Multifidelity Constitutive Modeling of Stress-Induced Anisotropic Behavior of Clay
JOURNAL OF GEOTECHNICAL AND GEOENVIRONMENTAL ENGINEERING(2024)
摘要
Rigorous modeling of the stress-induced anisotropy of soils with different stress histories and loading conditions typically requires advanced constitutive models. However, calibration of state-of-the-art constitutive models can be expensive due to a large number of parameters and can encounter convergence issues when implemented in finite element codes. To circumvent these limitations, this study combines the well-known modified Cam-Clay (MCC) model with a machine learning-based multifidelity training framework, which is distinctive compared to current modeling approaches. A 'low-fidelity' neural network is first trained on synthetic data generated by the MCC model to 'learn' the model's interpretations of critical state soil mechanics. A 'high-fidelity' neural network is subsequently trained using limited experimental data to fine-tune predictions of soil behavior. The proposed framework is applied to the prediction of stress-induced anisotropy of lower Cromer till (LCT) clay. The results show that the mechanical behavior of LCT under drained and undrained triaxial compression/extension with different consolidation histories can be accurately predicted by the model. The model is also shown to be insensitive to the exact composition of the synthetic data set, specifically, the base constitutive model and parameter set used. It also shows an ability to generalize unseen data outside of the calibration space due to the underpinning soil mechanics training. Finally, explicit consideration of prediction uncertainty increases the interpretability and reliability of the proposed model toward increasing the likelihood of industry take-up.
更多查看译文
关键词
Constitutive model,Clays,Anisotropy,Neural network,Uncertainty
AI 理解论文
溯源树
样例
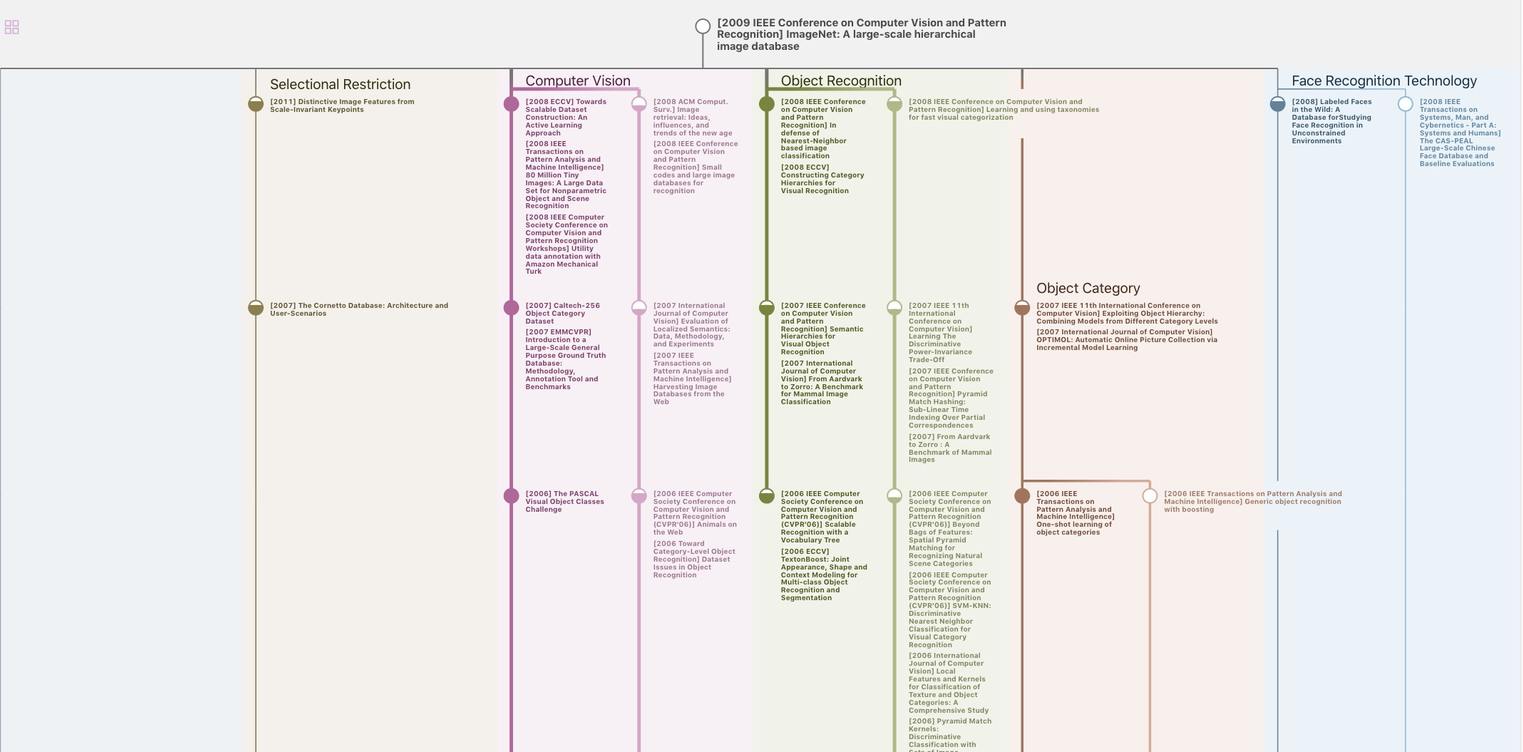
生成溯源树,研究论文发展脉络
Chat Paper
正在生成论文摘要