Utilizing autoencoders for analysis and classification of microscopic in situ hybridization images
COMPTES RENDUS DE L ACADEMIE BULGARE DES SCIENCES(2023)
Abstract
Currently, analysis of microscopic In Situ Hybridization (ISH) images is done manually by experts. Precise evaluation and classification of such micro-scopic images can ease experts' work and reveal further insights about the data. In this work, we propose a deep-learning workflow to detect and classify areas of microscopic images with similar levels of gene expression. Analysis of the data is done by employing a type of ANN - Deep Learning Autoencoders - suitable for unsupervised learning. The model's performance is optimised by balancing the latent layers' length and complexity and fine-tuning hyperparameters. The results are validated by adapting the mean-squared error (MSE) metric and comparison to expert's evaluation. Reconstruction of the whole-scale micro-scopic images is used to summarise and visualise the results.
MoreTranslated text
Key words
artificial neural networks,deep learning autoencoders,image analysis,unsupervised learning,fuzzy clustering
AI Read Science
Must-Reading Tree
Example
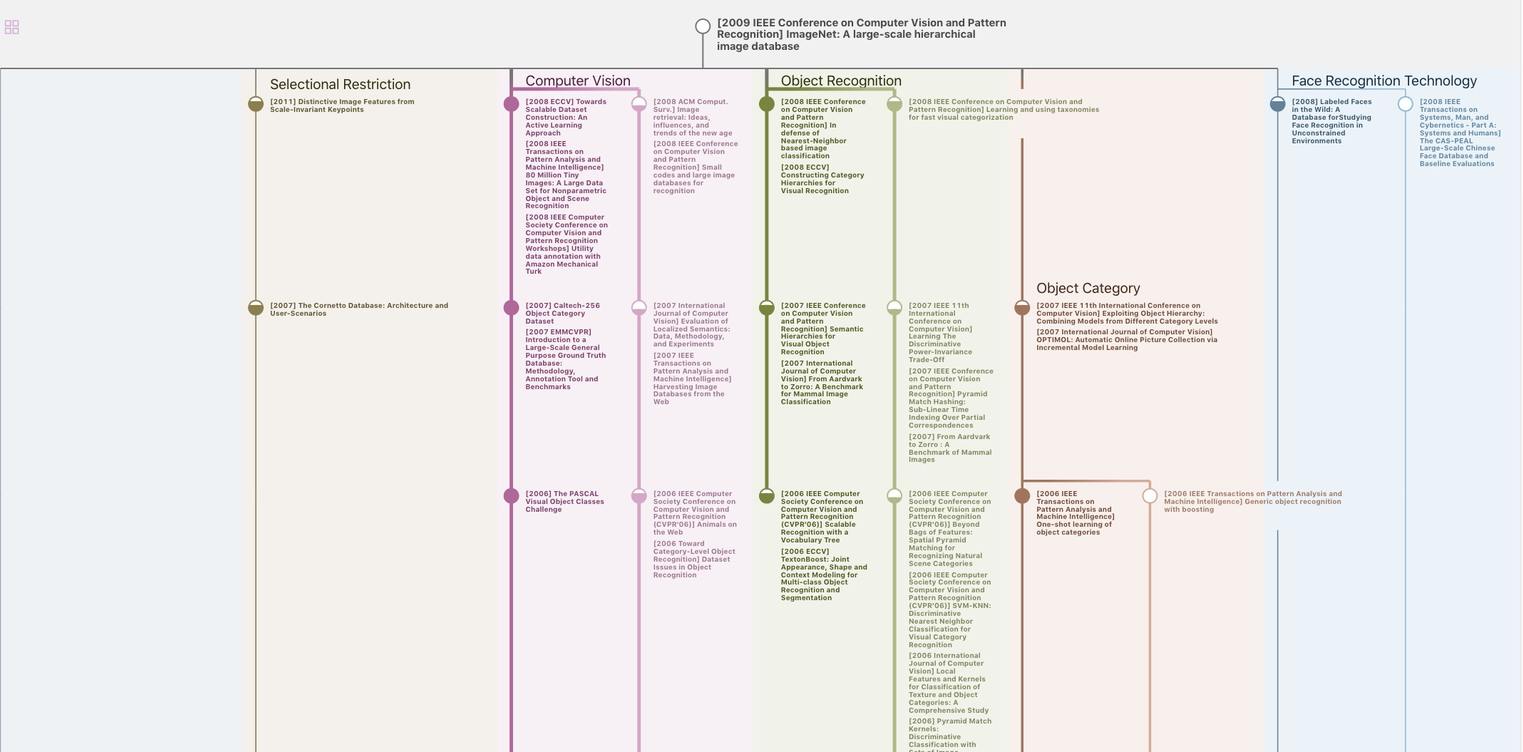
Generate MRT to find the research sequence of this paper
Chat Paper
Summary is being generated by the instructions you defined