Robustness Analysis and Evaluation Study of Chinese Text Event Detection Models
2023 9th International Conference on Big Data and Information Analytics (BigDIA)(2023)
摘要
Event detection aims to identify trigger words in given text and classify them into corresponding event types, making it an essential task in natural language processing. With the advancement of artificial intelligence, machine learning, and deep learning techniques have been further researched and applied in the field of event detection. However, current event detection methods heavily rely on manually labeled data, which is constrained by high-quality data requirements. When facing challenges such as imbalanced sample distribution, low-quality data labeling, and adversarial data contamination, the effectiveness of models is often compromised, leading to a lack of robustness and poor generalization capabilities. This significantly limits the universal applicability of event detection algorithms. Therefore, the robustness evaluation of text event detection algorithms is of paramount importance. In this paper, we have designed various text perturbation robustness datasets based on the characteristics of Chinese text and developed evaluation metrics specifically for robustness datasets to measure algorithm robustness. Building upon the robustness datasets, we have devised a resilient analysis framework. We have constructed a text event detection algorithm using multi-layer perceptrons, convolutional neural networks, and BERT. By comparing the model’s evaluation results on the robustness test dataset with the high-quality initial dataset, we intuitively reflect the model’s robustness. This approach holds strong theoretical value for further enhancing the model’s generalization capabilities.
更多查看译文
关键词
Event detection,Deep learning,Neural network,Robustness,Confrontation sample
AI 理解论文
溯源树
样例
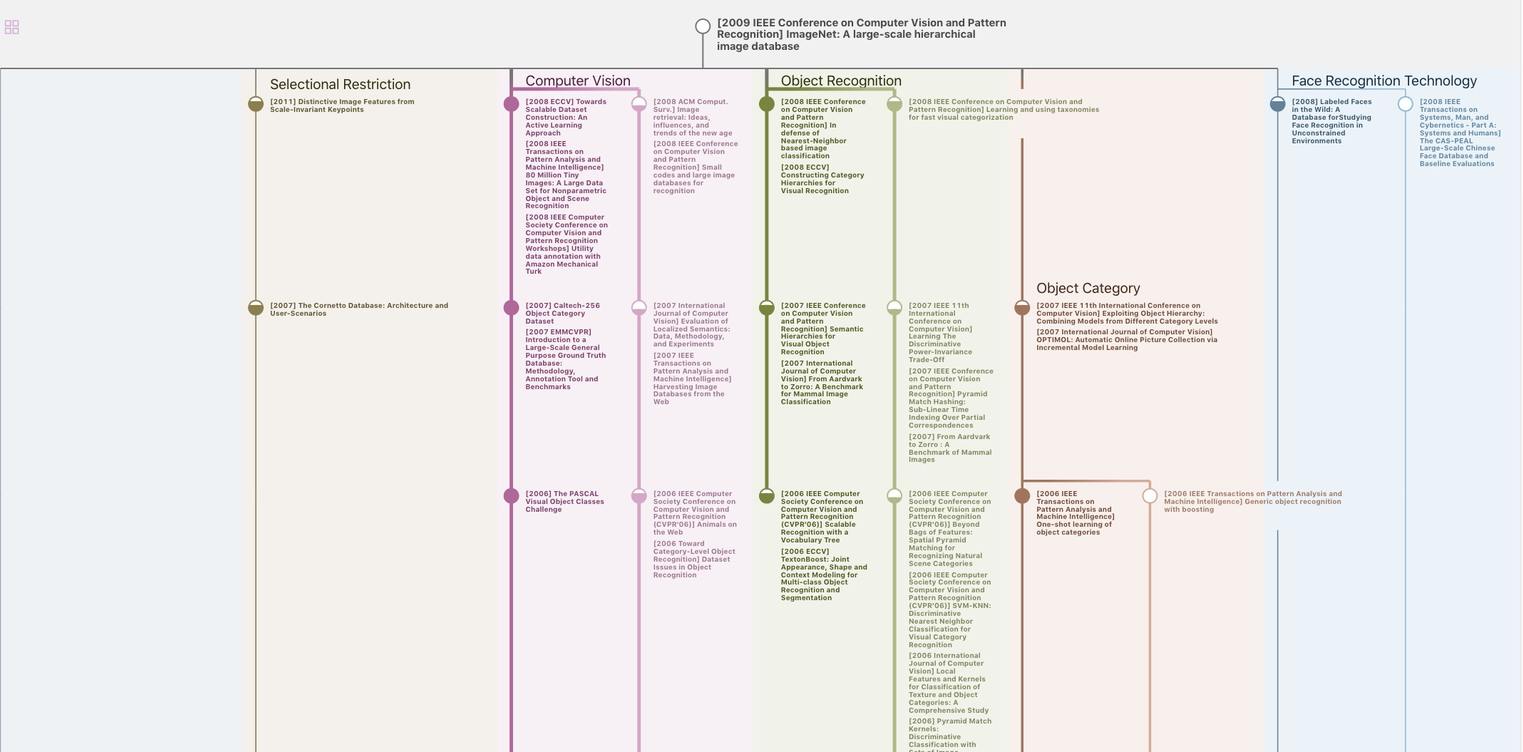
生成溯源树,研究论文发展脉络
Chat Paper
正在生成论文摘要