Enhancing model predictions through the fusion of stein estimator and principal component regression
JOURNAL OF STATISTICAL COMPUTATION AND SIMULATION(2024)
摘要
This research aims to enhance model predictions by introducing a novel approach that combines the Stein estimator technique with principal component regression (PCR) within the linear regression context. The study recognizes the challenges posed by multicollinearity in predictive modelling and addresses them by leveraging the strengths of the Stein estimator and PCR. We compared the novel estimator with other commonly used regression techniques to showcase its superiority theoretically. we investigate the impact of different parameter settings and sample sizes on the performance of the enhanced model predictions via simulation design. We analyzed two real-life data to validate the theoretical and simulation results. The mean squared error was employed to measure the performance gain achieved by the fusion of these techniques. The results show the benefits of combining the Stein estimator and PCR. It also provides valuable insights into advanced techniques for enhancing model predictions.
更多查看译文
关键词
Biased estimators,linear regression,multicollinearity,principal component regression,stein estimator
AI 理解论文
溯源树
样例
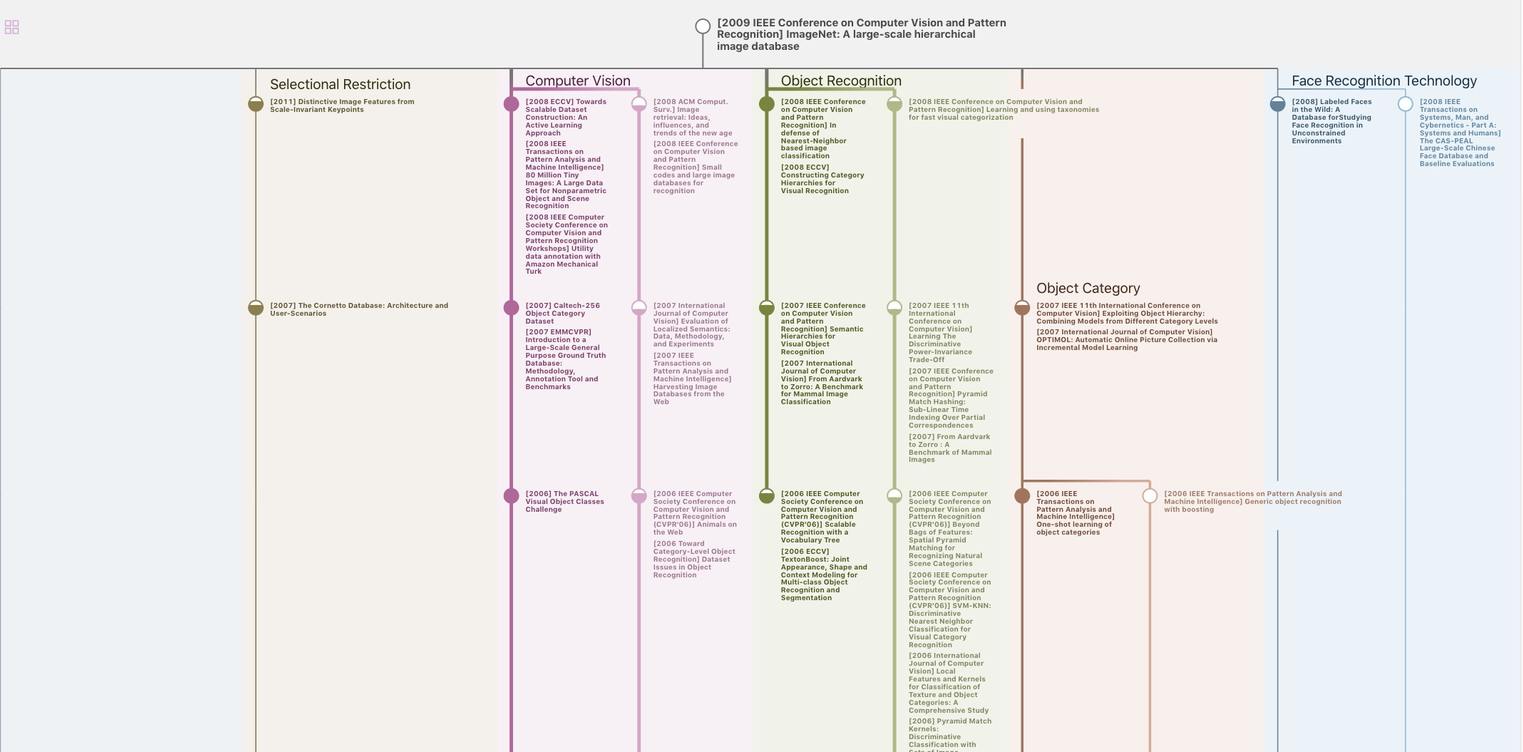
生成溯源树,研究论文发展脉络
Chat Paper
正在生成论文摘要