Study on dynamic prediction method for degradation state of electric drive system based on deep learning and uncertainty quantification
PROCEEDINGS OF THE INSTITUTION OF MECHANICAL ENGINEERS PART D-JOURNAL OF AUTOMOBILE ENGINEERING(2023)
Abstract
Accurate prediction of degradation state (DS) in electric drive system (EDS) under time-varying conditions is of great importance for its reliability assessment and health monitoring. To address the complex degradation trajectories and the difficulty of dynamically evaluating the degradation state of EDS under user conditions, this paper proposes a dynamic prediction method for the DS of multiple components in EDS based on deep learning and uncertainty quantification. The method utilizes actual operating data spanning 350,000 km and constructs operating condition characteristics by combining physical models of component failure. A deep clustering algorithm is proposed to achieve clustering of operating conditions with similar degradation rates. Based on the resulting six clusters, linear degradation models for different components are established. The 42-dimensional features characterizing the operating conditions are constructed from time domain, frequency domain and load counting perspectives. An integrated approach combining autoencoders, multi-layer convolutional neural networks, and weight optimization algorithms is utilized to achieve accurate recognition of operating conditions, resulting in an average recognition accuracy of 94.5%, outperforming traditional methods. Considering the uncertainty in degradation rate and the errors in operating condition recognition, dynamic prediction of DS under varying operating conditions is achieved. The effectiveness of the prediction results is verified through constructed performance metrics such as the coefficient of determination, root mean square error, and average reproducibility ratio. The proposed method provides a theoretical basis and technical support for the dynamic assessment of system reliability.
MoreTranslated text
Key words
Electric drive system,degradation state prediction,deep learning,uncertainty quantification,time-varying condition
AI Read Science
Must-Reading Tree
Example
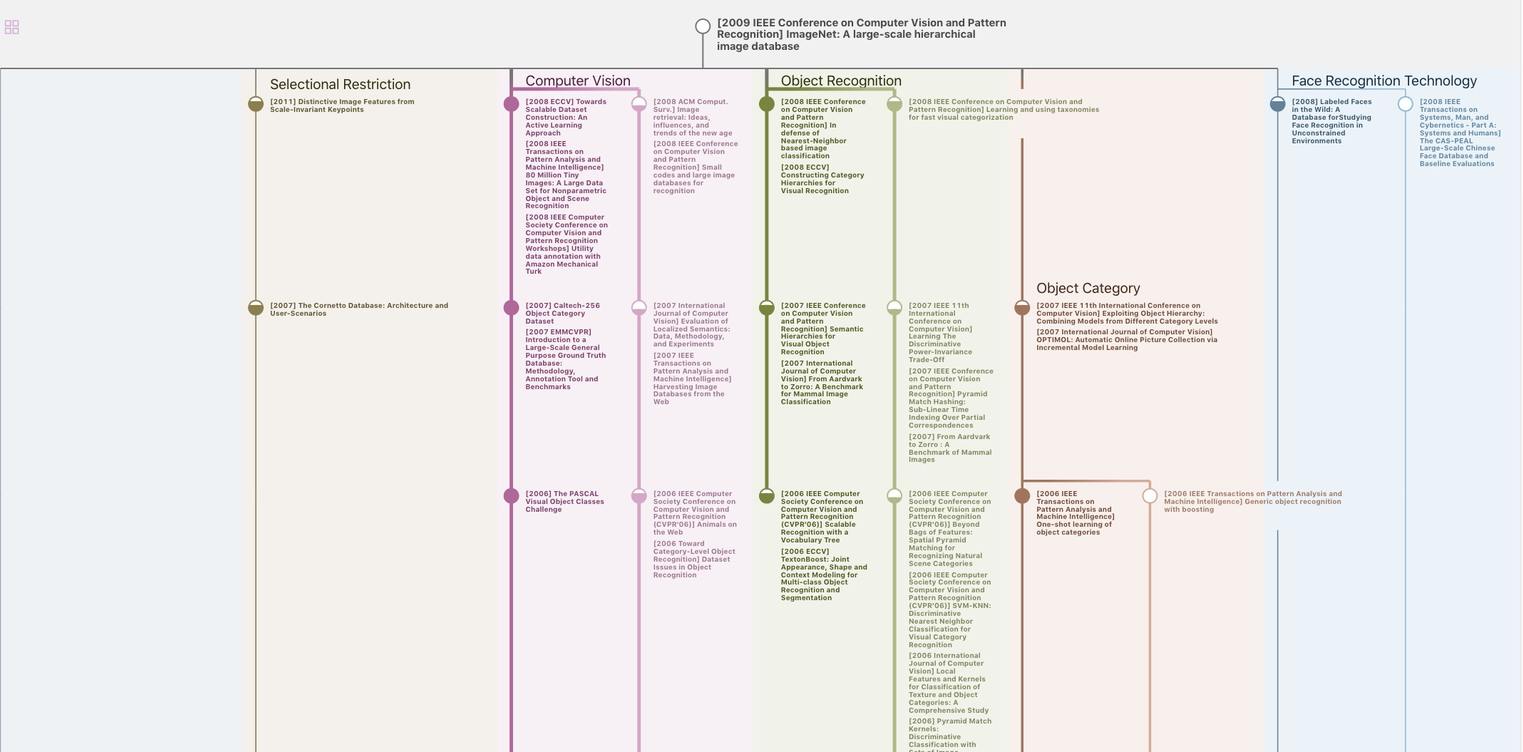
Generate MRT to find the research sequence of this paper
Chat Paper
Summary is being generated by the instructions you defined