The development and evaluation of dose-prediction tools for allopurinol therapy (Easy-Allo tools)
BRITISH JOURNAL OF CLINICAL PHARMACOLOGY(2024)
摘要
Aims: Dose escalation at the initiation of allopurinol therapy can be protracted and resource intensive. Tools to predict the allopurinol doses required to achieve target serum urate concentrations would facilitate the implementation of more efficient dose-escalation strategies. The aim of this research was to develop and externally evaluate allopurinol dosing tools, one for use when the pre-urate-lowering therapy serum urate is known (Easy-Allo1) and one for when it is not known (Easy-Allo2). Methods: A revised population pharmacokinetic-pharmacodynamic model was developed using data from 653 people with gout. Maintenance doses to achieve the serum urate target of <0.36 mmol L-1 in >80% of individuals were simulated and evaluated against external data. The predicted and observed allopurinol doses were compared using the mean prediction error (MPE) and root mean square error (RMSE). The proportion of Easy-Allo predicted doses within 100 mg of the observed was quantified. Results: Allopurinol doses were predicted by total body weight, baseline urate, ethnicity and creatinine clearance. Easy-Allo1 produced unbiased and suitably precise dose predictions (MPE 2 mg day-1 95% confidence interval [CI] -13-17, RMSE 91%, 90% within 100 mg of the observed dose). Easy-Allo2 was positively biased by about 70 mg day-1 and slightly less precise (MPE 70 mg day-1 95% CI 52-88, RMSE 131%, 71% within 100 mg of the observed dose). Conclusions: The Easy-Allo tools provide a guide to the allopurinol maintenance dose requirement to achieve the serum urate target of <0.36 mmol L-1 and will aid in the development of novel dose-escalation strategies for allopurinol therapy.
更多查看译文
关键词
allopurinol,dose,gout,NONMEM,pharmacokinetic-pharmacodynamic
AI 理解论文
溯源树
样例
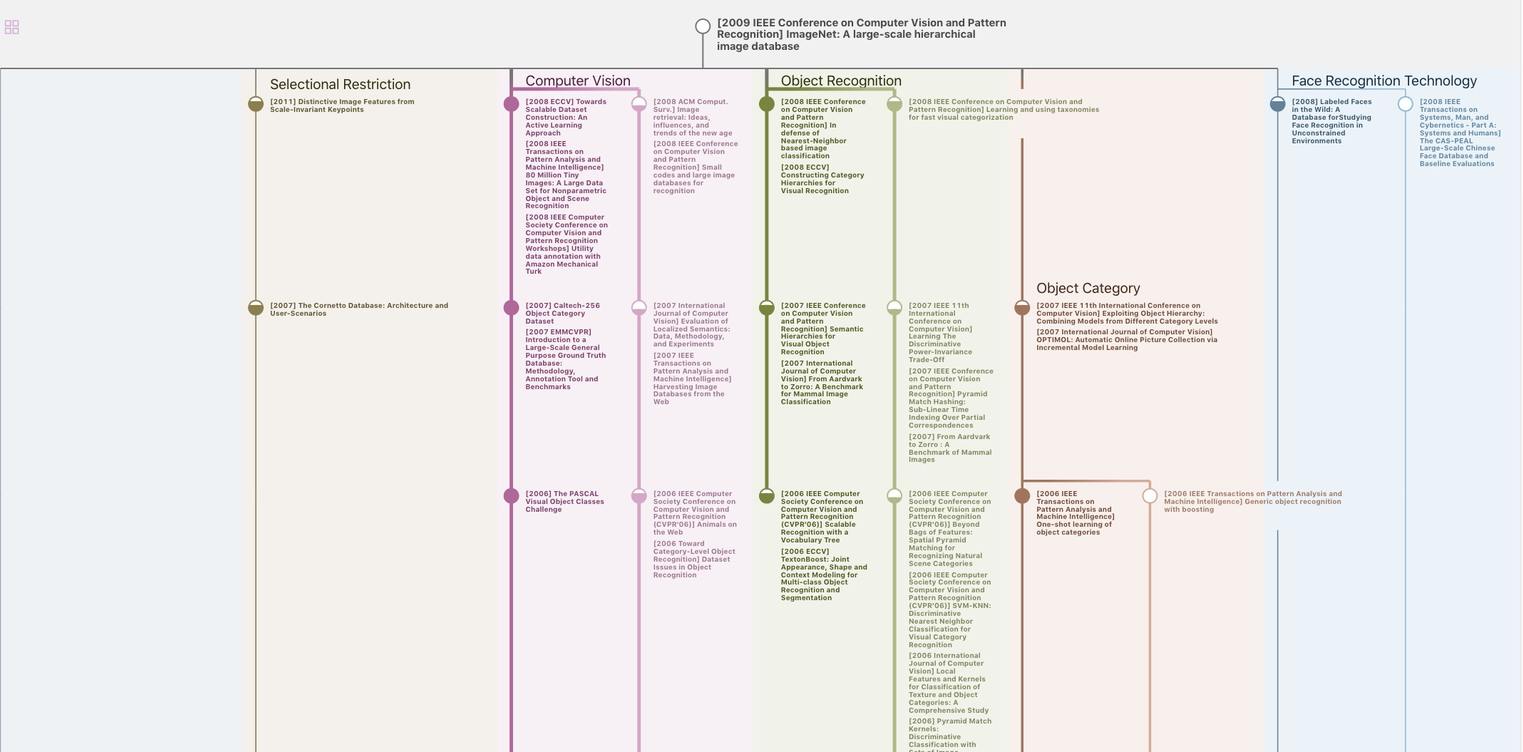
生成溯源树,研究论文发展脉络
Chat Paper
正在生成论文摘要