Fast Bayesian Linearized Inversion With an Efficient Dimension Reduction Strategy
IEEE TRANSACTIONS ON GEOSCIENCE AND REMOTE SENSING(2024)
摘要
Bayesian linearized inversion (BLI) stands out as an exceptional stochastic inversion method in the realms of geophysics and remote sensing. It excels in estimating inversion results and assessing their uncertainty with remarkable efficiency. However, one of the challenges faced by BLI lies in the inversion of its core matrix. To surmount this limitation, an innovative dimension reduction strategy is proposed based on the discrete cosine transform (DCT), thus formulating a rapid BLI approach termed DCT-BLI. Within this method, the DCT-based reduction strategy effectively compresses a large sparse matrix by extracting its essential information, transforming the inversion of this sizable matrix into the inversion of a reduced-size counterpart. A compression factor (CF), defined as the size ratio of matrices after and before reduction, quantifies the extent of matrix reduction. DCT-BLI integrates the strengths of both BLI and the DCT-based reduction strategy. Leveraging this reduction approach, DCT-BLI tackles the challenge of inverting its sizable core matrix. Through the synthetic and field data tests, DCT-BLI exhibits clear superiority over BLI in terms of efficiency, and the DCT-based reduction method achieves a remarkable two-thirds reduction in the core matrix size of BLI without compromising inversion accuracy.
更多查看译文
关键词
Bayesian inversion,dimension reduction,matrix inverse,stochastic inversion
AI 理解论文
溯源树
样例
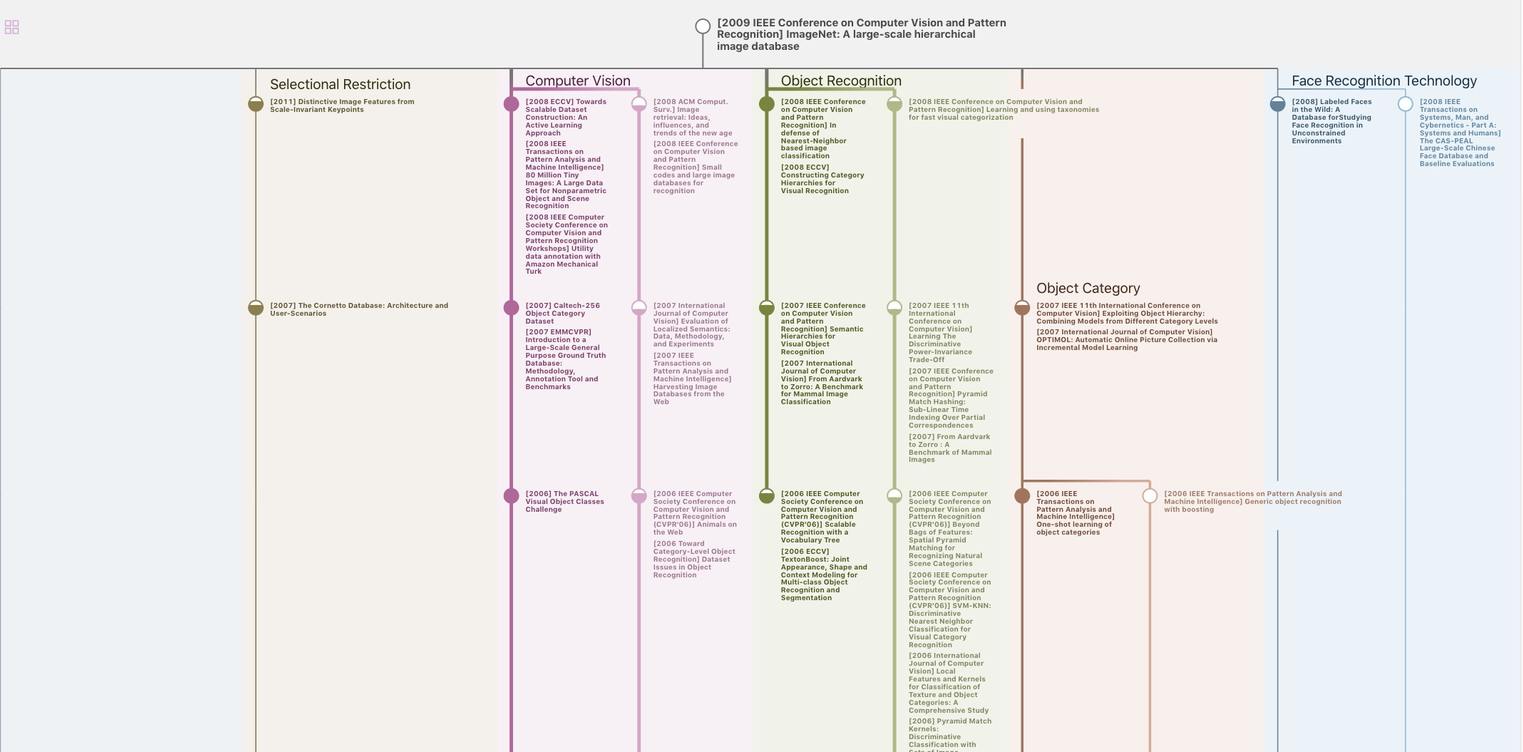
生成溯源树,研究论文发展脉络
Chat Paper
正在生成论文摘要