Tomato Health Monitoring System: Tomato Classification, Detection, and Counting System Based on YOLOv8 Model With Explainable MobileNet Models Using Grad-CAM plus
IEEE ACCESS(2024)
摘要
Fruits and vegetables (especially, tomatoes) healthy detection are important tasks for smart agriculture. Several works have been published in tomato detection, however, there is little research on using explainable AI to detect, classify and count tomato fruit status. In this work, we propose a Tomatoes Health Check System by evaluating MobileNet models based on the physiological tomato dataset. Our research conducts experiments to evaluate the accuracy of the MobileNets, MobileNetV2 and MobileNetV3 models based on the evaluation metrics; the highest accuracy of 96.69% belongs to the MobileNetV3 model. The proposed method we suggest is to utilize Grad-CAM++ for a visual explanation of predictions made by models belonging to the MobileNets family. Subsequently, we calculate Intersection over Union metrics at various thresholds (0%, 25%, and 50%) based on each heatmap or region of importance. To assess model reliability, Grad-CAM++ is used to explain and evaluate reliability, with MobileNetV2 achieving the highest values at 100.00% (delta=0), 100.00% (delta=0.25), and 98.89% (delta=0.5). An evaluation experiment combines the YOLOv8 and MobileNetV2 algorithms using the Simple Online and Real-time Tracking (SORT) algorithm to detect, classify, and count tomatoes based on physiological characteristics in videos. Finally, the research results are utilized to develop an application system.
更多查看译文
关键词
Explainable AI,interpretability,XAI,MobileNet models,smart farming,Grad-CAM plus plus,fruits object detection
AI 理解论文
溯源树
样例
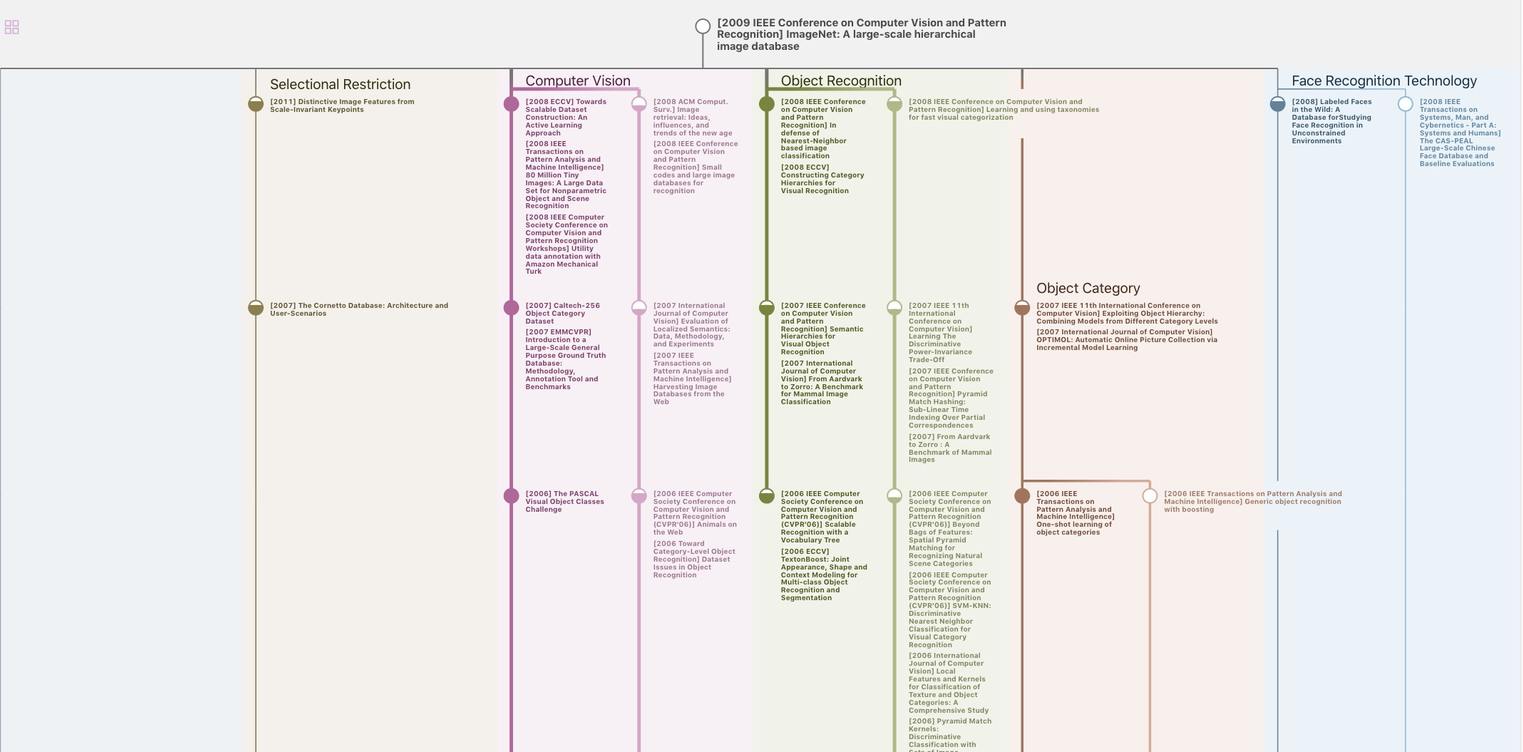
生成溯源树,研究论文发展脉络
Chat Paper
正在生成论文摘要