Pattern unlocking guided multi-modal continuous authentication for smartphone with multi-branch context-aware representation learning and auto encoder
TRANSACTIONS ON EMERGING TELECOMMUNICATIONS TECHNOLOGIES(2024)
摘要
Widely accepted explicit authentication protocols are vulnerable to a series of attacks, for example, shoulder surfing and smudge attacks, leaving users with the constant burden of periodic password changes. As such, we propose a novel framework for continuous authentication on smartphones. This approach is guided by pattern unlocking, which is widely used and will not cause learning cost. After collecting multi-modal data that describe both behavioral and contextual information, we employ a multi-branch context-aware attention network as the representation learner to perform feature extraction, then an auto encoder is then used for authentication. To overcome challenges, including cold-start and few-shot training, which is less discussed in other works, we incorporate transfer learning with a coarse-to-fine pre-training workflow. Additionally, we deploy a hierarchical approach to offload model tuning overhead from smartphones. Extensive experiments on more than 68 000 real-world recordings validate the effectiveness of the proposed method, with an EER (equal error rate) of 2.472% under mixed contexts, which consistently outperforms state-of-the-art approaches under both static and mixed contexts. Integration of context-aware representation learner and self-supervised auto encoder revolves continuous authentication performance. Transfer learning driven coarse-to-fine training addresses cold-start/few-shot problem and accelerates actual application. Test bed with more than 68k real-word samples shows our work achieves 2.472% EER under mixed contexts, outperforming state-of-the-art.image
更多查看译文
AI 理解论文
溯源树
样例
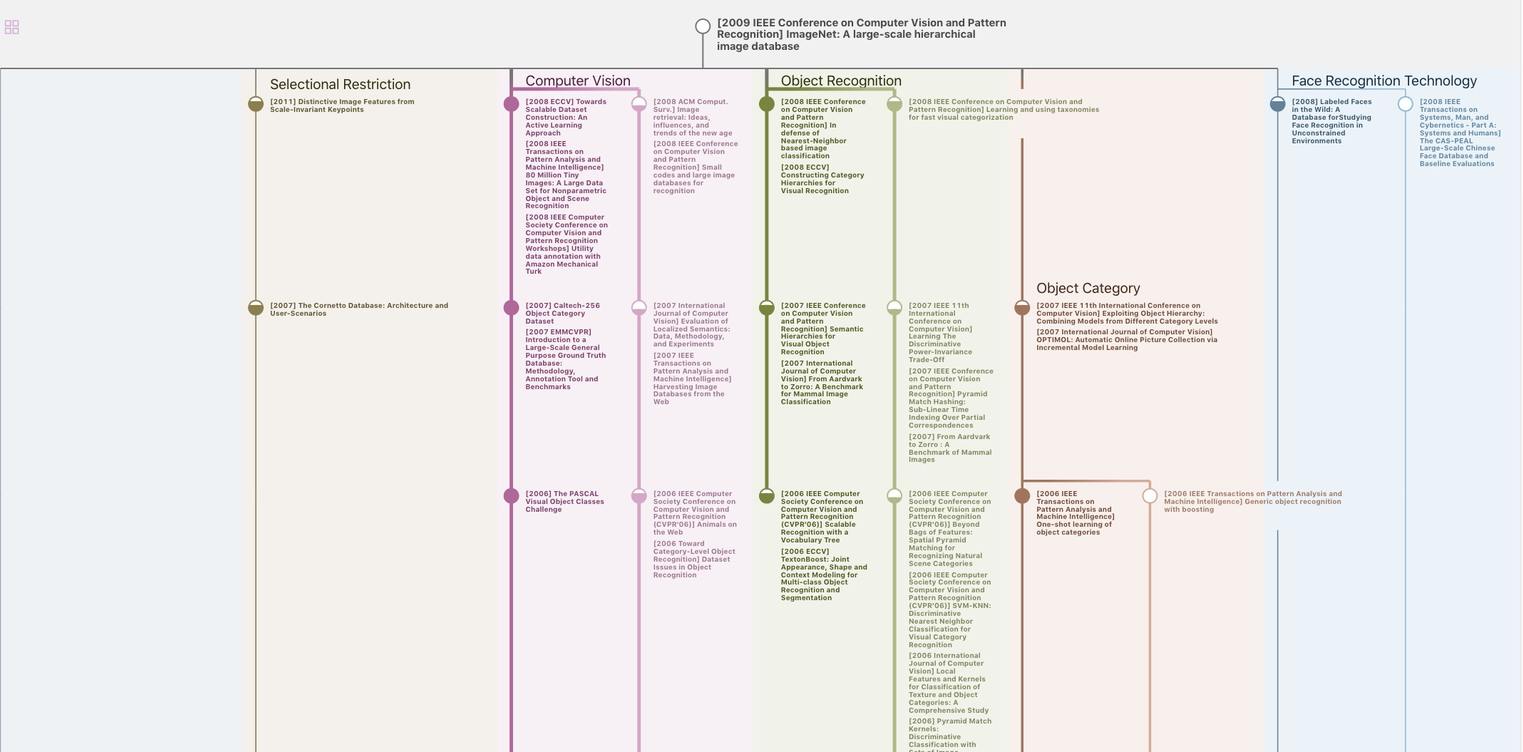
生成溯源树,研究论文发展脉络
Chat Paper
正在生成论文摘要