Fault diagnosis method of migration learning based on antagonism generation network
INTERNATIONAL JOURNAL OF INNOVATIVE COMPUTING INFORMATION AND CONTROL(2023)
摘要
Intelligence and automation technology are key to promoting the development of modern industrial manufacturing; therefore, ensuring the safe and effective operation of motors is an important research topic. Introducing deep learning technology into the fault diagnosis environment of motor systems, considering the nonlinear characteristics of industrial mechanical system signals, it is difficult to effectively obtain mechanical data signals. Therefore, the transfer learning of the physical diagnosis model is studied, the traditional confrontation generation network is optimized, and the collected real features are integrated into the physical model to build the mechanical fault diagnosis model of the boundary balanced confrontation generation network (Boundary Equilibrium GAN, BEGAN). At the same time, because the BEGAN model is faced with the problem of declining correlation of generated data, transfer learning is introduced to optimize the fault diagnosis model. Input the collected common motor fault data into the fault diagnosis model for training, improve the model's fault diagnosis accuracy, and thus achieve effective diagnosis of motor faults. In the test of motor bearing simulation spectrum diagram, the motor health, outer ring fault and inner ring fault meet the requirements of simulation vibration signal sequence, and the amplitude is A(0) > A(i) > A(H). The typical KNN and K-means algorithm are selected for comparison with the proposed FD model. In the FD of outer circle, the correct rate of FD of the proposed method is 0.923, which is higher than 0.815 and 0.885 of KNN and K-means. The diagnosis effect of the Fault Detection (FD) model is excellent and meets the needs of mechanical FD. The innovative application of machine learning technology in the field of motor faults through diagnostic model training and recognition of motor health status has important reference value for improving the effectiveness of industrial machinery fault diagnosis.
更多查看译文
关键词
BEGAN,Transfer learning,Motor fault,Diagnosis,Test
AI 理解论文
溯源树
样例
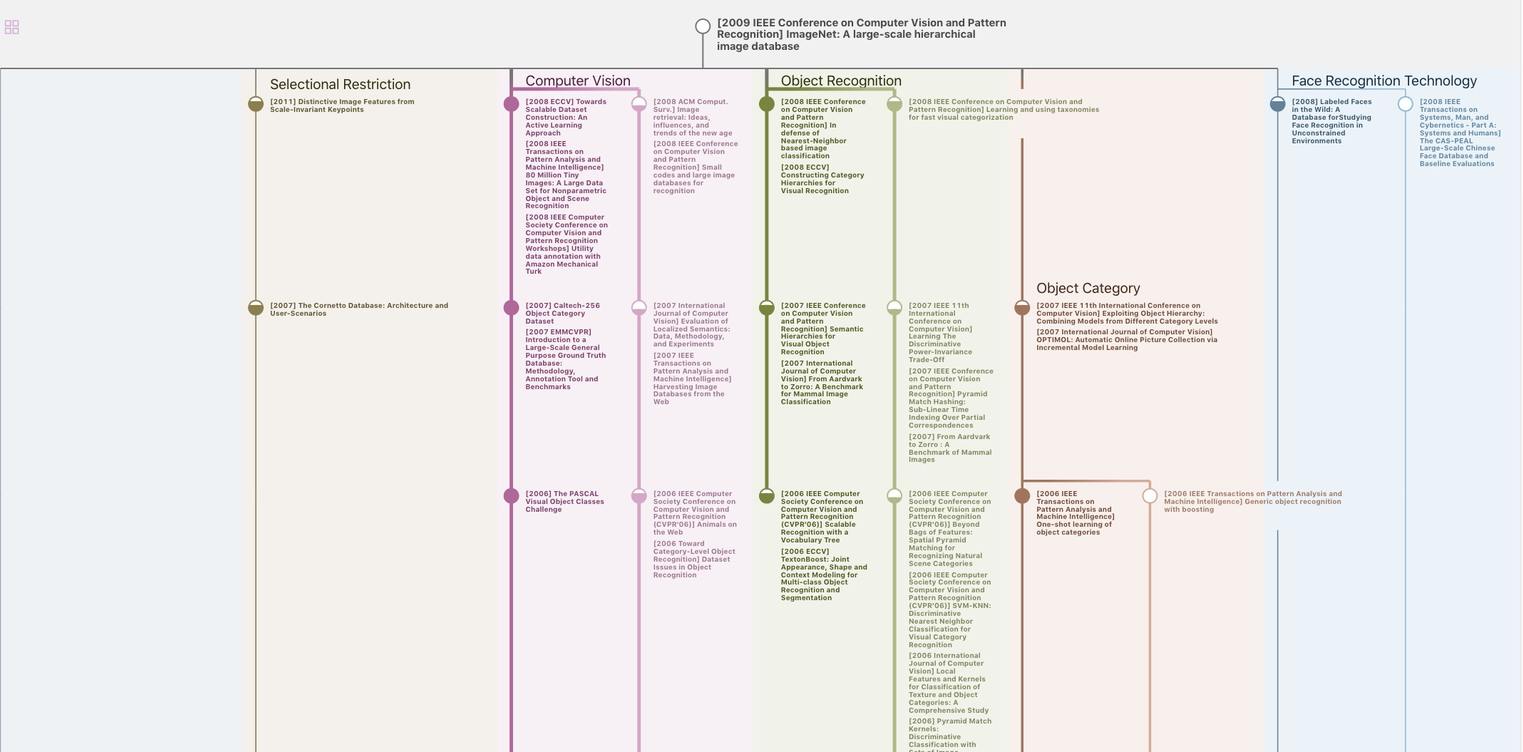
生成溯源树,研究论文发展脉络
Chat Paper
正在生成论文摘要