Federated learning on non-IID and globally long-tailed data via meta re-weighting networks
INTERNATIONAL JOURNAL OF WAVELETS MULTIRESOLUTION AND INFORMATION PROCESSING(2024)
摘要
Under a federated learning environment, the training samples are generally collected and stored locally on each client's device, which makes the machine learning procedure not meet the requirement of independent and identical distribution (IID). Existing federated learning methods to deal with non-IID data generally assume that the data is globally balanced. However, real-world multi-class data tend to exhibit long-tail distribution, where the majority of samples are in a few head classes and a large number of tail classes only have a small amount of data. This paper, therefore, focuses on addressing the problem of handling non-IID and globally long-tailed data in a federated learning scenario. Accordingly, we propose a new federated learning method called Federated meta re-weighting networks (FedReN), which assigns weights during the local training process from the class-level and instance-level perspectives, respectively. To deal with data non-IIDness and global long-tail, both of the two re-weighting functions are globally trained by the meta-learning approach to acquire the knowledge of global long-tail distribution. Experiments on several long-tailed image classification benchmarks show that FedReN outperforms the state-of-the-art federated learning methods. The code is available at https://github.com/pxqian/FedReN.
更多查看译文
关键词
Federated learning,long-tail learning,meta-learning,re-weighting
AI 理解论文
溯源树
样例
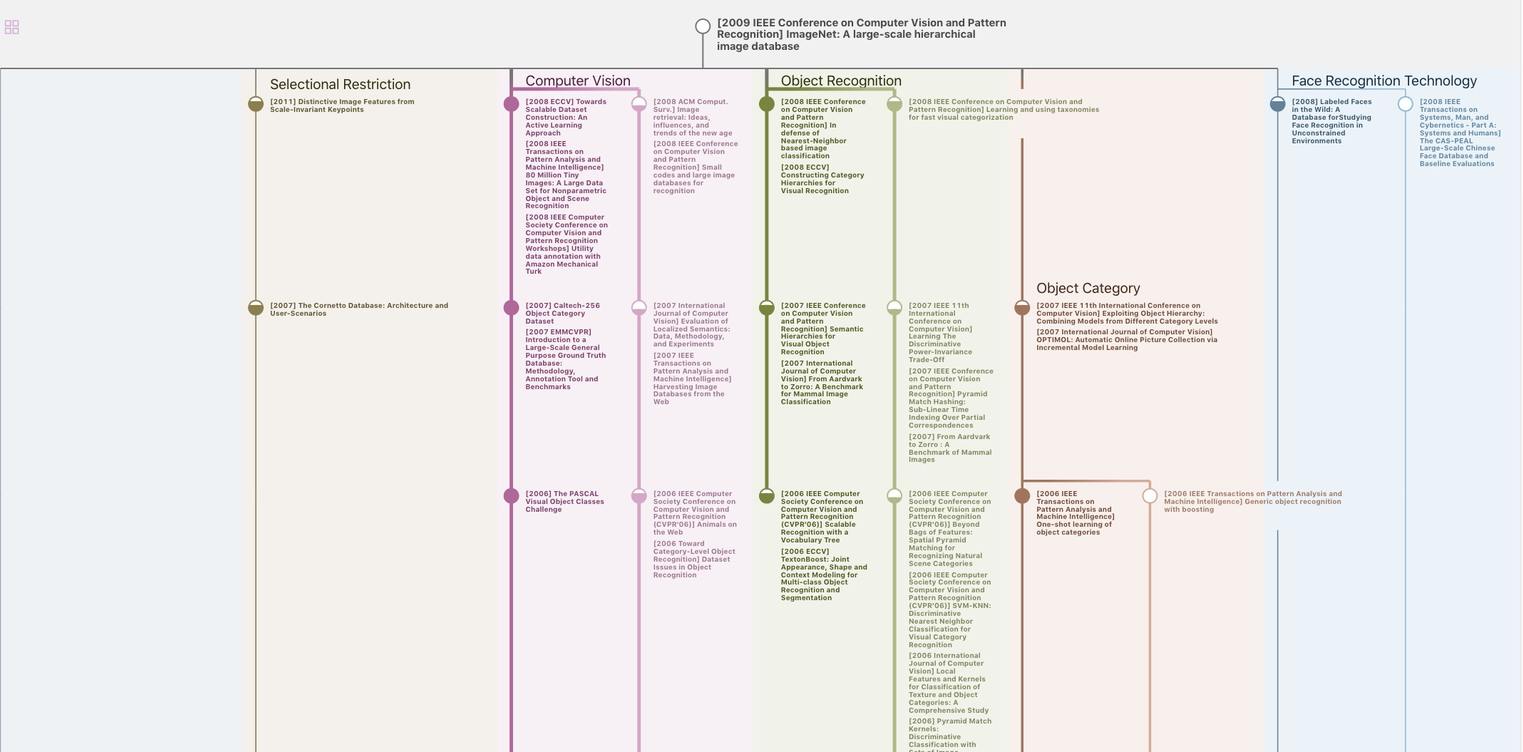
生成溯源树,研究论文发展脉络
Chat Paper
正在生成论文摘要