Deep Learning-Based Regional Ionospheric Total Electron Content Prediction-Long Short-Term Memory (LSTM) and Convolutional LSTM Approach
SPACE WEATHER-THE INTERNATIONAL JOURNAL OF RESEARCH AND APPLICATIONS(2024)
摘要
This study evaluates the performance of deep learning approach in the prediction of the ionospheric total electron content (TEC) during magnetically quiet periods. Two deep learning techniques, long short-term memory (LSTM) and convolutional LSTM (ConvLSTM), are employed to predict TEC values 24 hr ahead in the vicinity of the Korean Peninsula (26.5 degrees-40 degrees N, 121 degrees-134.5 degrees E). The LSTM method predicts TEC at a single point based on time series of data at that point, whereas the ConvLSTM method simultaneously predicts TEC values at multiple points using spatiotemporal distribution of TEC. Both the LSTM and ConvLSTM models are trained using the complete regional TEC maps reconstructed by applying the Deep Convolutional Generative Adversarial Network-Poisson Blending (DCGAN-PB) method to observed TEC data. The training period spans from 2002 to 2018, and the model performance is evaluated using 2019 data. Our results show that the ConvLSTM method outperforms the LSTM method, generating more reliable TEC maps with smaller root mean square errors when compared to the ground truth (DCGAN-PB TEC maps). This outcome indicates that deep learning models can improve the prediction accuracy of TEC at a specific point by taking into account spatial information of TEC. We conclude that ConvLSTM is a reliable and efficient approach for the prompt ionospheric prediction. The total electron content (TEC) from global navigation and satellite system (GNSS) serves as a crucial ionospheric parameter for studying the Earth's ionosphere and space weather. Given its significance, considerable efforts have been devoted to worldwide TEC data acquisition and future TEC prediction. Recent advancements in artificial intelligence, including neural network and deep learning, have been applied to generate global/regional TEC maps and to forecast TEC values. This study utilizes two deep learning techniques, namely long short-term memory (LSTM) and convolutional LSTM (ConvLSTM), to forecast TEC values 24 hr in advance within the vicinity of the Korean Peninsula. While previous studies have utilized the LSTM method for single-location TEC prediction, our study employs the ConvLSTM approach to simultaneously predict TECs across multiple points. Our results demonstrate the ConvLSTM method's superior performance over the LSTM method. This superiority is attributed to the ConvLSTM method's ability to use the spatiotemporal distribution of data in its prediction. We conclude that the ConvLSTM method is a reliable and efficient approach for accurately predicting future ionospheric conditions. Long short-term memory (LSTM) and convolutional LSTM methods are employed to predict total electron content (TEC) 24-hr advancePrediction accuracy at a specific point improves when LSTM models incorporate the data around the given pointHowever, convolutional LSTM method outperforms LSTM method, generating more reliable TEC maps with smaller Root mean square errors (RMSEs)
更多查看译文
AI 理解论文
溯源树
样例
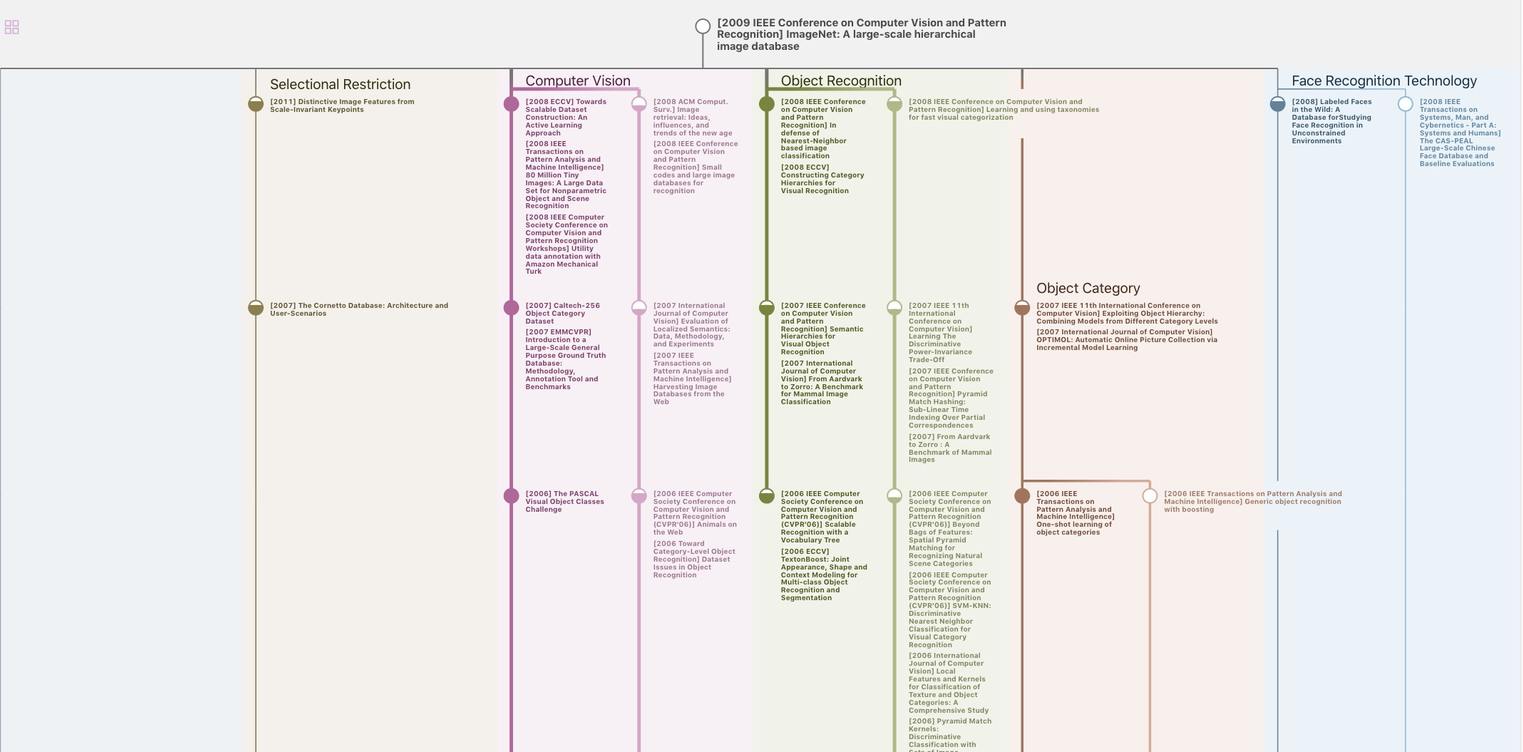
生成溯源树,研究论文发展脉络
Chat Paper
正在生成论文摘要