M-DDC: MRI based demyelinative diseases classification with U-Net and convolutional network
NEURAL NETWORKS(2024)
摘要
Childhood demyelinative diseases classification (DDC) with brain magnetic resonance imaging (MRI) is crucial to clinical diagnosis. But few attentions have been paid to DDC in the past. How to accurately differentiate pediatric-onset neuromyelitis optica spectrum disorder (NMOSD) from acute disseminated encephalomyelitis (ADEM) based on MRI is challenging in DDC. In this paper, a novel architecture M-DDC based on joint U-Net segmentation network and deep convolutional network is developed. The U-Net segmentation can provide pixel-level structure information, that helps the lesion areas location and size estimation. The classification branch in DDC can detect the regions of interest inside MRIs, including the white matter regions where lesions appear. The performance of the proposed method is evaluated on MRIs of 201 subjects recorded from the Children's Hospital of Zhejiang University School of Medicine. The comparisons show that the proposed DDC achieves the highest accuracy of 99.19% and dice of 71.1% for ADEM and NMOSD classification and segmentation, respectively.
更多查看译文
关键词
Pediatric demyelinating disease,Magnetic resonance imaging,Image classification,Image segmentation,Deep learning,U-Net
AI 理解论文
溯源树
样例
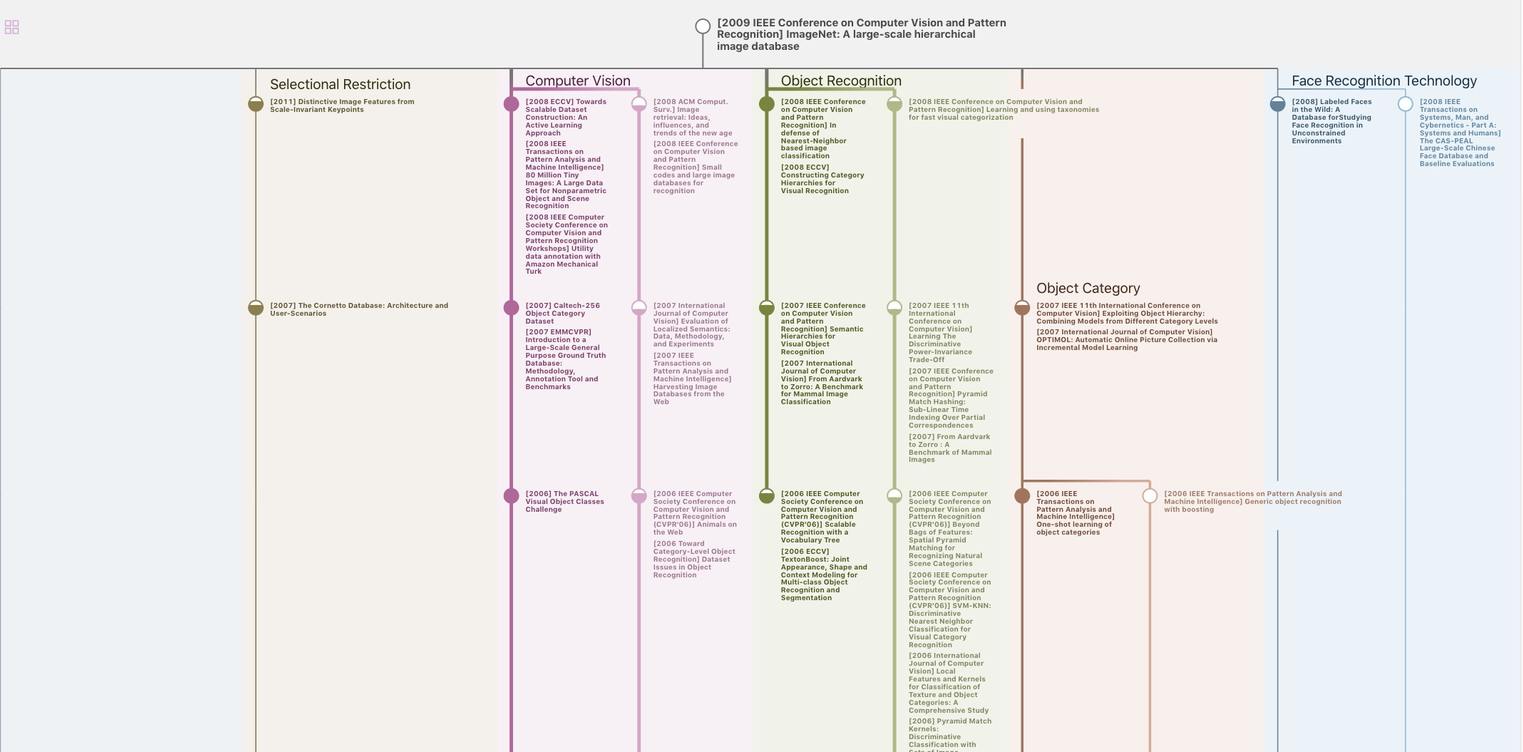
生成溯源树,研究论文发展脉络
Chat Paper
正在生成论文摘要