Optimizing cell selection for data services in mm-waves spectrum through enhanced extreme gradient boosting
RESULTS IN ENGINEERING(2024)
摘要
This paper proposes a supervised learning based cell selection/reselection prediction technique incorporating ensembles, and is reinforced by readily available lasso and ridge regression for regularization. This data-driven approach replaces event-driven cell selection/reselection requests and helps in solving complex calculations for generalizing the loss function. The proposed work uses the term "cell selection for affluence" to refer to both cell selection and reselection. In technical terms, handovers are reserved for calls, and cell selections are for data services. This study highlights that by exploiting advance information about the success or failure of cell selection request, how a data-driven cell selection can improve the cell selection success rate of future mobile networks. Our results demonstrate, the proposed algorithm can improve cell selection success rate by up to 4% and quickens model response time by up to 602 ms.
更多查看译文
关键词
5G and beyond,Data -driven,Artificial intelligence,Cell selection,Mm -waves
AI 理解论文
溯源树
样例
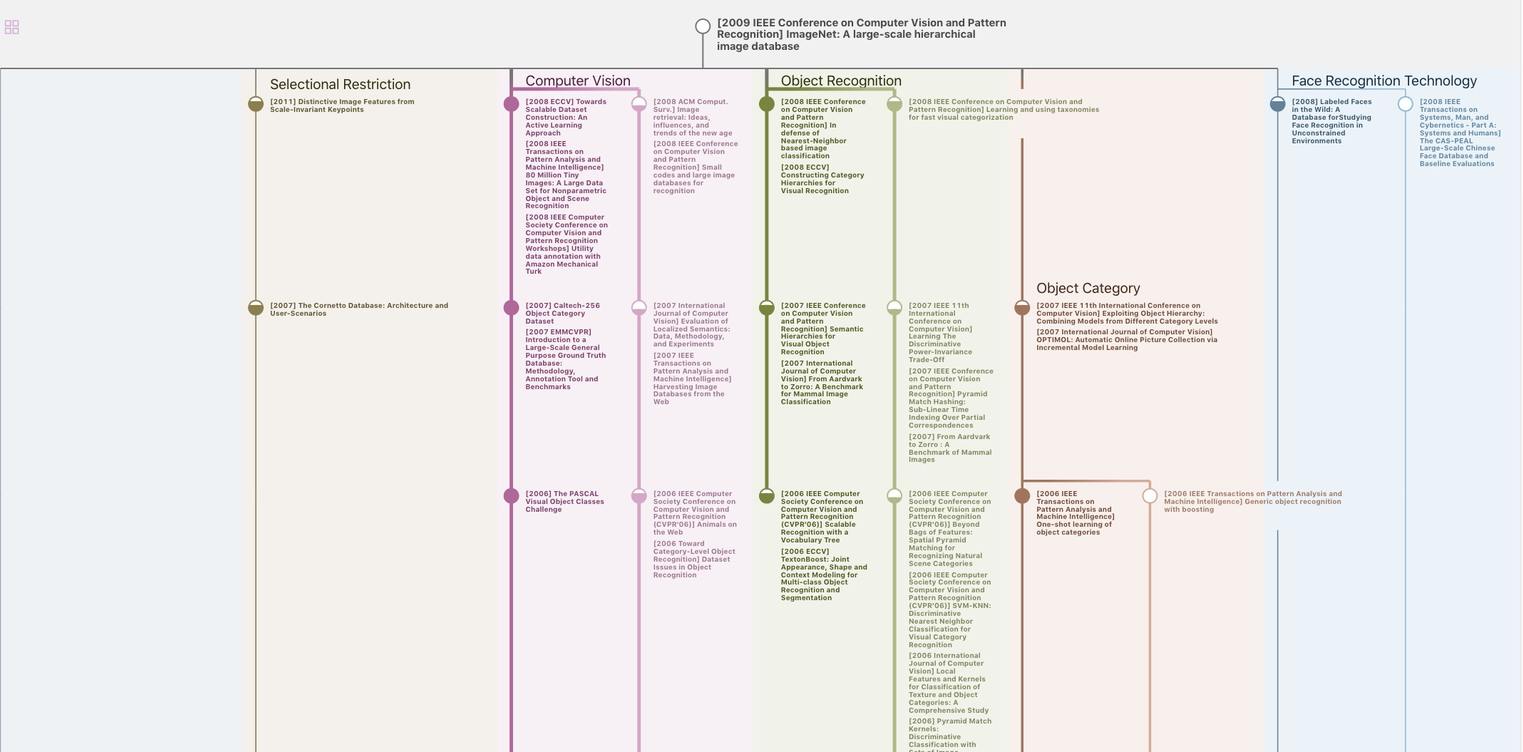
生成溯源树,研究论文发展脉络
Chat Paper
正在生成论文摘要