Mitigating allocative tradeoffs and harms in an environmental justice data tool
Nature Machine Intelligence(2024)
Abstract
Neighbourhood-level screening algorithms are increasingly being deployed to inform policy decisions. However, their potential for harm remains unclear: algorithmic decision-making has broadly fallen under scrutiny for disproportionate harm to marginalized groups, yet opaque methodology and proprietary data limit the generalizability of algorithmic audits. Here we leverage publicly available data to fully reproduce and audit a large-scale algorithm known as CalEnviroScreen, designed to promote environmental justice and guide public funding by identifying disadvantaged neighbourhoods. We observe the model to be both highly sensitive to subjective model specifications and financially consequential, estimating the effect of its positive designations as a 104% (62–145%) increase in funding, equivalent to US$2.08 billion (US$1.56–2.41 billion) over four years. We further observe allocative tradeoffs and susceptibility to manipulation, raising ethical concerns. We recommend incorporating technical strategies to mitigate allocative harm and accountability mechanisms to prevent misuse.
MoreTranslated text
AI Read Science
Must-Reading Tree
Example
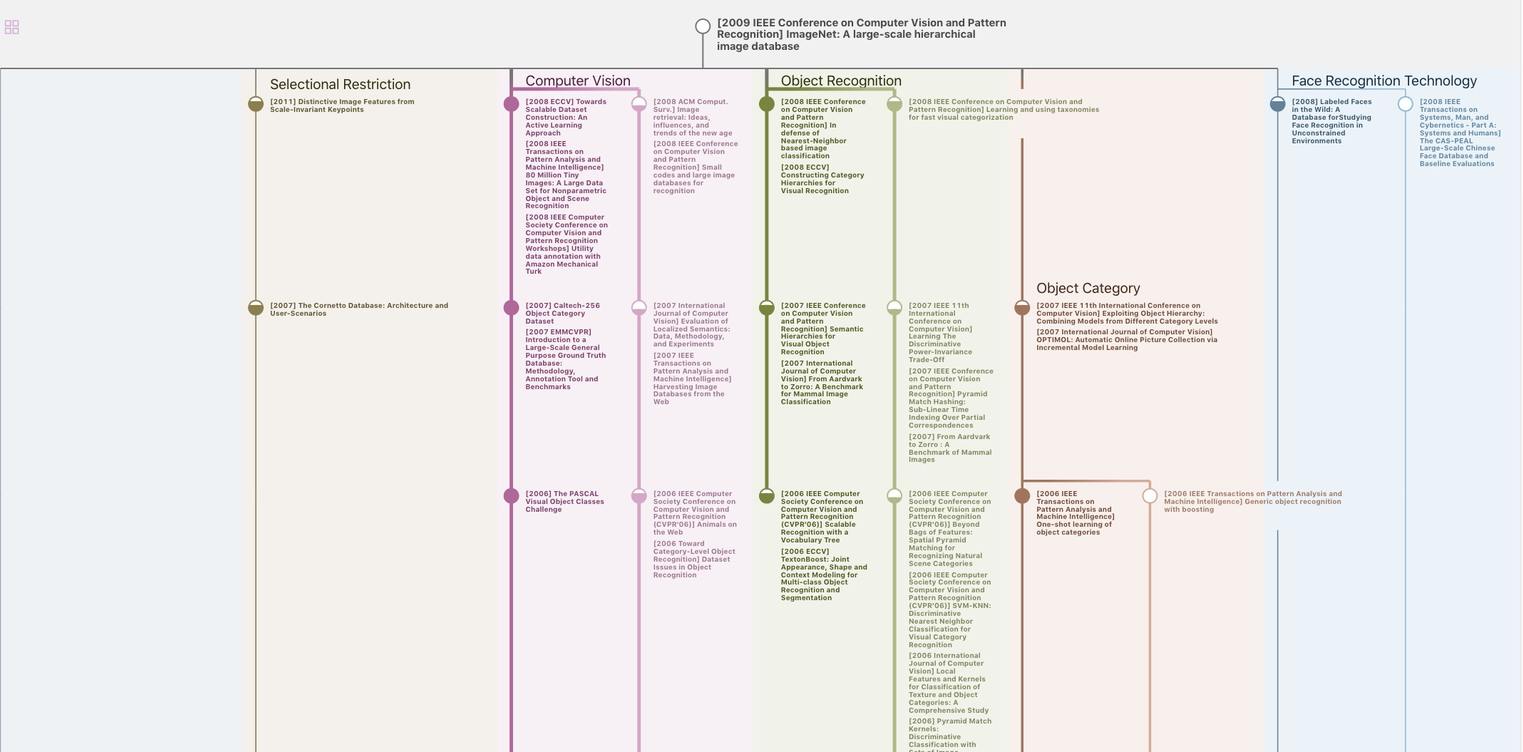
Generate MRT to find the research sequence of this paper
Chat Paper
Summary is being generated by the instructions you defined