Agricultural Price Forecasting Based on Variational Mode Decomposition and Time-Delay Neural Network
STATISTICS AND APPLICATIONS(2023)
Abstract
Agricultural commodities prices are very unpredictable and complex, and thus, forecasting these prices is one of the research hotspots. In this paper, we propose a new hybrid VMD-TDNN model combining variational mode decomposition (VMD) and time-delay neural network (TDNN) to improve the accuracy of agricultural price forecasting. Specifically, the VMD decomposes a price series into a set of intrinsic mode functions (IMFs), and the obtained IMFs are modelled and forecasted separately using the TDNN models. Finally, the forecasts of all IMFs are combined to provide an ensemble output for the price series. VMD overcomes the limitation of the mode mixing and end effect problems of the empirical mode decomposition (EMD) based variants. The prediction ability of the proposed model is compared with TDNN, and EMD based variants coupled with TDNN model using international monthly price series of maize, palm oil, and soybean in terms of evaluation criteria like root mean squared error, mean absolute percentage error and, directional prediction statistics. Additionally, Diebold-Mariano test and Technique for Order of Preference by Similarity to Ideal Solution (TOPSIS), a ranking system, are used to evaluate the accuracy of the models. The empirical results confirm that the proposed hybrid model is superior in terms of evaluation criteria and improves the prediction accuracy significantly.
MoreTranslated text
Key words
Agricultural price forecasting,Empirical mode decomposition,Intrinsic mode,function,Time-delay neural network,Variational mode decomposition.
AI Read Science
Must-Reading Tree
Example
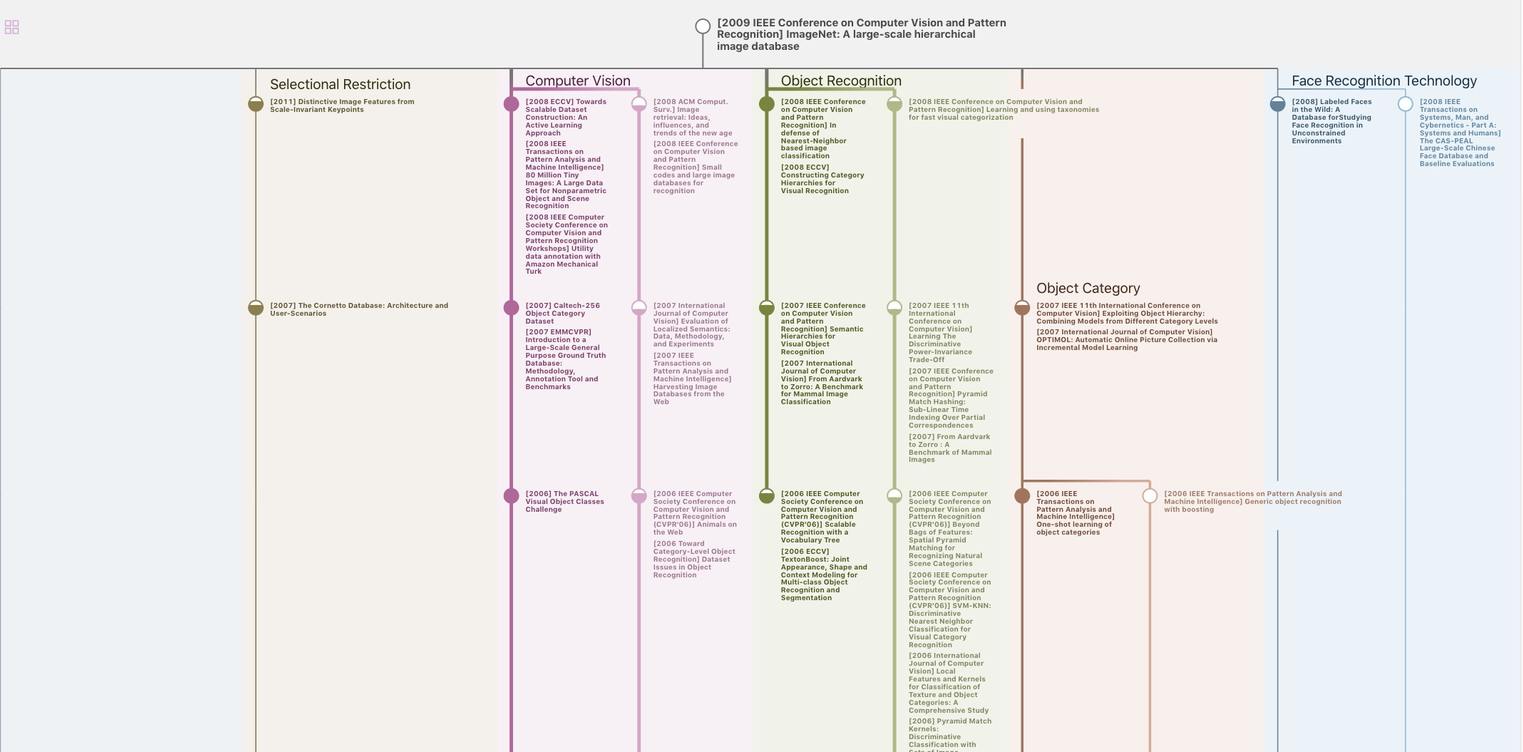
Generate MRT to find the research sequence of this paper
Chat Paper
Summary is being generated by the instructions you defined