Predicting dynamic responses of continuous deformable bodies: A graph-based learning approach
COMPUTER METHODS IN APPLIED MECHANICS AND ENGINEERING(2024)
Abstract
Predicting dynamic responses of continuous deformable bodies (CDBs) is essential for various research fields, including civil engineering and computer graphics. Machine learning models, unlike traditional physically-based models, learn from data without predefined physical proper-ties and have significantly advanced the simulation of rigid body systems and fluids. Graph neural network (GNN)-based simulators excel in this area due to their ability to naturally represent physical bodies and interactions using graphs composed of nodes and edges. However, predicting the dynamic responses of CDBs remains challenging because interactions in a CDB are complex, heterogeneous, and dynamic. The complexity of interactions arises from the spatial variability of internal stress, making it challenging to encapsulate such multifaceted interactions of one edge into one edge attribute vector. Additionally, those interactions are heterogeneous and dynamic due to the material and geometric nonlinearity of CDBs. CDBs with elastic, plastic, and elastoplastic materials follow different deformation rules during vibrations. These rules also vary under small and large deformations for a single material type. To address these challenges, we introduce a new GNN-based simulator called physics-informed edge recurrent simulator (Piers) for learning CDB dynamics. We first formulate the CDB simulation as a sequence-to-sequence input-output relationship modeling problem and incorporate a recurrent neural network (RNN) to learn edge updates within short timesteps, during which stiffness changes are negligible. To accurately capture complex interactions, we initialize the RNN module's hidden states with prior physical knowledge and equip Piers with a physics-informed loss function by assigning the physical properties of interactions as the target edge output. Extensive experimental results demonstrate that Piers can simulate the dynamics of elastic, plastic, and elastoplastic CDBs with smaller response prediction errors than alternative baselines. Piers reduces prediction errors by 78% to 99% across four typical CDB physical systems.
MoreTranslated text
Key words
Physical system simulation,AI for science,Physics-informed machine learning,Graph neural network
AI Read Science
Must-Reading Tree
Example
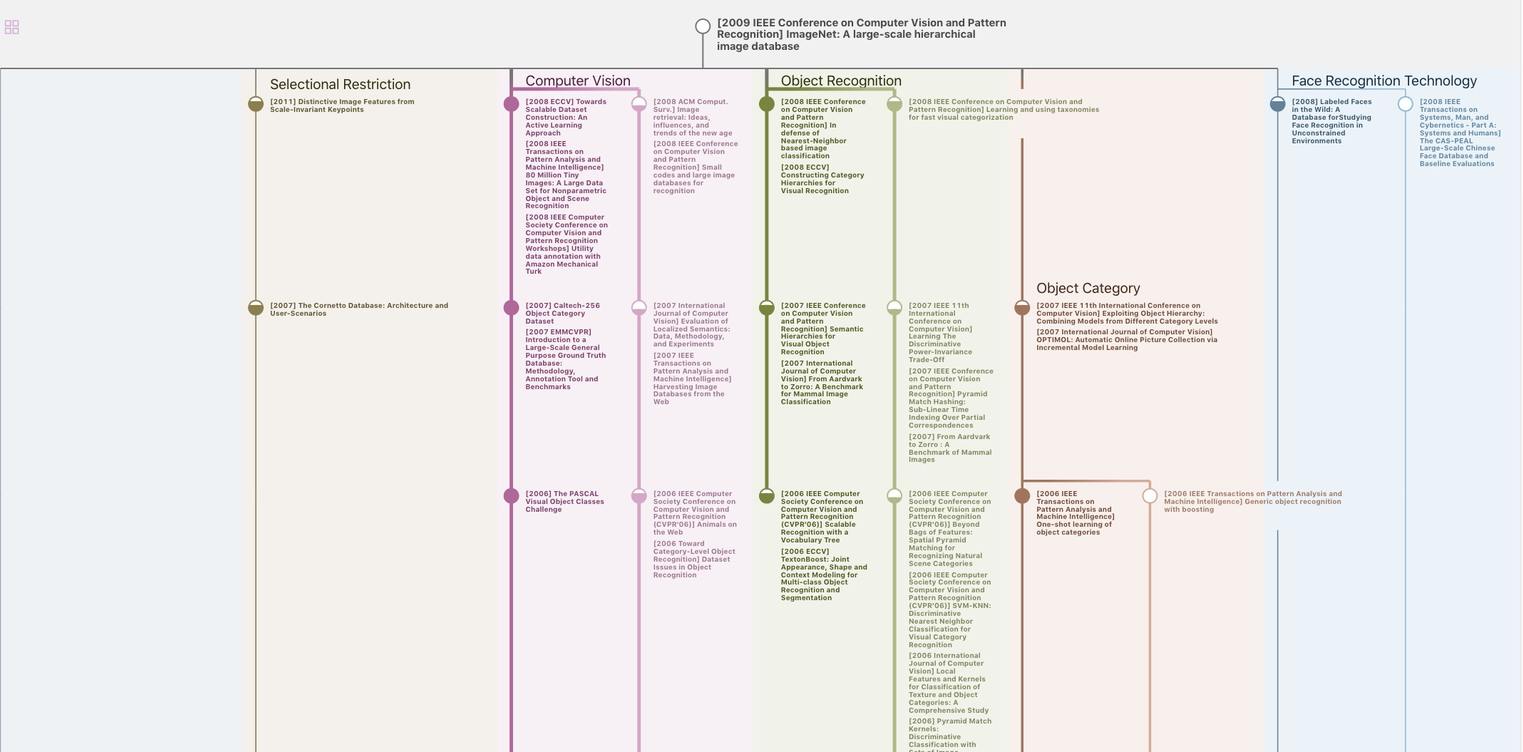
Generate MRT to find the research sequence of this paper
Chat Paper
Summary is being generated by the instructions you defined