Integrated climate-responsive thermal load ML model and cost/embodied energy estimate from a preliminary building design
ENERGY AND BUILDINGS(2024)
摘要
This paper proposes a framework applicable to preliminary building design that cohesively integrates material cost estimation, embodied energy assessment, and the prediction of the thermal demand of a building. At its core is a climate-responsive machine learning model specifically developed using readily accessible early-stage design data. This model forecasts the thermal demand both in the present and in the face of likely climatic shifts. To strike a balance in energy performance goals, material cost estimation is seamlessly incorporated. Building on the gradient boosting machine, the proposed approach showed better performance than other algorithms, predicting thermal demand 25 to 37.5 times faster than conventional energy software without compromising accuracy. The key findings of the presented case studies highlight the significant influence of the Window-to-Wall ratio and floor thermal mass on thermal demand-especially in mitigating cooling demands posed by potential climate change scenarios. By enhancing interior floor types, heating demand can be reduced by nearly 49%. The study further suggests that incorporating climate change considerations into life cycle energy designs is projected to amplify thermal and cooling loads by 32% and 94%, respectively, highlighting the crucial role of early design decisions in shaping sustainable and efficient buildings.
更多查看译文
关键词
Machine learning,Sustainability,Building energy,Environmental impact,Construction material
AI 理解论文
溯源树
样例
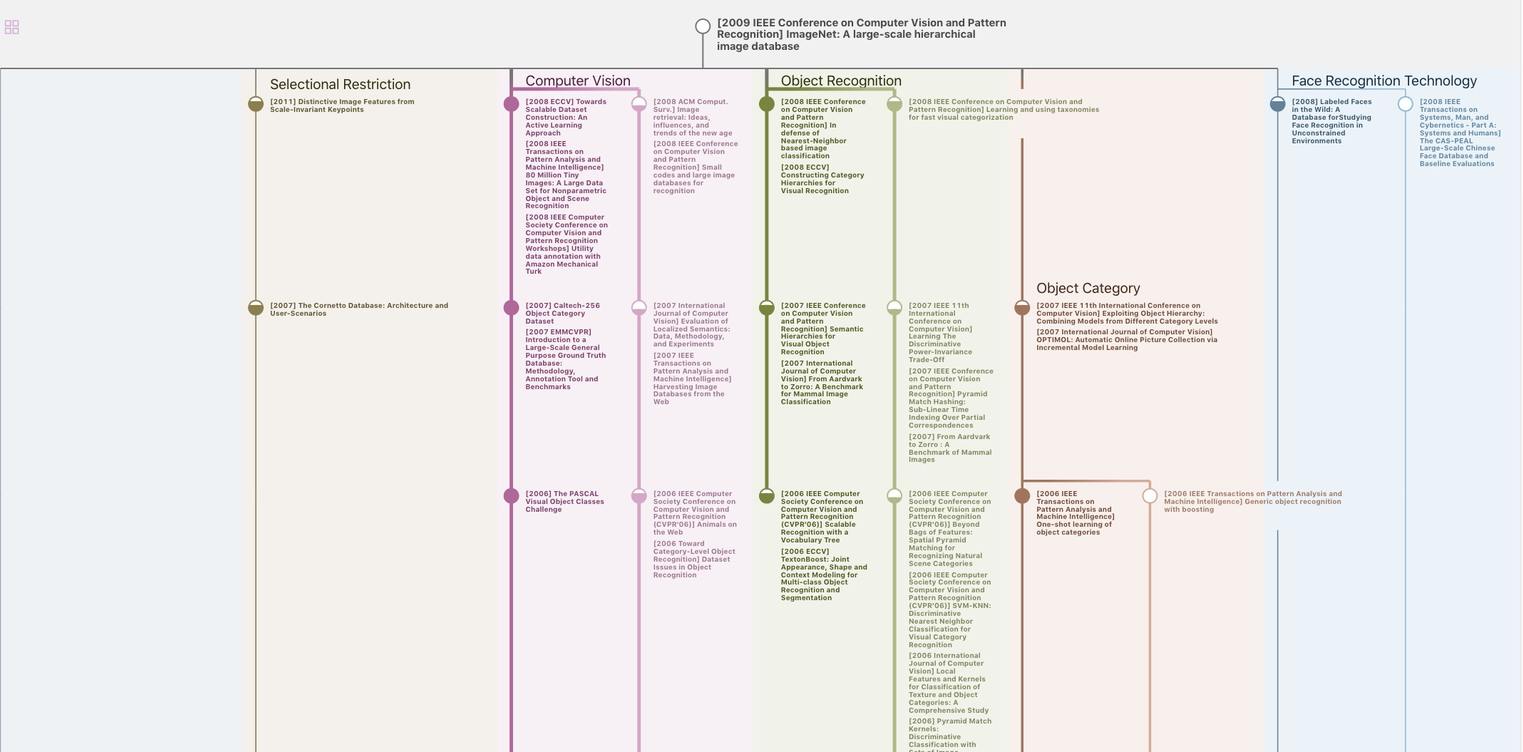
生成溯源树,研究论文发展脉络
Chat Paper
正在生成论文摘要