A multi-scale collaborative fusion residual neural network-based approach for bearing fault diagnosis
MEASUREMENT SCIENCE AND TECHNOLOGY(2024)
摘要
In recent years, deep learning techniques have become popular for diagnosing equipment faults. However, their real industrial application performance is hindered by challenges related to noise and variable load conditions that prevent accurate extraction of valid feature information. To tackle these challenges, this paper proposed a novel approach known as the multi-scale collaborative fusion residual neural network (MCFRNN) for bearing fault diagnosis. To begin with, the methodology introduces a multi-scale systolic denoising module designed to extract features at multiple scales while mitigating the influence of noise. Subsequently, a central fusion module is employed to explore the intrinsic correlation among the multiple channels and effectively fuse their respective features. Additionally, a global sensing module is incorporated to enhance the perceptual field of MCFRNN, thereby facilitating the extraction of global features. Furthermore, online label smoothing and AdamP are applied to alleviate overfitting and improve the diagnostic capability of MCFRNN under small sample. Finally, the effectiveness of MCFRNN is verified with two publicly available datasets under complex operational and limited sample conditions. The experimental results show that the proposed method has more excellent diagnostic performance and adaptivity than the existing popular methods.
更多查看译文
关键词
bearing fault diagnosis,multi-scale shrinkage denoising module,global sensing module,central fusion module,online label smoothing
AI 理解论文
溯源树
样例
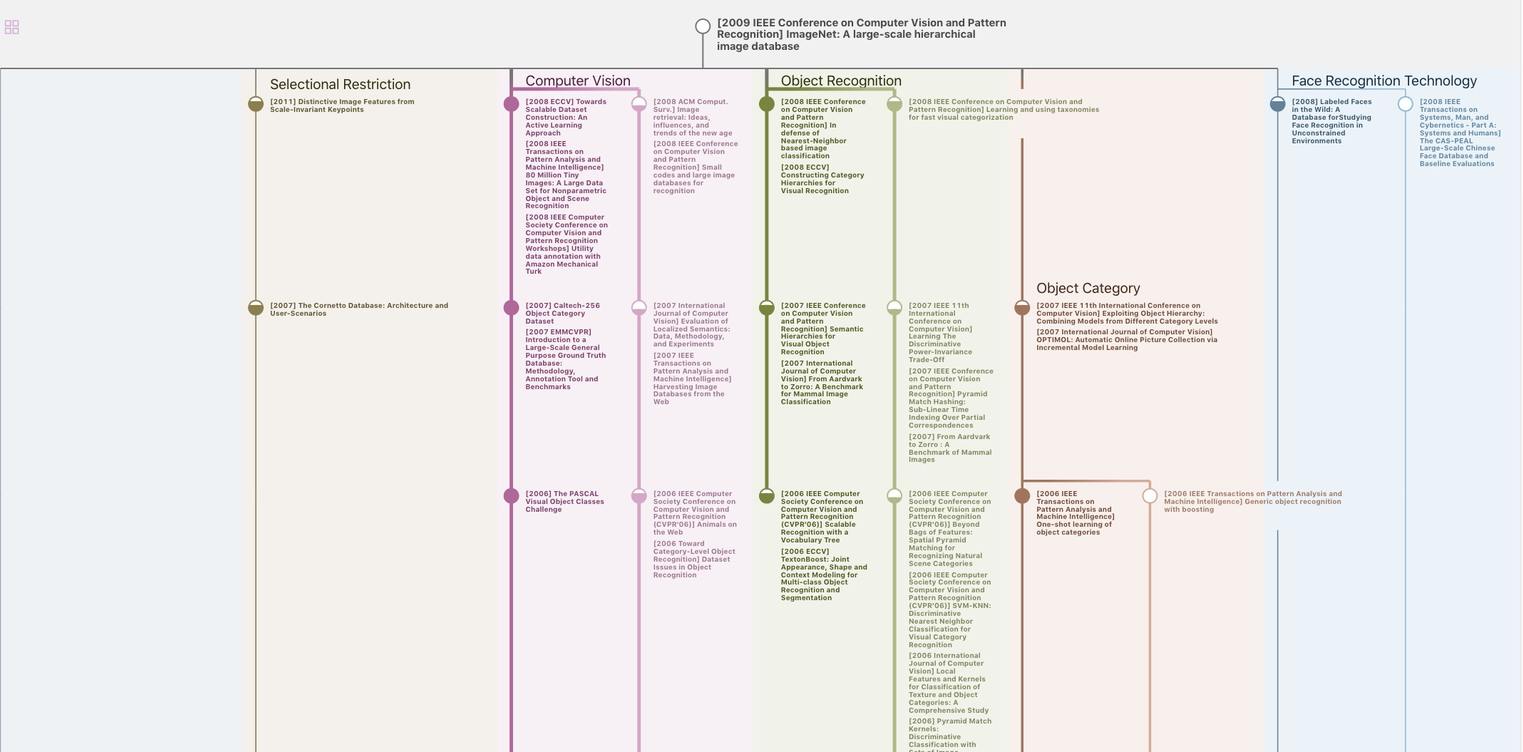
生成溯源树,研究论文发展脉络
Chat Paper
正在生成论文摘要