Personalized federated reinforcement learning: Balancing personalization and via distance constraint
EXPERT SYSTEMS WITH APPLICATIONS(2024)
摘要
Traditional federated reinforcement learning methods aim to find an optimal global policy for all agents. However, due to the heterogeneity of the environment, the optimal global policy is often only a suboptimal solution. To resolve this problem, we propose a personalized federated reinforcement learning method, named perFedDC, which aims to establish an optimal personalized policy for each agent. Our method involves creating a global model and multiple local models, using the I2-norm to measure the distance between the global model and the local model. We introduce a distance constraint as a regularization term in the update of the local model to prevent excessive policy updates. While the distance constraint can facilitate experience sharing, it is important to strike a balance between personalization and sharing appropriately. As much as possible, agents benefit from the advantages of shared experience while developing personalization. The experiments demonstrated that perFedDC was able to accelerate agent training in a stable manner while still maintaining the privacy constraints of federated learning. Furthermore, newly added agents to the federated system were able to quickly develop effective policies with the aid of convergent global policies.
更多查看译文
关键词
Reinforcement learning,Federated learning,Personalization,Regularization,Distance constraint,Experience sharing
AI 理解论文
溯源树
样例
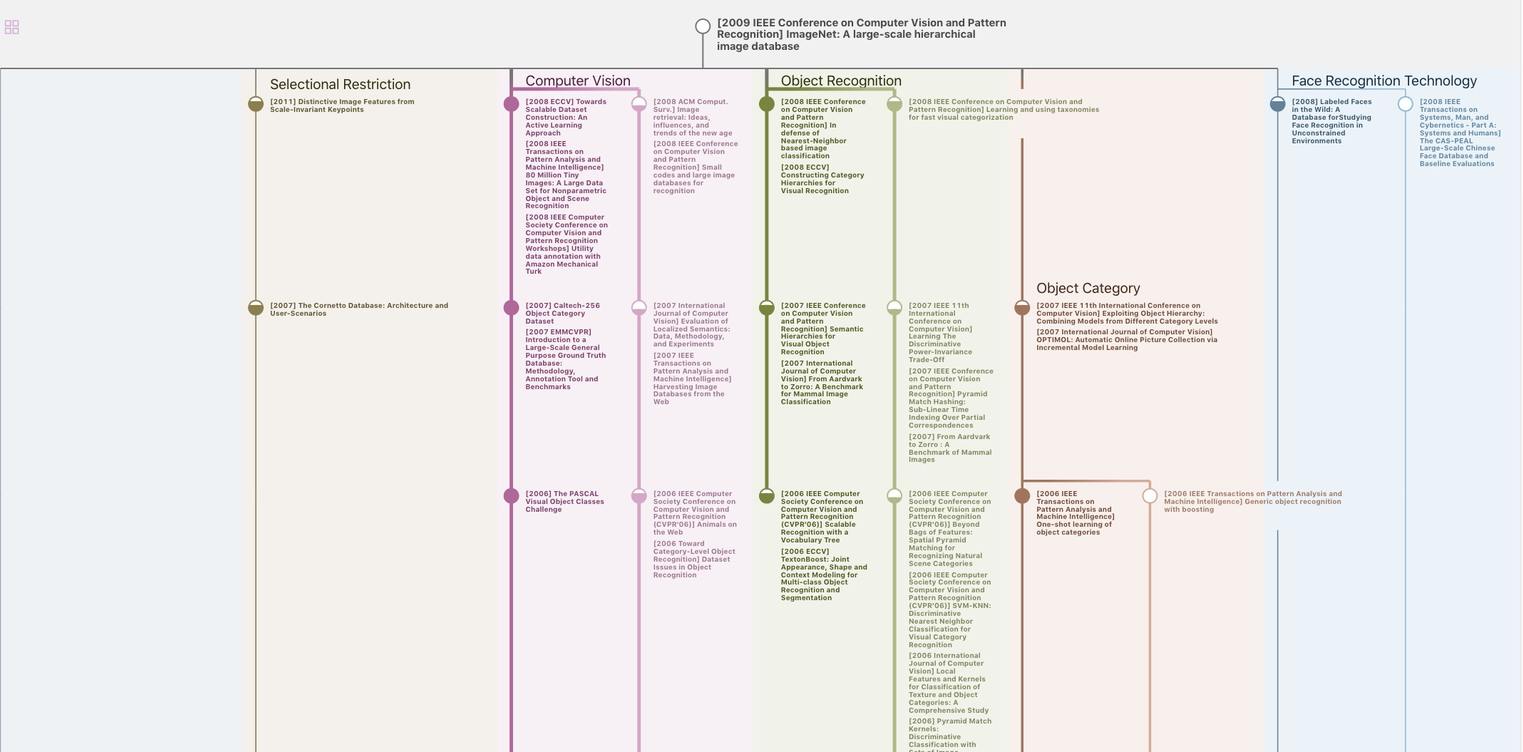
生成溯源树,研究论文发展脉络
Chat Paper
正在生成论文摘要