Revealing microcanonical phases and phase transitions of strongly correlated systems via time-averaged classical shadows
PHYSICAL REVIEW B(2023)
摘要
Quantum computers and simulators promise to enable the study of strongly correlated quantum systems. Yet, surprisingly, it is hard for them to compute ground states. They can, however, efficiently compute the dynamics of closed quantum systems. We propose a method to study the quantum thermodynamics of strongly correlated electrons from quantum dynamics. We define time-averaged classical shadows (TACS) and prove it is a classical shadow(CS) of the von Neumann ensemble, the time-averaged density matrix. We then show that the diffusion maps, an unsupervised machine learning algorithm, can efficiently learn the phase diagram and phase transition of the one-dimensional transverse field Ising model both for ground states using CS and state trajectories using TACS. It does so from state trajectories by learning features that appear to be susceptibility and entropy from a total of 90 000 shots taken along a path in the microcanonical phase diagram. Our results suggest a low number of shots from quantum simulators can produce quantum thermodynamic data with a quantum advantage.
更多查看译文
AI 理解论文
溯源树
样例
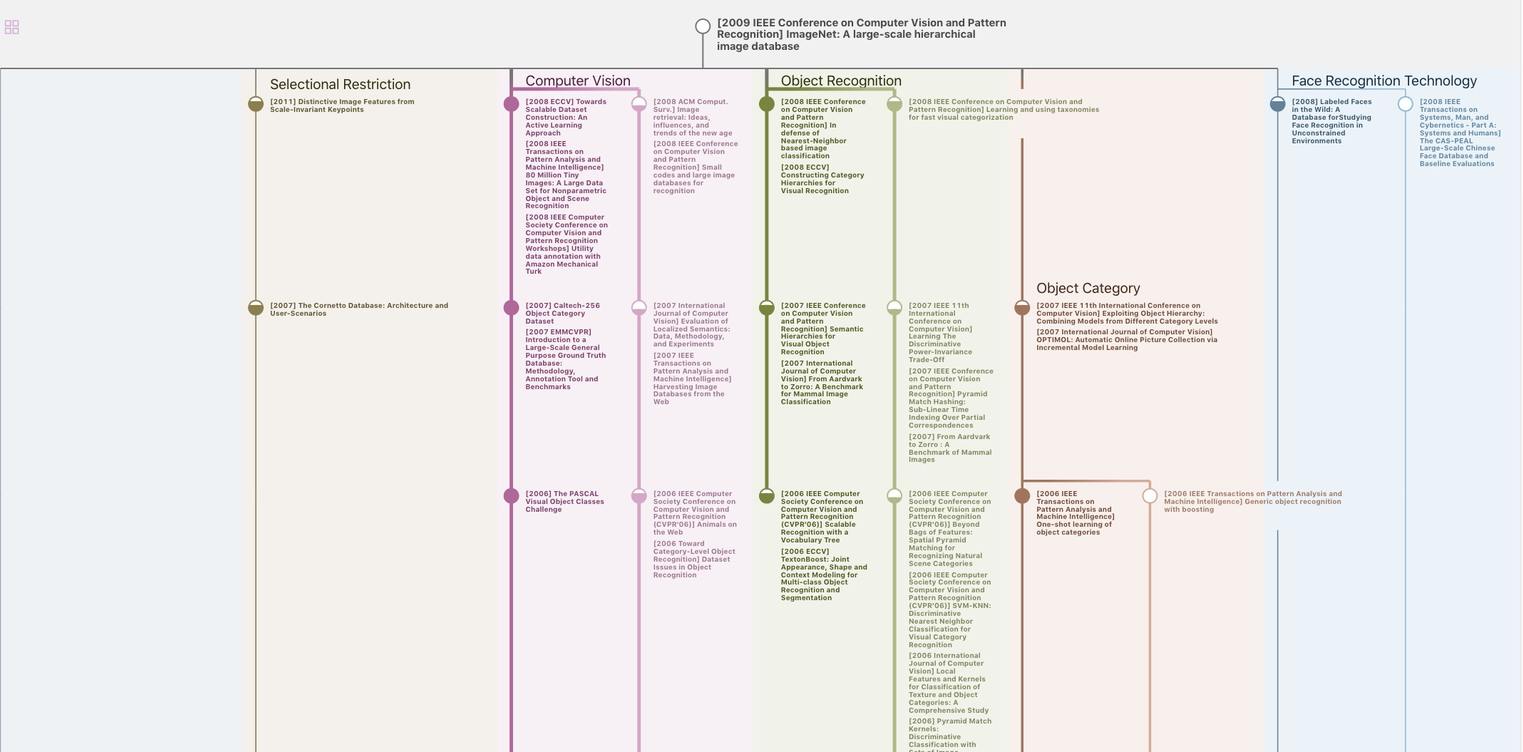
生成溯源树,研究论文发展脉络
Chat Paper
正在生成论文摘要