A Lightweight Transformer With Multigranularity Tokens and Connected Component Loss for Land Cover Classification
IEEE TRANSACTIONS ON GEOSCIENCE AND REMOTE SENSING(2024)
摘要
Onboard land cover classification provides ever-updating land cover information, supporting various intelligent satellite applications that demand timely autonomous decision-making based on current and continuous land cover data. However, due to space, weight, and power constraints, satellites possess limited computational resources, rendering them unable to execute conventional land cover classification networks. In response to this challenge, we have designed a lightweight network for land cover classification featuring two efficient transformer attention mechanisms enhanced by multigranularity tokens. Diverging from traditional transformer attention mechanisms that solely capture token-to-token correlations at a single granularity, our approach splits the tokens into four segments and uses atrous convolutions across various dilation rates to aggregate token segments from diverse receptive fields, forming token segment combinations that encompass not only point information but also information from patches of varying sizes. These multigranularity tokens are subsequently processed through the windowed squeeze axial transformer attention (WSATA) and multigranularity bilevel routing attention (MGBRA) for feature enhancement. In another aspect, empirical observations reveal that prediction errors are more prone to manifest on land covers of small extent; however, conventional methods treat all pixels uniformly. This realization motivates us to propose a novel network-agnostic loss named connected component loss (CCL), which specifically targets small-scale land covers and their boundaries. Quantitative metrics and visual interpretations from comprehensive experiments confirm that our method attains state-of-the-art accuracy on two land cover classification datasets while exhibiting significantly faster inference speed than other lightweight networks, underscoring the practical potential of our method on embedded systems.
更多查看译文
关键词
Land cover classification,lightweight neural network,semantic segmentation,transformer
AI 理解论文
溯源树
样例
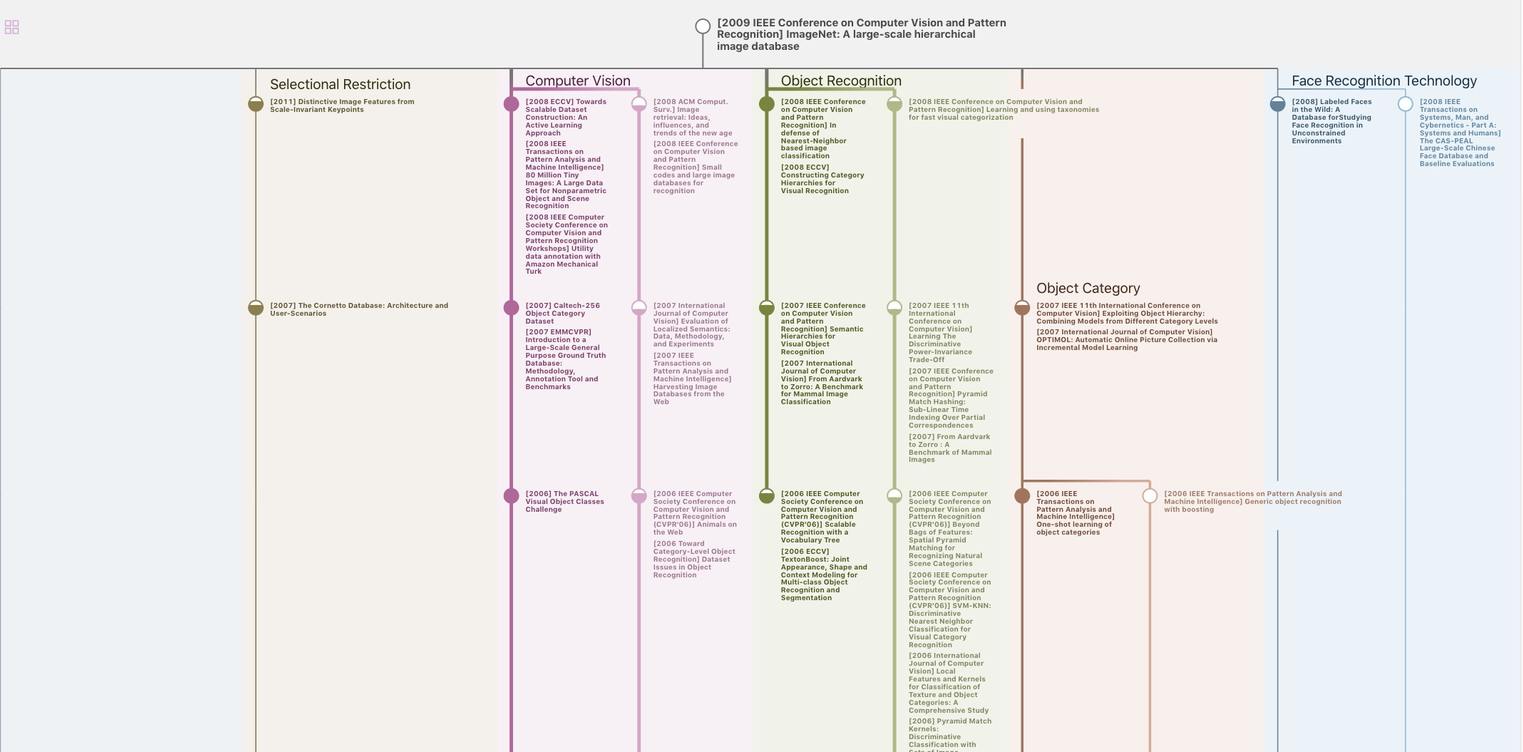
生成溯源树,研究论文发展脉络
Chat Paper
正在生成论文摘要