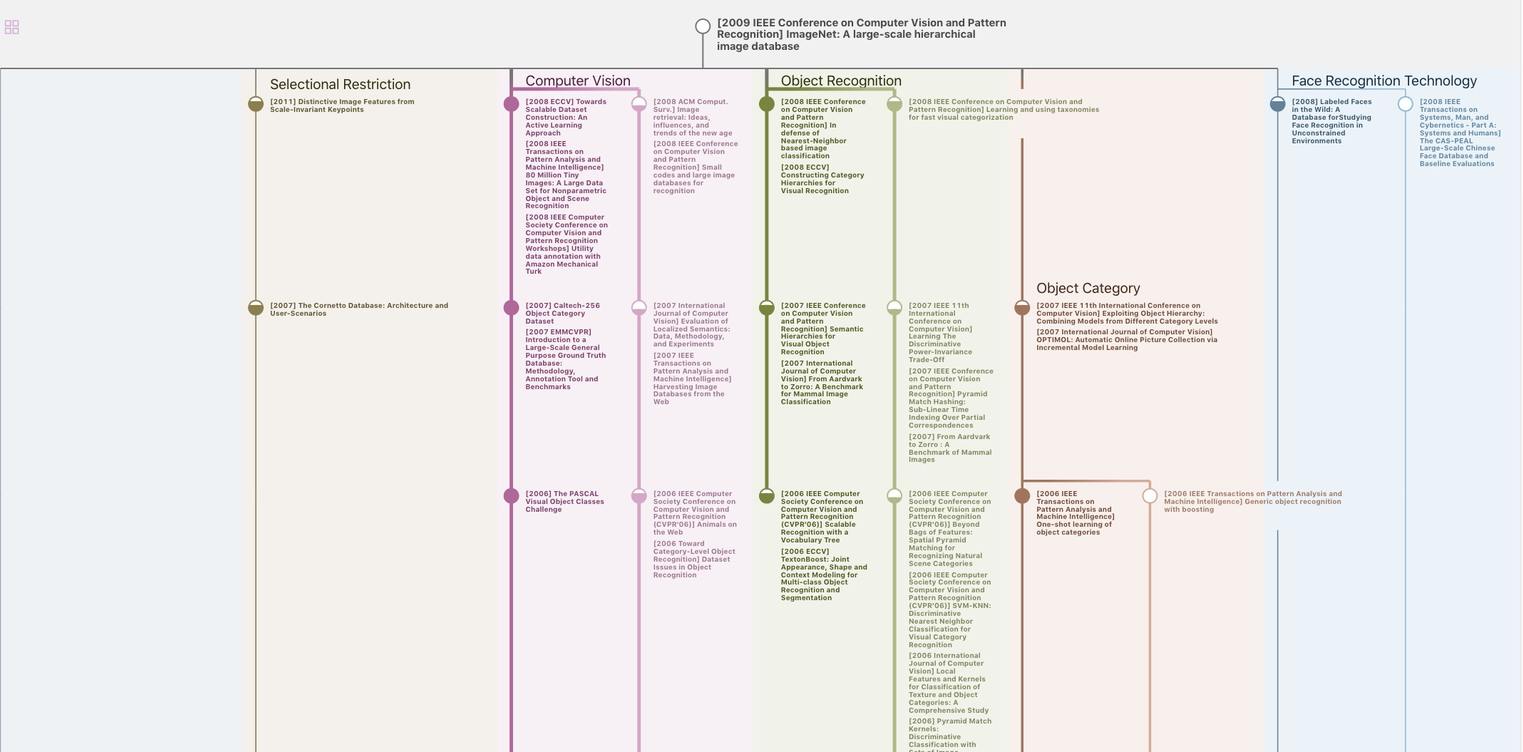
生成溯源树,研究论文发展脉络
Chat Paper
正在生成论文摘要