A Robust Framework for Multimodal Sentiment Analysis with Noisy Labels Generated from Distributed Data Annotation
CMES-COMPUTER MODELING IN ENGINEERING & SCIENCES(2024)
Abstract
Multimodal sentiment analysis utilizes multimodal data such as text, facial expressions and voice to detect people's attitudes. With the advent of distributed data collection and annotation, we can easily obtain and share such multimodal data. However, due to professional discrepancies among annotators and lax quality control, noisy labels might be introduced. Recent research suggests that deep neural networks (DNNs) will overfit noisy labels, leading to the poor performance of the DNNs. To address this challenging problem, we present a Multimodal Robust Meta Learning framework (MRML) for multimodal sentiment analysis to resist noisy labels and correlate distinct modalities simultaneously. Specifically, we propose a two-layer fusion net to deeply fuse different modalities and improve the quality of the multimodal data features for label correction and network training. Besides, a multiple meta-learner (label corrector) strategy is proposed to enhance the label correction approach and prevent models from overfitting to noisy labels. We conducted experiments on three popular multimodal datasets to verify the superiority of our method by comparing it with four baselines.
MoreTranslated text
Key words
Distributed data collection,multimodal sentiment analysis,meta learning,learn with noisy labels
AI Read Science
Must-Reading Tree
Example
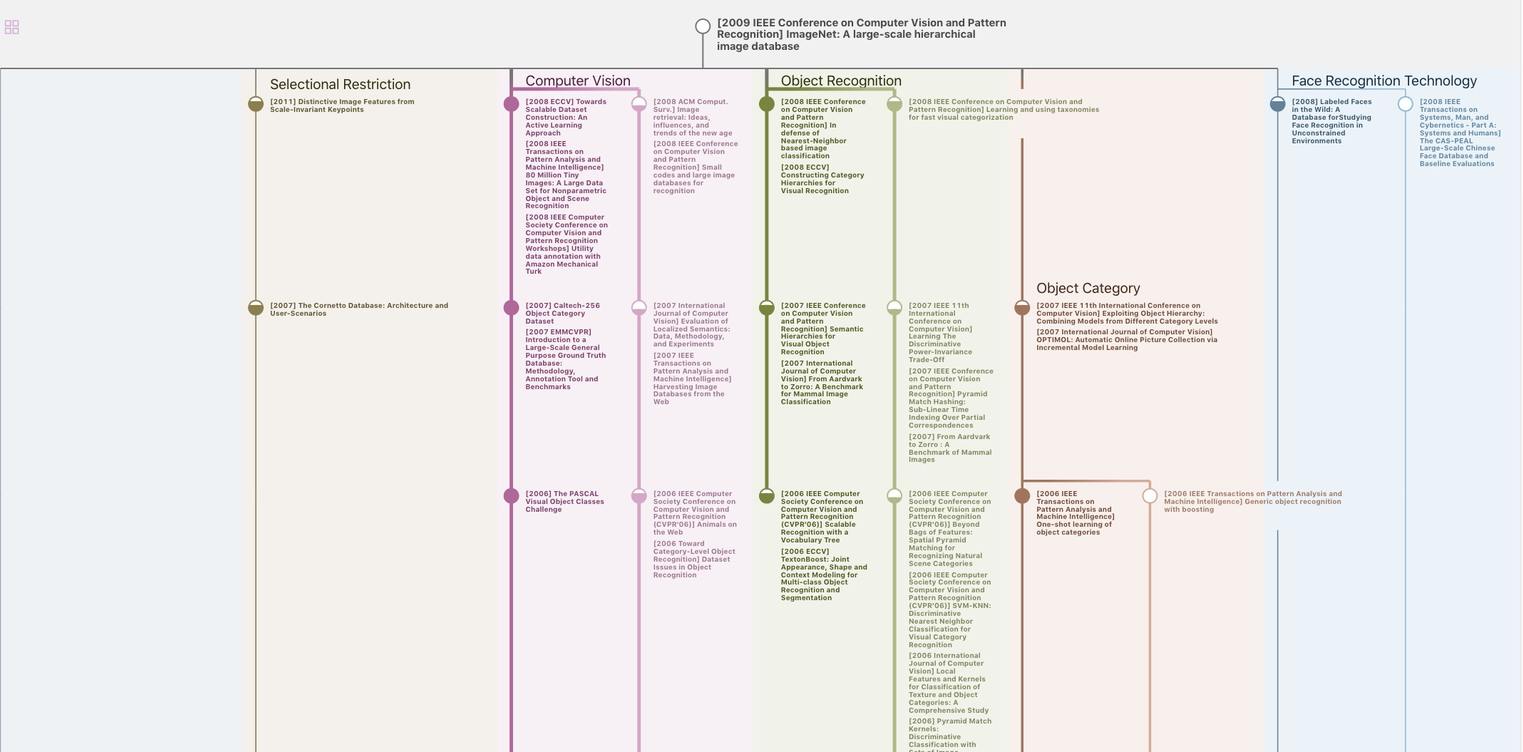
Generate MRT to find the research sequence of this paper
Chat Paper
Summary is being generated by the instructions you defined