Machine Learning-Based Prediction of Immunomodulatory Properties of Polymers: Toward a Faster and Easier Development of Anti-Inflammatory Biomaterials
ADVANCED NANOBIOMED RESEARCH(2024)
摘要
In biomaterials development, creating materials with desirable properties can be a time-consuming and resource-intensive process, often relying on serendipitous discoveries. A potential route to accelerate this process is to employ artificial intelligence methodologies such as machine learning (ML). Herein, the possibility to predict anti-inflammatory properties of the polymers by using a simplified model of inflammation and a restrained dataset is explored. Cellular assays with 50 different polymers are conducted using the murine macrophage cell line RAW 264.7 as a model. These experiments generate a dataset which is used to develop a ML model based on Bayesian logistic regression. After conducting a Bayesian logistic regression analysis, two ML models, K-nearest neighbors (KNN) and Naive Bayes, are employed to predict anti-inflammatory polymers properties. The study finds that the probability of a polymer having anti-inflammatory properties is multiplied by three if it is a polycation, and that nitric oxide secretion is a good indicator in determining the anti-inflammatory properties of a polymer, which in this work are defined by tumor necrosis factor alpha expression decrease. Overall, the study suggests that with appropriate dataset design, ML techniques can provide valuable information on functional polymer properties, enabling faster and more efficient biomaterial development. Polymeric materials are widely used for biomedical applications, from implants to thin nanoscale coatings. Herein, the possibility to predict anti-inflammatory properties of the polymers using machine learning algorithms is explored. The study suggests that with appropriate dataset design, machine learning techniques can provide valuable information on functional polymer properties, enabling faster and more efficient biomaterial development.image (c) 2024 WILEY-VCH GmbH
更多查看译文
关键词
Bayesian logistic regression,in silico,inflammation,machine learning,polymers,predictive models
AI 理解论文
溯源树
样例
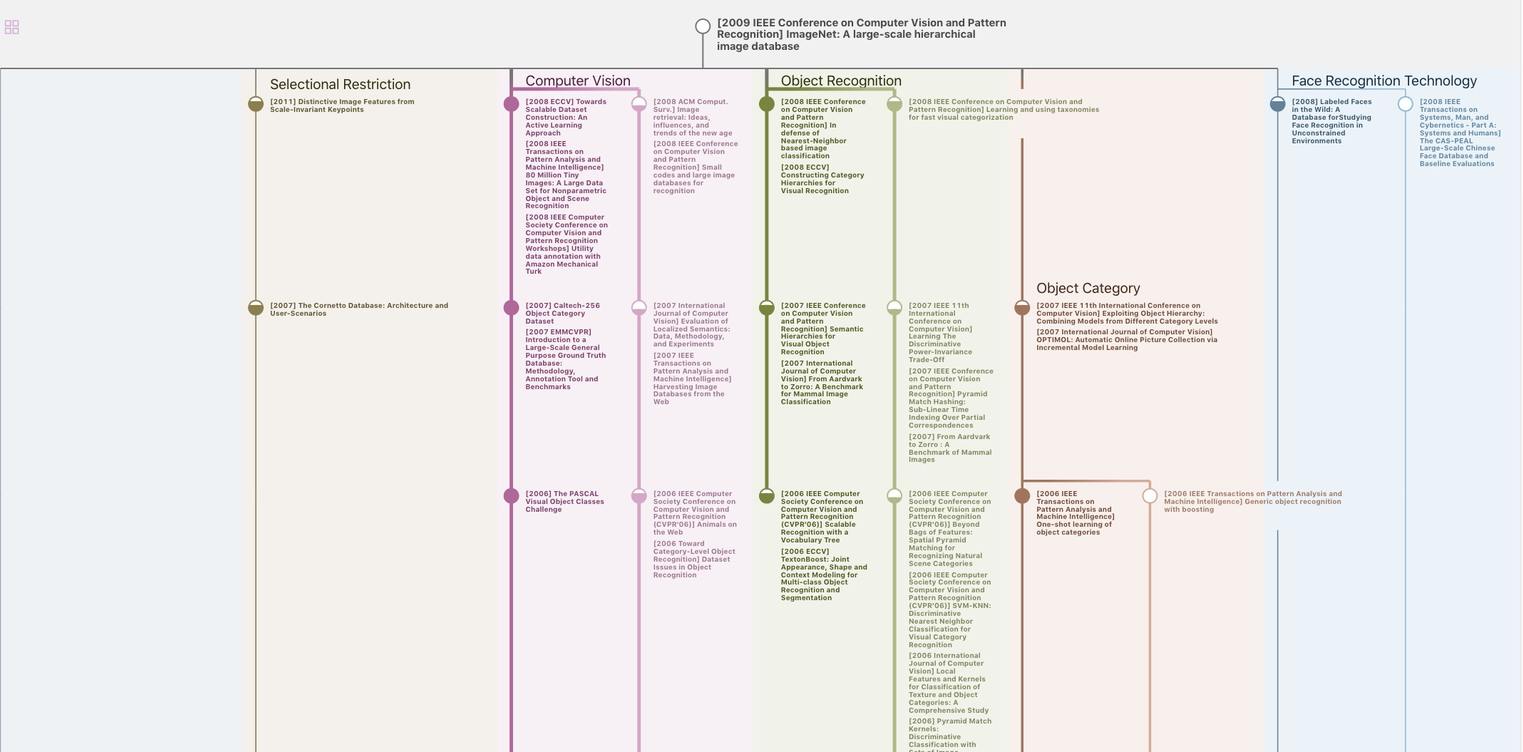
生成溯源树,研究论文发展脉络
Chat Paper
正在生成论文摘要