Data-driven numerical simulation with extended Kalman filtering and long short-term memory networks for highway traffic flow prediction
JOURNAL OF MECHANICS(2024)
摘要
Developing an accurate and reliable computational tool for traffic flow prediction has always been an active research topic in transportation engineering and planning. The available predictive tools generally fall into parametric, nonparametric and PDE-based approaches. In particular, the machine learning methods, such as the long short-term memory (LSTM) networks, belong to the nonparametric methods. This study proposes the data assimilation technique with LSTM for predicting highway traffic flows. The proposed method is developed under the framework of the extended Kalman filter (EKF) algorithm, which consists of two key components: the analysis and prediction steps. As the numerical simulator, a kernel component of the predictive tool, we use an explicit (EX) Godunov's scheme to discretize the Lighthill-Whitham-Richards model, where the MacNicholas formulation is used as the fundamental relation between the velocity and density. EKF combines LSTM prediction from two perspectives. In practical scenarios, future data at the upstream or downstream boundary points are unavailable. Therefore, the predicted values generated by LSTM are employed to set boundary conditions. Furthermore, two stages in EKF assimilate the LSTM predicted values, known as pseudo-observations, and the observed data in order with background values obtained through numerical simulation and observed data whenever available. This assimilation process aims to obtain a better initial condition for subsequent predictions, resulting in improved accuracy. Based on historical traffic data of the Hsuehshan Tunnel highway in Taiwan, the numerical results demonstrate that our method can effectively reduce the observation error and outperforms three baselines: EX, EKF and LSTM.
更多查看译文
关键词
Highway traffic flow prediction,data assimilation,Kalman filtering,deep learning
AI 理解论文
溯源树
样例
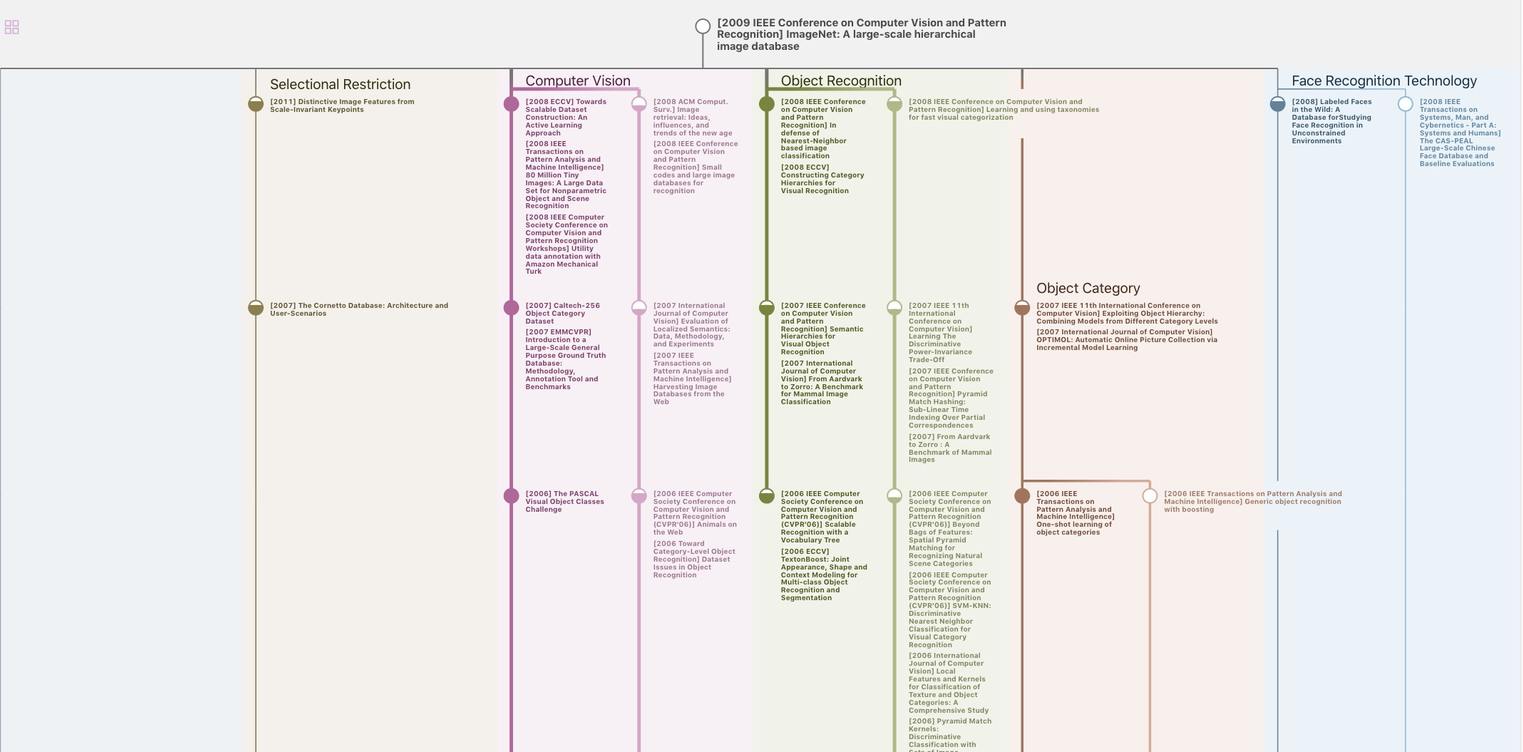
生成溯源树,研究论文发展脉络
Chat Paper
正在生成论文摘要