Nonparametric Bayesian functional clustering with applications to racial disparities in breast cancer
STATISTICAL ANALYSIS AND DATA MINING(2024)
摘要
As we have easier access to massive data sets, functional analyses have gained more interest. However, such data sets often contain large heterogeneities, noises, and dimensionalities. When generalizing the analyses from vectors to functions, classical methods might not work directly. This paper considers noisy information reduction in functional analyses from two perspectives: functional clustering to group similar observations and thus reduce the sample size and functional variable selection to reduce the dimensionality. The complicated data structures and relations can be easily modeled by a Bayesian hierarchical model due to its flexibility. Hence, this paper proposes a nonparametric Bayesian functional clustering and peak point selection method via weighted Dirichlet process mixture (WDPM) modeling that automatically clusters and provides accurate estimations, together with conditional Laplace prior, which is a conjugate variable selection prior. The proposed method is named WDPM-VS for short, and is able to simultaneously perform the following tasks: (1) Automatic cluster without specifying the number of clusters or cluster centers beforehand; (2) Cluster for heterogeneously behaved functions; (3) Select vibrational peak points; and (4) Reduce noisy information from the two perspectives: sample size and dimensionality. The method will greatly outperform its comparison methods in root mean squared errors. Based on this proposed method, we are able to identify biological factors that can explain the breast cancer racial disparities.
更多查看译文
关键词
functional clustering,nonparametric Bayesian model,peak point selection,surface-enhanced Raman spectroscopy,WDPM-VS,weighted Dirichlet process mixture
AI 理解论文
溯源树
样例
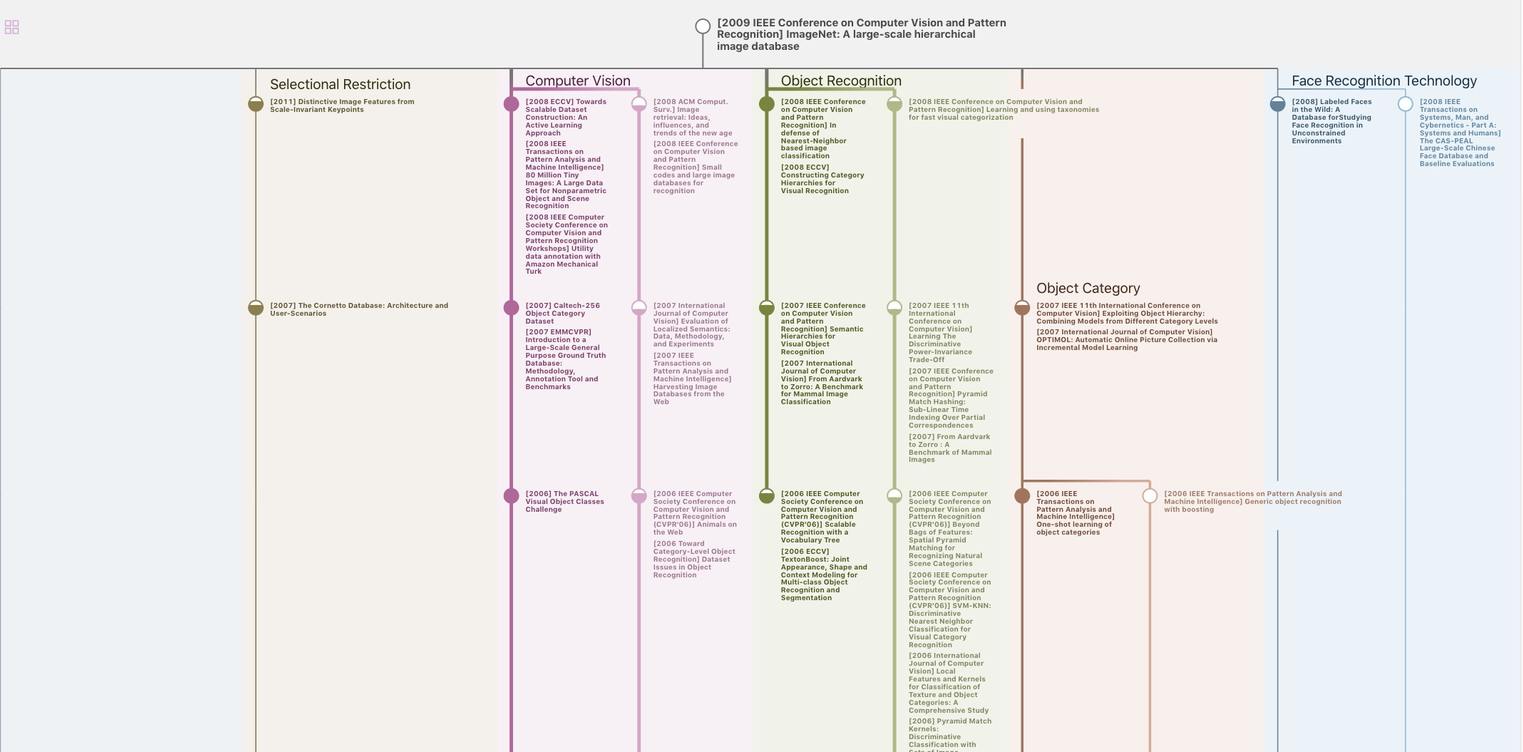
生成溯源树,研究论文发展脉络
Chat Paper
正在生成论文摘要