Optimal deep transfer learning driven computer-aided breast cancer classification using ultrasound images
EXPERT SYSTEMS(2024)
摘要
Breast cancer (BC) is regarded as the second leading type of cancer among women globally. Ultrasound images are typically used for the identification and classification of abnormalities that exist in the breast. To enhance diagnosis performance, the computer assisted diagnosis (CAD) model finds it effective for identifying and classifying BC. Generally, the CAD technique contains distinct procedures like feature extraction, preprocessing, segmentation, and classification. The recent developments of deep learning (DL) algorithms in the form of CAD system helps to minimize the cost and enhance the ability of radiologists to interpret medical images. Therefore, this study develops an optimal deep transfer learning driven computer aided BC classification (ODTLD-CABCC) technique on ultrasound images. The presented ODTLD-CABCC algorithm undergoes pre-processing in two levels such as median filtering based noise removal and graph cut segmentation. Furthermore, the residual network (ResNet101) model can be used as a feature extractor. Finally, the sailfish optimizer (SFO) with a labelled weighted extreme learning machine (LWELM) algorithm is used for the classification process. The SFO technique is employed to choose optimal parameters involved in the LWELM algorithm. A comprehensive set of simulations are conducted on the benchmark data and the experimental outcomes are examined under numerous aspects. The comparative examination represents the supremacy of the ODTLD-CABCC technique over the other approaches.
更多查看译文
关键词
breast cancer,computer-aided diagnosis,deep learning,image classification,machine learning,ultrasound images
AI 理解论文
溯源树
样例
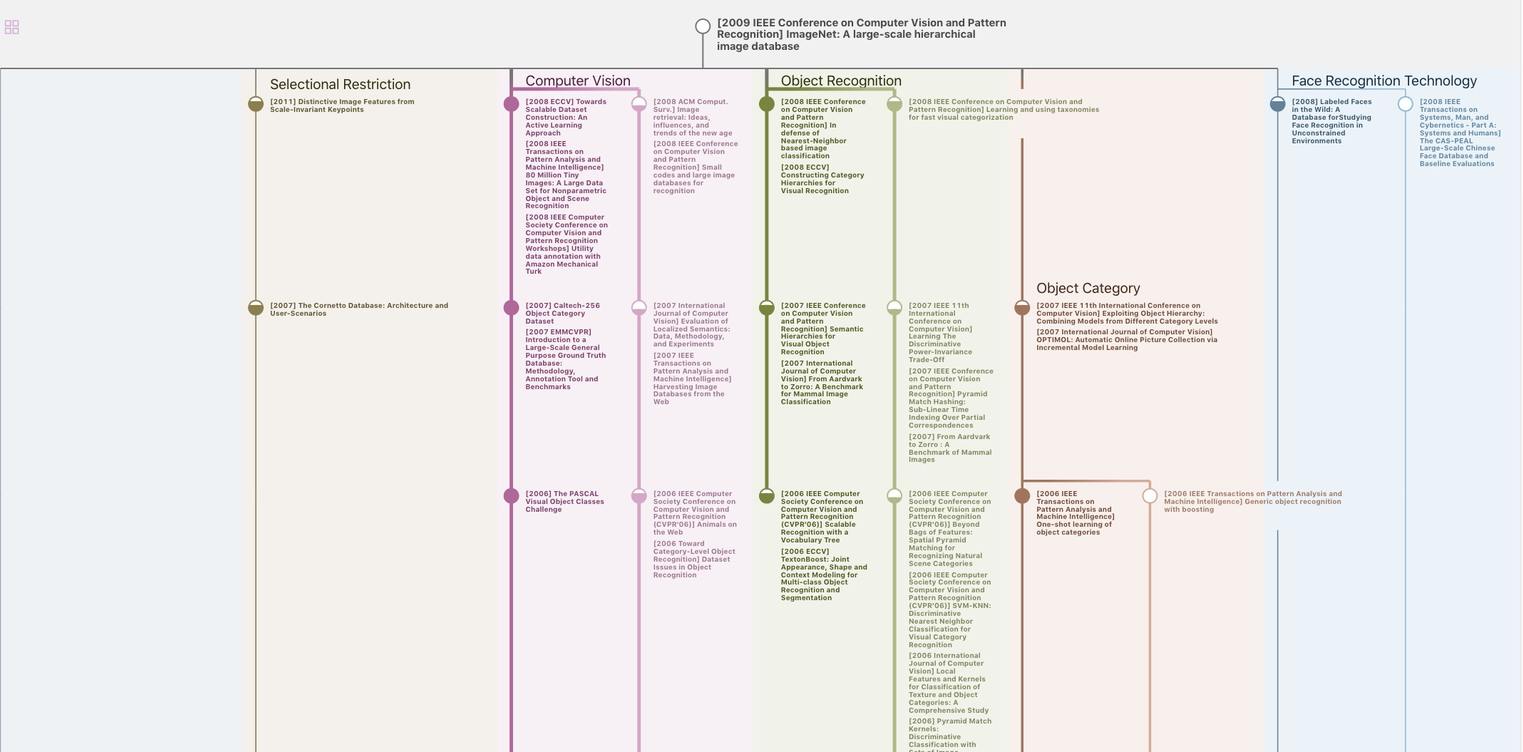
生成溯源树,研究论文发展脉络
Chat Paper
正在生成论文摘要