De novo molecule design towards biased properties via a deep generative framework and iterative transfer learning
DIGITAL DISCOVERY(2024)
摘要
De novo design of molecules with targeted properties represents a new frontier in molecule development. Despite enormous progress, two main challenges remain: (i) generating novel molecules conditioned on targeted, continuous property values; (ii) obtaining molecules with property values beyond the range in the training data. To tackle these challenges, we propose a reinforced regressional and conditional generative adversarial network (RRCGAN) to generate chemically valid molecules with targeted HOMO-LUMO energy gap (Delta EH-L) as a proof-of-concept study. As validated by density functional theory (DFT) calculation, 75% of the generated molecules have a relative error (RE) of <20% of the targeted Delta EH-L values. To bias the generation toward the Delta EH-L values beyond the range of the original training molecules, transfer learning was applied to iteratively retrain the RRCGAN model. After just two iterations, the mean Delta EH-L of the generated molecules increases to 8.7 eV from the mean value of 5.9 eV shown in the initial training dataset. Qualitative and quantitative analyses reveal that the model has successfully captured the underlying structure-property relationship, which agrees well with the established physical and chemical rules. These results present a trustworthy, purely data-driven methodology for the highly efficient generation of novel molecules with different targeted properties.
更多查看译文
AI 理解论文
溯源树
样例
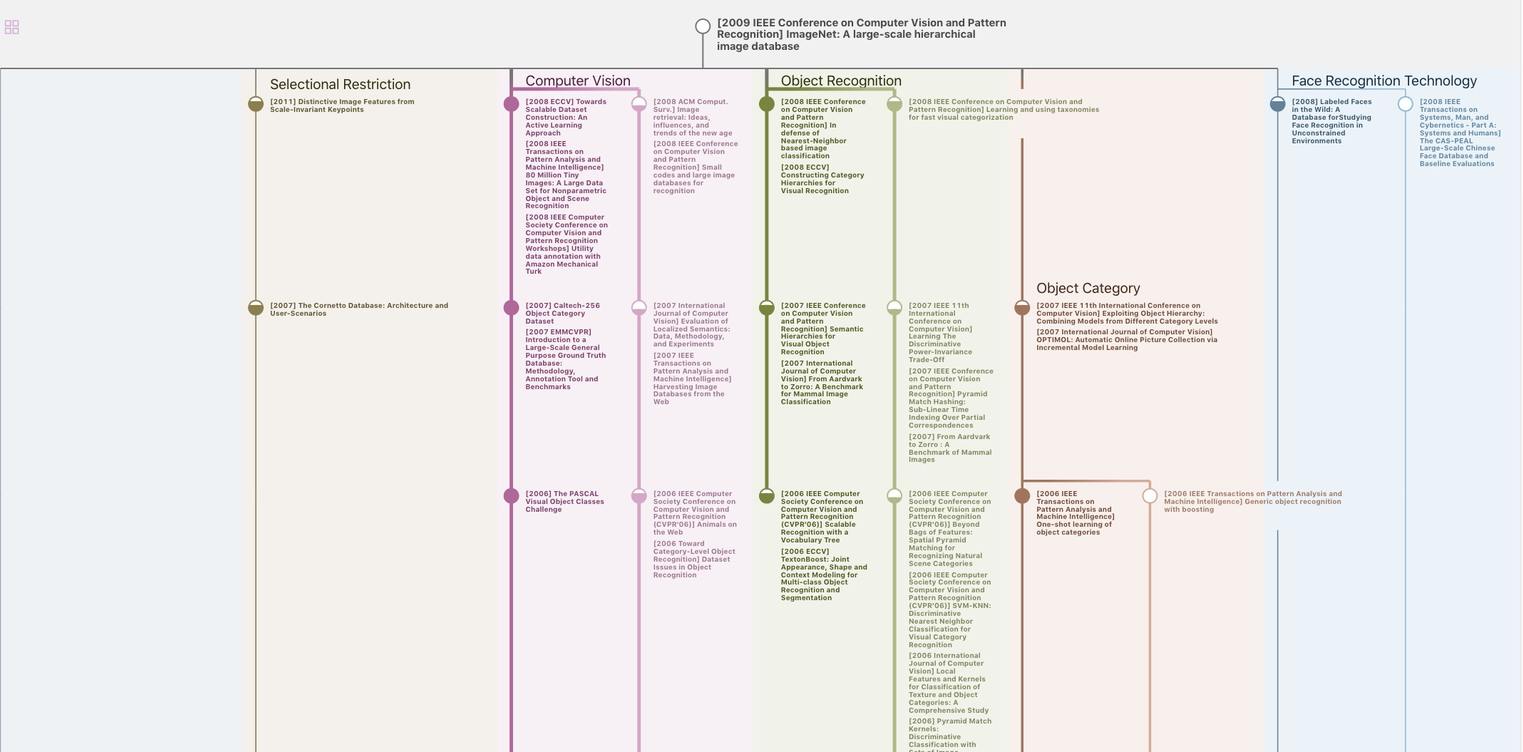
生成溯源树,研究论文发展脉络
Chat Paper
正在生成论文摘要