Machine Learning-Supported Enzyme Engineering toward Improved CO2-Fixation of Glycolyl-CoA Carboxylase
ACS SYNTHETIC BIOLOGY(2023)
摘要
Glycolyl-CoA carboxylase (GCC) is a new-to-nature enzyme that catalyzes the key reaction in the tartronyl-CoA (TaCo) pathway, a synthetic photorespiration bypass that was recently designed to improve photosynthetic CO2 fixation. GCC was created from propionyl-CoA carboxylase (PCC) through five mutations. However, despite reaching activities of naturally evolved biotin-dependent carboxylases, the quintuple substitution variant GCC M5 still lags behind 4-fold in catalytic efficiency compared to its template PCC and suffers from futile ATP hydrolysis during CO2 fixation. To further improve upon GCC M5, we developed a machine learning-supported workflow that reduces screening efforts for identifying improved enzymes. Using this workflow, we present two novel GCC variants with 2-fold increased carboxylation rate and 60% reduced energy demand, respectively, which are able to address kinetic and thermodynamic limitations of the TaCo pathway. Our work highlights the potential of combining machine learning and directed evolution strategies to reduce screening efforts in enzyme engineering.
更多查看译文
关键词
photorespiration,CO2 fixation,machine learning,directed evolution,enzyme engineering,glycolyl-CoA carboxylase
AI 理解论文
溯源树
样例
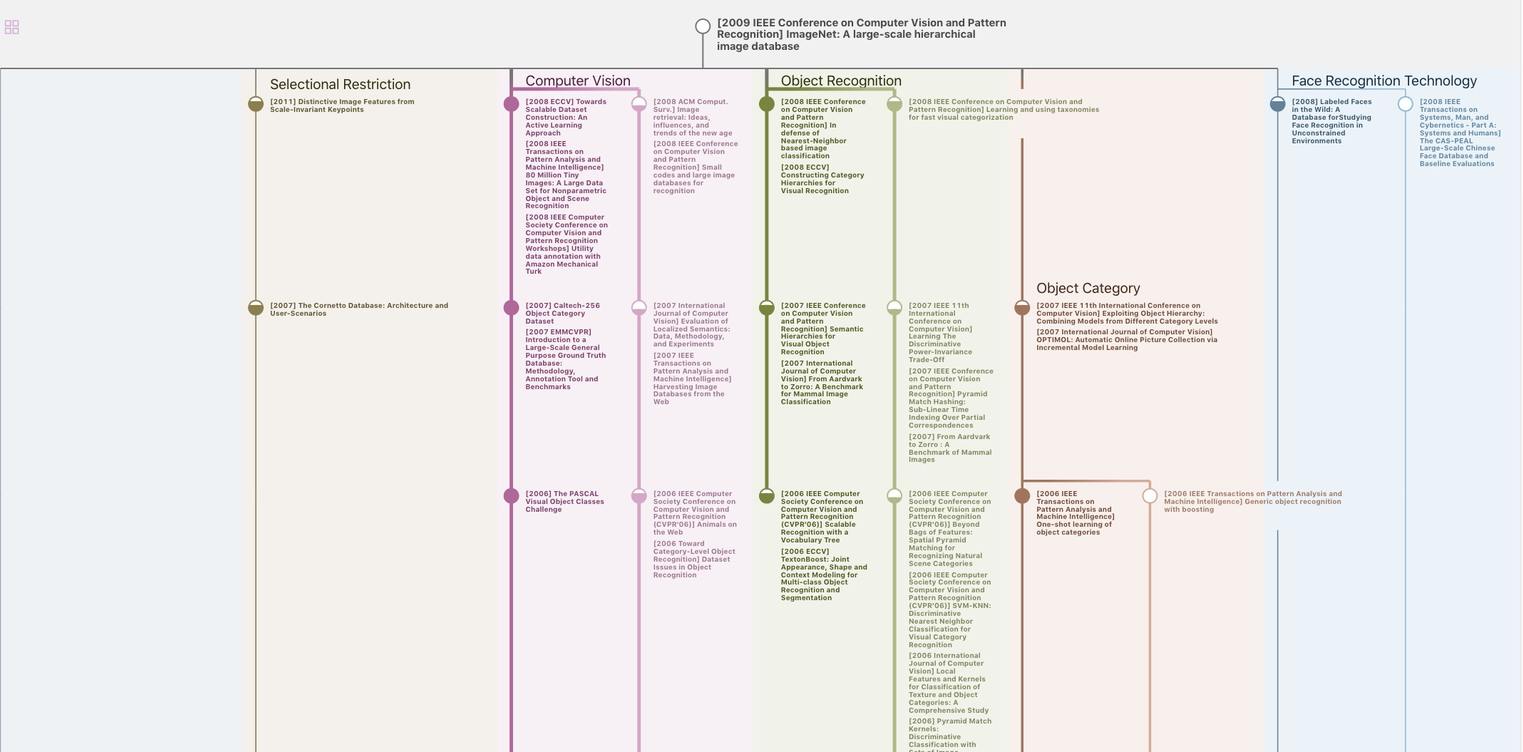
生成溯源树,研究论文发展脉络
Chat Paper
正在生成论文摘要